Diffusion kurtosis imaging and standard diffusion imaging in the magnetic resonance imaging assessment of prostate cancer
Introduction
Prostate cancer (PCa) is one of the most diagnosed cancers affecting men and represents one of the major causes of cancer-related death (1).
Current algorithms for PCa diagnosis and management provide different markers in the attempt to precociously diagnose PCa, since it could be asymptomatic in early phase or even associated with benign conditions (2-12).
Biopsy remains the gold standard for a confident diagnosis of PCa, although progression of technologies has led to increasing interest for advanced imaging (13,14) (Figure 1).
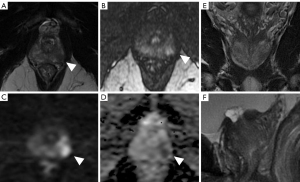
Notably, in latest years, magnetic resonance imaging (MRI) has assumed a primary role in the study of various districts and pathologies, becoming an integral component for diagnosis, risk stratification and staging of different cancers, and lately for targeting treatment (15-32).
Good diagnostic validity of MRI for PCa diagnosis derives from a high capability in combining morphological and functional data (33-43). From the study of Dola et al., multiparametric MRI (mpMRI) reached a sensitivity and specificity of 82.6% and 91.3%, respectively, with a positive and negative predictive value near to 100% (44).
MRI ability to adequately detect prostate lesions translate also in an improved diagnostic yield of biopsies, mostly through targeting the sample, and a good performance to detect local recurrency (45,46).
Current recommendations for prostate MRI acquisition protocol and interpretation, Prostate Imaging-Reporting and Data System (PI-RADS v2 and v2.1), edited by the American College of Radiology and ESUR, advise the acquisition of T2-weighted (T2W), diffusion-weighted imaging (DWI) and dynamic contrast-enhanced (DCE) sequences.
However, it remains essential to codify appropriate decision algorithm capable of modeling the pre-test risk of patients in order to help the standardization of the MRI approach.
Controversies in prostate MRI
The improvement of imaging accuracy for PCa diagnosis through new MRI techniques and sequences remains today a primary target for the radiology community, e.g., in the latest years, several studies focused on the use of quantitative analysis and computer-assisted diagnosis (CAD) methods, including artificial intelligence (AI) tools, to mitigate the subjective nature of MRI interpretation (47-55). Besides, high attention is recently posed on deep learning and radiomics application in various district and pathologies, including PCa (16,17,56-66). However, radiomics has shown a translational gap in clinical practice, and many issues remain to be solved.
Meanwhile, main concern regards DCE, considering that PI-RADS v2.1 poses greater emphasis on T2W and DWI as primary sequences for PCa diagnosis, confining DCE as a dichotomic variable (67,68). A wide literature recently highlighted the overlapping diagnostic validity of biparametric and multiparametric protocol in detecting clinically significant PCa (43,69-75).
Actually, mpMRI showed a relative superior sensitivity than biparametric protocol, returning the mpMRI a valuable complement in equivocal cases or smaller lesions, although with the risk for higher indolent cancer detection (69).
On the other hand, biparametric approach needs high standard of image quality and level of expertise than multiparametric ones. In particular, DWI optimization remains crucial for a correct interpretation of prostate MRI.
Current recommendations advise for high b-values acquisition to improve DWI accuracy, although higher b-values pair with a reduced signal-to-noise ratio. Moreover, ultrahigh b-values often reveal the presence of non-Gaussian diffusion effects, which requires a more sophisticated model for the analysis (76,77).
Purpose of this narrative review is to analyze the application of DWI and diffusion kurtosis imaging (DKI) for prostate analysis and discuss current evidence of DKI approach in prostate field. We present this article in accordance with the Narrative Review reporting checklist (available at https://gs.amegroups.com/article/view/10.21037/gs-23-53/rc).
Methods
Information used to write this paper was collected from PubMed (keywords: DKI; DWI; prostate cancer; and combination of this words) and included narrative overview; clinical research; systematic review and meta-analysis. The sources are also listed in Table 1.
Table 1
Items | Specification |
---|---|
Date of search | Start of research: June 2021; last editing: July 2023 |
Databases and other sources searched | PubMed |
Search terms used | DKI, DWI, prostate, prostate cancer |
Timeframe | 2000–May 2023 |
Inclusion and exclusion criteria | Inclusion criteria: research article, narrative review, systematic review, meta-analysis (only English article) |
Selection process | The research of literature was performed independently by four different authors (one senior researcher and three junior researchers) who then compared searches to avoid overlap. The resulting articles was then analyzed by the senior researcher who cataloged the evidence of each paper dividing them by category as follows: paper concerning the state of the art of MRI and prostate cancer; paper concerning the basic principles of DWI-ADC and DKI sequences; paper concerning evidence of DWI-ADC and DKI performance in PCa diagnosis. The final manuscript was compiled following the evidence and cataloging method |
DKI, diffusion kurtosis imaging; DWI, diffusion-weighted imaging; MRI, magnetic resonance imaging; ADC, apparent diffusion coefficient; PCa, prostate cancer.
Discussion
DWI
Owing to its unique sensitivity to the evaluation of molecular self-diffusion of water, DWI is a powerful tool for the non-invasive study of micro-structural properties of biological tissue in vivo.
Specifically, DWI analyzes the spontaneous mobility of water molecules (termed Brownian motion) reflecting their degree of motion (termed diffusion), thus potentially mirroring a cellular abnormality in a specific biologic tissue (78-82).
As diffusion is mostly restricted by cell membranes, the extent of restriction of free motion could be indeed proportionate to the cellular density of a tissue (83-85).
DWI currently results as one of the main sequences in detection and characterization of cancer lesion (86-93).
In prostate gland, as example, reduction in the movements of free water can derived from the replacement of large interstitial spaces and glandular lumens by nests of tumor cells and fibrous stroma as in PCa (46,94-99).
DWI is recognized as a primary determining sequence to assign the PI-RADS score for lesions within the peripheral zone (which account for 70–75% of the glandular tissue, and where the 85% of PCa cases are localized), identifying 5 scores based on the degree of restriction and the size of the restricted area (68,100).
DW-MRI most commonly relies on single-shot echo-planar-imaging spin-echo sequences with an application of two rectangular gradient pulses of an equal strength, applied before and after a 180° refocusing pulse (101). “Restricted” water molecules are dephased by the first pulse and completely rephrased by the second pulse (which gives back high signal) (102).
The strength and duration of the gradient pulses is expressed by the b-value.
High b-value are helpful for the visualization of clinically significant PCa by preserving the signal intensity only in the highly restricted area, especially in sub-capsular lesions (103-116).
Usually three b-values are obtained in clinical practice (with low values of about 50 s/mm2, and the higher one of at least 1,000 s/mm2), with current recommendation suggesting also high b-value for an adequate acquisition, although there is no widely accepted “high b-value” available in literature. Maximum b-value ranges from 2,000 to 3,000 seconds/mm2 (29), while higher b-values are not recommended.
From the study of Metens et al., highest tumor visibility was reached using b-values ranging from 1,500 to 2,000 seconds/mm2, with the best contrast-to-noise ratio (CNR) for b 1,500 seconds/mm2 using a 3-T magnetic resonance (MR) scanner (117). These results were confirmed by Katahira et al., who found the highest sensitivity (73.2%), specificity (89.7%) and accuracy (84.2%) for PCa detection using a b-value of 2,000 seconds/mm2 in addition to T2W imaging (T2WI) (106).
However, sensitivity in detecting clinically significant PCa tend to decrease with b-value higher than 3,200 seconds/mm2 (0.871 to 0.800), considering that signal-to-noise ratio (SNR) decreases as the b-value increases (118).
Historically, one of the main limits of MRI lies in fact, in the low ability to obtain good images quality at b-values greater than 1,000 seconds/mm2 due to insufficient SNR. Improvements in hardware and software however has recently enable the acquisition of ultrahigh b-value (119).
Moreover, acquiring DWI at ultrahigh b-values often reveals the presence of non-Gaussian diffusion effects, thus requiring a more sophisticated model for analysis (e.g., DKI) (120).
Other significant advantages derive from apparent diffusion coefficient (ADC) (121) maps. ADC refers to the measure of the magnitude of diffusion, resulting as the expression of the signal decay with increased b-value.
Water molecules restriction derived from areas with densely packed tumor cells, shows bright signal on DW-MRI and darker on the ADC map during visual qualitative assessment.
At least two b-values allows the calculation of ADC.
According to the ESUR guidelines, lower recommended values is 50–100 seconds/mm2 while high b-value is recommended in the range from 800–1,000 to 2,000 seconds/mm2.
However, besides the qualitative analysis, ADC allows also a quantitative assessment, proving to be a useful marker of tumor aggressiveness (122).
In fact, ADC values showed high correlation with cellularity in different study.
Different options are available for quantitative analysis, with ADC-ratio showing the higher accuracy (123), thus improving MRI accuracy in detection and localization of PCa.
Uncertainty regarding the reproducibility of the ADC hampers the use of quantitative DWI in PCa-MRI. From the study of Boss et al., test-retest repeatability and multi-day reproducibility were largely equivalent, with an inter-reader reliability for focal lesion ADC high across time points. However, controversial results derive from literature, and a quantitative ADC analysis results still limited (124-126).
Noteworthy, in latest years radiomics and machine learning (ML) have emerged as novel techniques for MRI analysis, through a quantitative assessment of intra- and intertumoral heterogeneities in the effort to extract latent information from standard acquisition (the so-called “radiomics hypothesis”).
In particular, texture analysis, as part of radiomics, allows grey-level intensity and pixels’ position, arrangement evaluation, and voxel intensities interrelation (127).
Several researchers have reported the usefulness of ML models using texture features extracted from DWI and T2WI for detecting and grading PCa. From the study of Fehr et al., PCa diagnosis can be improved by combining data-augmented classification together with ML model, compared with using ADC mean or T2 signal intensities alone [e.g., combined data reached an accuracy of 93% in differentiating Gleason Score (GS) of 6 and ≥7 for cancers occurring in both peripheral and transition zones vs. 58% using ADC mean only] (128).
Literature however is still lacking extensive studies including texture analysis for PCa, and validation studies in large cohorts are needed.
DKI
DKI analyses the non-Gaussian water diffusivity.
Specifically, DKI model describe the effect of signal intensity (SI) decay related to high b-value. Logarithmic SI decay plot for high b-value exhibits a non-linear shape, with a positive deviation from the plot of the mono-exponential model [mono-exponential model, valuable for low b-values up to 600–1,000 seconds/mm2, applies a linear fit to the natural logarithm of the signal intensity (SI)]. This deviation indicates the presence of water diffusion behaviors different from Gaussian predictions. Accordingly, both models should be applied (129).
DKI model uses two main metrics, defined as Dapp and Kapp.
Kapp refers to the apparent diffusional kurtosis (unitless) and reflects the more peaked distribution of tissue diffusivities occurring within the setting of non-Gaussian diffusion behavior.
Dapp is the diffusion coefficient (unit: ×10−3 mm2/s, µm2/millisecond, or ×103 µm2/s) corrected to account for the observed non-Gaussian behavior (130). Dapp is determined by the slope of the SI decay plot as b approaches to 0 (131).
Differently from other mathematical model including the bi-exponential one, DKI model potentially better describes water diffusivity in tissues at ultrahigh b-values, providing also an additional parameter (i.e., Kapp) that contains specific information on the non-Gaussian diffusion behavior. However, up to date, all models for high b-value diffusion-weighted images in PCa, including the biexponential, kurtosis, stretched exponential, and gamma distribution models achieve similar areas under the curve (AUCs) for discrimination of normal and cancer tissue, although biexponential and gamma distribution models produce statistically preferred fits (132).
Kapp is a phenomenological parameter with no biophysical correlate, similar to ADC.
ADC, as mentioned, reflects only water restriction outside of cells, which is influenced by tissue architectural properties. Therefore, besides the increasing cellular density, greater concentration of macromolecules and increased viscosity also can affect ADC.
Kapp is supposed to represent the direct interaction of water molecules with cell membranes and intracellular compounds, although other factors could influence these interactions. From the studies of Le Bihan (129), complex interaction of water molecules, interfaces and protein, with a polar nature of micromolecular components, may result in a significant restriction to water motion and contribution to non-Gaussian diffusion observations. Increased kurtosis could occur in the setting of more irregular and heterogeneous environments with many or large interfaces, including the increased nuclear-cytoplasmic ratio of tumor cells (133-135).
However, some technical aspect should be considered for DKI analysis.
First, DKI analysis needs separate post-processing software since current MR systems do not offer in-line DKI post-processing options.
DKI assessment should offer two maps (Dapp and Kapp).
Dapp map is similar to ADC map. Therefore, ADC and mean diffusivity values in PCa resulted lower than the regular parenchyma, while mean kurtosis value resulted higher (136-138) (Figures 2,3). Nevertheless, quantitative analysis of both Dapp and Kapp values is recommended since a reduced of Dapp not necessarily pairs with elevated kapp, such as for viscous or turbid fluid.
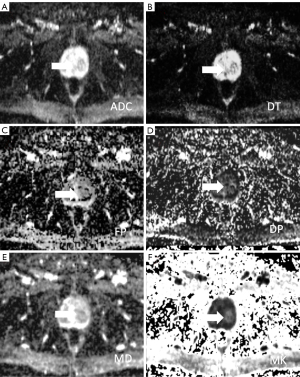
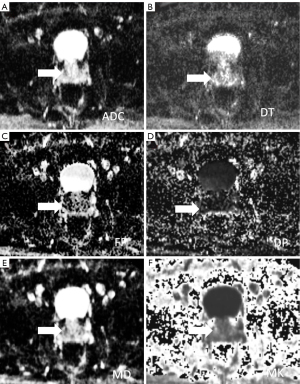
Second, to increase DKI metrics accuracy, sufficient SNR is critical. In fact, low SI leads to biased estimation of Kapp (139,140). Therefore, excessively high b-values (i.e., over 3,000 s/mm2) are therefore discouraged (141). Also, the use of a 3-T system, when available, could be a successful strategy to improves SNR (142).
In this regard, obtaining adequate SNR using high b-value is often difficult in body imaging. In fact, the use of sequences with faster acquisitions to avoid typical artifacts (e.g., breathing artifact) is associated with a faster decay of the signal. Moreover, DKI requires a minimum of three b-values, although with the risk of increasing the overall scan time and the likelihood of motion artifacts (142).
On the other hand, b-values including both high (500–1,000 s/mm2) and ultrahigh (1,500–2,000 s/mm2) ranges, may be useful for successfully capturing the mono-exponential and non-Gaussian components of the SI decay curve, respectively.
Therefore, the optimal number of b-values to obtain cannot be strictly prescribed and will depend on the clinical application, given the pronounced risk of longer acquisition and subsequent artifact (143,144).
DKI and PCa
DKI was first described in 2004 (145) and 2005 (131), and initially applied exclusively for brain imaging. Among multiple extra-cranial sites, DKI was recently explored also in PCa. However, the relatively young age of this analysis pairs with contrasting results especially about its added value compared to standard DWI protocol.
First interesting results came from the study of Rosenkrantz et al. (146), including a comparative analysis between diffusion imaging metrics and 121 cancerous sextants from 47 prostate patients (70 with a GS of 6, and the remaining 51 cancerous sextants with a GS greater than 6).
From a mixed-model analysis of variance and ROC analysis, DKI metrics resulted significantly altered both in tumor compared to normal parenchyma as well as in tumor, with respect of the GS grading. Notably, Kapp showed a higher sensitivity than ADC and Dapp for tumor vs. regular parenchyma differentiation (93.3% vs. 78.5% of ADC, P<0.001; and vs. 83.5% of Dapp, P<0.001), as a higher AUC for GS differentiation (146).
The obvious clinical impact of a correct differentiation of the degree of cancer aggressiveness is also shown by the recent evidences regarding the post-operative upgrading of the GS, widely recognized as an unfavorable prognostic factor both for a worse patient prognosis and for the risk of retreatment (147-149).
Therefore, imaging metrics capable in correcting staging a higher tumor aggressiveness and potential post-operative GS upgrading should be considered of primary importance in regular analysis.
In this regard, some interesting evidences are suggested by the study of Hectors et al., in which DKI shows a good correlation with the histopathological parameters of PCa (150); and the study of Wu et al. showed that a comprehensive consideration of DKI and prostate-specific antigen (PSA) may be a promising approach to predicting GS upgrade, with an AUC of the model Kapp-PSA reaching 0.868 vs. 0.819 shown by the single Kapp parameters (151).
A better estimation of tumor aggressiveness should be mandatory also in active surveillance patients, with preliminary results of a different study of Rosenkrantz et al., suggesting that diffusional kurtosis imaging findings may have more value than standard DWI as a marker of adverse final pathologic outcome among active surveillance candidates (152).
Of note, two other study corroborate an advantageous DKI impact.
From the study of Park et al., comparing the diagnostic performance of DKI metrics and ADC for determination of clinically significant prostate cancer (csPCa) (i.e., GS >7) in 92 patients, a major sensitivity of DKI was highlighted compared to mono-exponential ADC (153).
And similarly, robust results derived also from a recent meta-analysis of Shen et al., including 14 studies involving 1,847 lesions in 1,107 patients.
Pooled analysis showed an overall AUC of 0.89 for Kapp and 0.92 for Dappvs. 0.89 of DWI, with the superiority of Dapp to Kapp and ADC in separating malignant cancers from benign lesions, also confirmed by their subgroup analysis of PCa (154).
These finding could suggest an added value of DKI to the routine imaging protocol for screening cancer.
However, the body of literature resulted still controversial on DKI superiority considering that different works showed also a failed superiority of DKI metrics than mono-exponential ADC.
Firstly, from the study of Quentin et al., including 14 PCa patients and 10 healthy volunteers, although the mean kurtosis value was significantly higher in PCa than in the normal peripheral and central zones, DKI metrics weakly correlated with GS (155).
Secondly, from the study of Roethke et al., prostate DKI yields no significant added value for cancer detection compared with a standard DWI-derived mono-exponential ADC measurement (156).
Similar result was shown also from the study of Tamada et al. which included a larger population sample (255 patients), with the purpose to compare the value of DW-MRI and DKI for detection and characterization of PCa (157,158).
ADC and Kapp, in fact, were highly correlated, had similar diagnostic performance, and were concordant for the various outcomes in the large majority of cases, with non-different AUC for csPCa differentiation (P=0.15).
Finally, from a recent meta-analysis of Si et al., DKI does not provide significant added value for tumor detection in the peripheral zone. Because of the significant overlap in quantitative values between different tissue types, neither DKI nor ADC alone seems promising for a patient-based assessment of tumor aggressiveness. Therefore, for routine clinical application, ADC derived from single-exponential DWI remains the standard (156,159,160). A summary of the mentioned studies published focusing on DKI in prostate analysis is shown in Table 2.
Table 2
Study | Journal | Country | No. of patients | Type of paper | Validation of results | Results |
---|---|---|---|---|---|---|
Rosenkrantz et al. (146) | Radiology | USA | 47 | Original | Systematic sextant needle biopsy | K higher in cancerous sextants than in benign PZ |
K higher in cancerous sextants with higher rather than lower GS | ||||||
K showed greater SE than ADC or D (93.3% vs. 78.5% and 83.5%, respectively), with equal SP | ||||||
K had significantly greater AUC for differentiating sextants with low- and high-grade cancer than ADC | ||||||
Hectors et al. (150) | Radiology | USA | 24 | Original | Prostatectomy | DWI parameters (including DKI) were significantly different between prostate cancer and PZ |
Kurtosis showed significant correlations with histopathologic parameters (P<0.04) | ||||||
Wu et al. (151) | AJR Am J Roentgenol | China | 52 | Original | Prostatectomy | K max had the highest ROC AUC value (0.819, P<0.05) |
PSA-K max had the highest AUC (0.868, P<0.05) and Youden index (0.652) | ||||||
Rosenkrantz et al. (152) | AJR Am J Roentgenol | USA | 58 | Original | Biopsy cores | Only D was significantly lower in patients with adverse final pathologic findings |
Park et al. (153) | Abdom Radiol (NY) | Korea | 92 | Original | Pathologic topographic maps or systemic biopsy results | Similar ROC-AUC of K, ADC and D for discriminating CSC from non-CSC |
Shen et al. (154) | Clin Imaging | China | NA | Meta-analysis | NA | Pooled analysis showed a superiority of D analysis to K and ADC: |
K = SE: 0.83; SP: 0.83; +LR: 4.61; −LR: 0.22; AUC: 0.89 | ||||||
D = SE: 0.85; SP: 0.85; +LR: 6.39; −LR: 0.19; AUC: 0.92 | ||||||
ADC = SE: 0.82; SP: 0.85; +LR: 4.75; −LR: 0.24; AUC: 0.89 | ||||||
Quentin et al. (155) | Magn Reson Imaging | Germany | 24 | Original | Biopsy proven PCa | Monoexponential ADC is sufficient to discriminate prostate cancer from normal tissue (b-values ranging from 0 to 800 s/mm) |
Roethke et al. (156) | Invest Radiol | Germany | 55 | Original | Image-guided targeted biopsy | D was significantly lower in tumor compared with control regions |
K was significantly higher in tumor | ||||||
D was significantly higher than standard ADC both in tumor regions and in controls | ||||||
ROC analyses showed similar capability between DKI and ADC for detection of PCa | ||||||
ROC analyses showed significant capability between DKI and ADC for discrimination between high- and low-grade findings | ||||||
Tamada et al. (157) | Radiology | USA | 285 | Original | Prostatectomy | ADC and K showed significant differences for benign vs. tumor tissues |
ROC AUC-ADC (0.921) > ROC AUC-K (0.902) for benign vs. malignant tissue but was similar for high GS discrimination | ||||||
Si et al. (159) | AJR Am J Roentgenol | China | NA | Meta-analysis | NA | ADC = pooled SE: 0.89; pooled SP: 0.86; ROC AUC: 0.93 |
D = pooled SE: 0.91; pooled SP: 0.78; ROC AUC: 0.89 | ||||||
K = pooled SE: 0.87; pooled SP: 0.85; ROC AUC: 0.93 |
K, kurtosis; PZ, peripheral zone; GS, Gleason Score; SE, sensitivity; ADC, apparent diffusion coefficient; D, diffusion; SP, specificity; AUC, area under the curve; DWI, diffusion-weighted imaging; DKI, diffusion kurtosis imaging; max, maximum; ROC, receiver operating characteristic; PSA, prostate-specific antigen; CSC, cancer stem cells; NA, not available; LR, likelihood ratio.
Given these premises, DKI did not show a clear added value compared with standard DWI for clinical PCa, therefore remaining debatable whether it should be incorporated into routine clinical imaging, also considering the longer scan time given the need to acquire at least three b-values.
However, the need to optimize MRI protocols for cancer screening is continuously growing, with high attention on non-conventional analysis, as radiomics. And therefore, this evidence may represent the starting point for the development of protocols including the use of DKI, thanks to its promising diagnostic application and interesting preliminary results in PCa detection and staging (161).
Conclusions
DWI and its associated ADC map remain, at present, the most reliable imaging approach to the PCa. Recently, different studies have examined the value of DKI compared with standard DWI in detecting PCa and assessing its aggressiveness. However, the results still remain controversial, probably limited also from the study samples investigated. Wider application and greater technical knowledge of DKI, however, may help prove its intrinsic validity in the field of oncology and therefore in the study of csPCa.
Acknowledgments
The authors wish to thank Angela Martella (Department of Applied Clinical Sciences and Biotechnology, University of L’Aquila, L’Aquila, Italy) for the English revision.
Funding: None.
Footnote
Reporting Checklist: The authors have completed the Narrative Review reporting checklist. Available at https://gs.amegroups.com/article/view/10.21037/gs-23-53/rc
Peer Review File: Available at https://gs.amegroups.com/article/view/10.21037/gs-23-53/prf
Conflicts of Interest: All authors have completed the ICMJE uniform disclosure form (available at https://gs.amegroups.com/article/view/10.21037/gs-23-53/coif). R.F. is an employee at Medical Oncology Division, Igea SpA. The other authors have no conflicts of interest to declare.
Ethical Statement: The authors are accountable for all aspects of the work in ensuring that questions related to the accuracy or integrity of any part of the work are appropriately investigated and resolved.
Open Access Statement: This is an Open Access article distributed in accordance with the Creative Commons Attribution-NonCommercial-NoDerivs 4.0 International License (CC BY-NC-ND 4.0), which permits the non-commercial replication and distribution of the article with the strict proviso that no changes or edits are made and the original work is properly cited (including links to both the formal publication through the relevant DOI and the license). See: https://creativecommons.org/licenses/by-nc-nd/4.0/.
References
- Sung H, Ferlay J, Siegel RL, et al. Global Cancer Statistics 2020: GLOBOCAN Estimates of Incidence and Mortality Worldwide for 36 Cancers in 185 Countries. CA Cancer J Clin 2021;71:209-49. [Crossref] [PubMed]
- Culp MB, Soerjomataram I, Efstathiou JA, et al. Recent Global Patterns in Prostate Cancer Incidence and Mortality Rates. Eur Urol 2020;77:38-52. [Crossref] [PubMed]
- Mantica G, Chierigo F, Suardi N, et al. Minimally invasive strategies for the treatment of prostate cancer recurrence after radiation therapy: a systematic review. Minerva Urol Nefrol 2020;72:563-78. [Crossref] [PubMed]
- Slawin KM, Diblasio CJ, Kattan MW. Minimally Invasive Therapy for Prostate Cancer: Use of Nomograms to Counsel Patients about the Choice and Probable Outcome of Therapy. Rev Urol 2004;6:S3-8.
- Barra S, Guarnieri A, di Monale E, Bastia MB, et al. Short fractionation radiotherapy for early prostate cancer in the time of COVID-19: long-term excellent outcomes from a multicenter Italian trial suggest a larger adoption in clinical practice. Radiol Med 2021;126:142-6. [Crossref] [PubMed]
- Fersino S, Borghesi S, Jereczek-Fossa BA, et al. PROACTA: a survey on the actual attitude of the Italian radiation oncologists in the management and prescription of hormonal therapy in prostate cancer patients. Radiol Med 2021;126:460-5. [Crossref] [PubMed]
- Mazzola R, Cuccia F, Figlia V, et al. Stereotactic body radiotherapy for oligometastatic castration sensitive prostate cancer using 1.5 T MRI-Linac: preliminary data on feasibility and acute patient-reported outcomes. Radiol Med 2021;126:989-97. [Crossref] [PubMed]
- Francolini G, Detti B, Di Cataldo V, et al. Study protocol and preliminary results from a mono-centric cohort within a trial testing stereotactic body radiotherapy and abiraterone (ARTO-NCT03449719). Radiol Med 2022;127:912-8. [Crossref] [PubMed]
- Francolini G, Jereczek-Fossa BA, Di Cataldo V, et al. Stereotactic or conventional radiotherapy for macroscopic prostate bed recurrence: a propensity score analysis. Radiol Med 2022;127:449-57. [Crossref] [PubMed]
- Francolini G, Stocchi G, Detti B, et al. Dose-escalated pelvic radiotherapy for prostate cancer in definitive or postoperative setting. Radiol Med 2022;127:206-13. [Crossref] [PubMed]
- Nicosia L, Mazzola R, Vitale C, et al. Postoperative moderately hypofractionated radiotherapy in prostate cancer: a mono-institutional propensity-score-matching analysis between adjuvant and early-salvage radiotherapy. Radiol Med 2022;127:560-70. [Crossref] [PubMed]
- Valeriani M, Detti B, Fodor A, et al. Radiotherapy at oligoprogression for metastatic castration-resistant prostate cancer patients: a multi-institutional analysis. Radiol Med 2022;127:108-16. [Crossref] [PubMed]
- Leslie SW, Soon-Sutton TL, R I A, et al. Prostate Cancer. 2023 Nov 13. In: StatPearls [Internet]. Treasure Island (FL): StatPearls Publishing; 2023.
- Manetta R, Palumbo P, Gianneramo C, et al. Correlation between ADC values and Gleason score in evaluation of prostate cancer: multicentre experience and review of the literature. Gland Surg 2019;8:S216-22. [Crossref] [PubMed]
- Litwin MS, Tan HJ. The Diagnosis and Treatment of Prostate Cancer: A Review. JAMA 2017;317:2532-42. [Crossref] [PubMed]
- Autorino R, Gui B, Panza G, et al. Radiomics-based prediction of two-year clinical outcome in locally advanced cervical cancer patients undergoing neoadjuvant chemoradiotherapy. Radiol Med 2022;127:498-506. [Crossref] [PubMed]
- Granata V, Fusco R, De Muzio F, et al. Radiomics textural features by MR imaging to assess clinical outcomes following liver resection in colorectal liver metastases. Radiol Med 2022;127:461-70. [Crossref] [PubMed]
- Grasso RF, Bernetti C, Pacella G, et al. A comparative analysis of thermal ablation techniques in the treatment of primary and secondary lung tumors: a single-center experience. Radiol Med 2022;127:714-24. [Crossref] [PubMed]
- Orlacchio A, Guastoni C, Beretta GD, et al. SIRM-SIN-AIOM: appropriateness criteria for evaluation and prevention of renal damage in the patient undergoing contrast medium examinations-consensus statements from Italian College of Radiology (SIRM), Italian College of Nephrology (SIN) and Italian Association of Medical Oncology (AIOM). Radiol Med 2022;127:534-42. [Crossref] [PubMed]
- Salvestrini V, Becherini C, Desideri I, et al. The impact of patient preference in the treatment algorithm for recurrent/metastatic head and neck squamous cell carcinoma. Radiol Med 2022;127:866-71. [Crossref] [PubMed]
- Tagliafico AS, Bignotti B, Torri L, et al. Sarcopenia: how to measure, when and why. Radiol Med 2022;127:228-37. [Crossref] [PubMed]
- Wang FH, Zheng HL, Li JT, et al. Prediction of recurrence-free survival and adjuvant therapy benefit in patients with gastrointestinal stromal tumors based on radiomics features. Radiol Med 2022;127:1085-97. [Crossref] [PubMed]
- Xue K, Liu L, Liu Y, et al. Radiomics model based on multi-sequence MR images for predicting preoperative immunoscore in rectal cancer. Radiol Med 2022;127:702-13. [Crossref] [PubMed]
- Chiloiro G, Cusumano D, de Franco P, et al. Does restaging MRI radiomics analysis improve pathological complete response prediction in rectal cancer patients? A prognostic model development. Radiol Med 2022;127:11-20. [Crossref] [PubMed]
- De Robertis R, Geraci L, Tomaiuolo L, et al. Liver metastases in pancreatic ductal adenocarcinoma: a predictive model based on CT texture analysis. Radiol Med 2022;127:1079-84. [Crossref] [PubMed]
- Deandrea S, Sardanelli F, Calabrese M, et al. Provision of follow-up care for women with a history of breast cancer following the 2016 position paper by the Italian Group for Mammographic Screening and the Italian College of Breast Radiologists by SIRM: a survey of Senonetwork Italian breast centres. Radiol Med 2022;127:484-9. [Crossref] [PubMed]
- Fan Y, Zhao Z, Wang X, et al. Radiomics for prediction of response to EGFR-TKI based on metastasis/brain parenchyma (M/BP)-interface. Radiol Med 2022;127:1342-54. [Crossref] [PubMed]
- Fusco R, Granata V, Sansone M, et al. Validation of the standardized index of shape tool to analyze DCE-MRI data in the assessment of neo-adjuvant therapy in locally advanced rectal cancer. Radiol Med 2021;126:1044-54. [Crossref] [PubMed]
- Girometti R, Linda A, Conte P, et al. Multireader comparison of contrast-enhanced mammography versus the combination of digital mammography and digital breast tomosynthesis in the preoperative assessment of breast cancer. Radiol Med 2021;126:1407-14. [Crossref] [PubMed]
- Granata V, Faggioni L, Grassi R, et al. Structured reporting of computed tomography in the staging of colon cancer: a Delphi consensus proposal. Radiol Med 2022;127:21-9. [Crossref] [PubMed]
- Meattini I, Palumbo I, Becherini C, et al. The Italian Association for Radiotherapy and Clinical Oncology (AIRO) position statements for postoperative breast cancer radiation therapy volume, dose, and fractionation. Radiol Med 2022;127:1407-11. [Crossref] [PubMed]
- Williams TR, Benjamin TGR, Schwartz MJ, et al. Narrative review-focal therapy: are we ready to change the prostate cancer treatment paradigm? Ann Transl Med 2023;11:24. [Crossref] [PubMed]
- D'Amico AV, Cormack RA, Tempany CM. MRI-guided diagnosis and treatment of prostate cancer. N Engl J Med 2001;344:776-7. [Crossref] [PubMed]
- Comet-Batlle J, Vilanova-Busquets JC, Saladié-Roig JM, et al. The value of endorectal MRI in the early diagnosis of prostate cancer. Eur Urol 2003;44:201-7; discussion 207-8. [Crossref] [PubMed]
- Kozlowski P, Chang SD, Jones EC, et al. Combined diffusion-weighted and dynamic contrast-enhanced MRI for prostate cancer diagnosis--correlation with biopsy and histopathology. J Magn Reson Imaging 2006;24:108-13. [Crossref] [PubMed]
- Costouros NG, Coakley FV, Westphalen AC, et al. Diagnosis of prostate cancer in patients with an elevated prostate-specific antigen level: role of endorectal MRI and MR spectroscopic imaging. AJR Am J Roentgenol 2007;188:812-6. [Crossref] [PubMed]
- Ibrahiem EI, Mohsen T, Nabeeh AM, et al. DWI-MRI: single, informative, and noninvasive technique for prostate cancer diagnosis. ScientificWorldJournal 2012;2012:973450. [Crossref] [PubMed]
- Turkbey B, Choyke PL. Multiparametric MRI and prostate cancer diagnosis and risk stratification. Curr Opin Urol 2012;22:310-5. [Crossref] [PubMed]
- Ghai S, Haider MA. Multiparametric-MRI in diagnosis of prostate cancer. Indian J Urol 2015;31:194-201. [Crossref] [PubMed]
- Rees J. MP-MRI could improve the diagnosis of prostate cancer. Practitioner 2017;261:5.
- Kasivisvanathan V, Emberton M, Moore CM. MRI-Targeted Biopsy for Prostate-Cancer Diagnosis. N Engl J Med 2018;379:589-90. [Crossref] [PubMed]
- Kasivisvanathan V, Rannikko AS, Borghi M, et al. MRI-Targeted or Standard Biopsy for Prostate-Cancer Diagnosis. N Engl J Med 2018;378:1767-77. [Crossref] [PubMed]
- Ma XZ, Lv K, Sheng JL, et al. Application evaluation of DCE-MRI combined with quantitative analysis of DWI for the diagnosis of prostate cancer. Oncol Lett 2019;17:3077-84. [Crossref] [PubMed]
- Dola EF, Nakhla OL, Genidi EA. Assessing the validity of Prostate Imaging Reporting and Data System version 2 (PI-RADS v2) scoring system in diagnosis of peripheral zone prostate cancer. Eur J Radiol Open 2017;4:19-26. [Crossref] [PubMed]
- Rosenkrantz AB, Verma S, Choyke P, et al. Prostate Magnetic Resonance Imaging and Magnetic Resonance Imaging Targeted Biopsy in Patients with a Prior Negative Biopsy: A Consensus Statement by AUA and SAR. J Urol 2016;196:1613-8. [Crossref] [PubMed]
- Chatterjee A, Harmath C, Oto A. New prostate MRI techniques and sequences. Abdom Radiol (NY) 2020;45:4052-62. [Crossref] [PubMed]
- Turkbey B, Haider MA. Artificial Intelligence for Automated Cancer Detection on Prostate MRI: Opportunities and Ongoing Challenges, From the AJR Special Series on AI Applications. AJR Am J Roentgenol 2022;219:188-94. [Crossref] [PubMed]
- Santone A, Brunese MC, Donnarumma F, et al. Radiomic features for prostate cancer grade detection through formal verification. Radiol Med 2021;126:688-97. [Crossref] [PubMed]
- Bicchetti M, Simone G, Giannarini G, et al. A novel pathway to detect muscle-invasive bladder cancer based on integrated clinical features and VI-RADS score on MRI: results of a prospective multicenter study. Radiol Med 2022;127:881-90. [Crossref] [PubMed]
- Brunese L, Brunese MC, Carbone M, et al. Automatic PI-RADS assignment by means of formal methods. Radiol Med 2022;127:83-9. [Crossref] [PubMed]
- Cipollari S, Pecoraro M, Forookhi A, et al. Biparametric prostate MRI: impact of a deep learning-based software and of quantitative ADC values on the inter-reader agreement of experienced and inexperienced readers. Radiol Med 2022;127:1245-53. [Crossref] [PubMed]
- Yao F, Bian S, Zhu D, et al. Machine learning-based radiomics for multiple primary prostate cancer biological characteristics prediction with 18F-PSMA-1007 PET: comparison among different volume segmentation thresholds. Radiol Med 2022;127:1170-8. [Crossref] [PubMed]
- Fei B. Computer-aided diagnosis of prostate cancer with MRI. Curr Opin Biomed Eng 2017;3:20-7. [Crossref] [PubMed]
- Transin S, Souchon R, Gonindard-Melodelima C, et al. Computer-aided diagnosis system for characterizing ISUP grade≥2 prostate cancers at multiparametric MRI: A cross-vendor evaluation. Diagn Interv Imaging 2019;100:801-11. [Crossref] [PubMed]
- Ellmann S, Schlicht M, Dietzel M, et al. Computer-Aided Diagnosis in Multiparametric MRI of the Prostate: An Open-Access Online Tool for Lesion Classification with High Accuracy. Cancers (Basel) 2020;12:2366. [Crossref] [PubMed]
- Calandrelli R, Boldrini L, Tran HE, et al. CT-based radiomics modeling for skull dysmorphology severity and surgical outcome prediction in children with isolated sagittal synostosis: a hypothesis-generating study. Radiol Med 2022;127:616-26. [Crossref] [PubMed]
- Caruso D, Polici M, Rinzivillo M, et al. CT-based radiomics for prediction of therapeutic response to Everolimus in metastatic neuroendocrine tumors. Radiol Med 2022;127:691-701. [Crossref] [PubMed]
- Cozzi D, Bicci E, Cavigli E, et al. Radiomics in pulmonary neuroendocrine tumours (NETs). Radiol Med 2022;127:609-15. [Crossref] [PubMed]
- Granata V, Fusco R, De Muzio F, et al. Radiomics and machine learning analysis based on magnetic resonance imaging in the assessment of liver mucinous colorectal metastases. Radiol Med 2022;127:763-72. [Crossref] [PubMed]
- Kao YS, Lin KT. A meta-analysis of the diagnostic test accuracy of CT-based radiomics for the prediction of COVID-19 severity. Radiol Med 2022;127:754-62. [Crossref] [PubMed]
- Shiradkar R, Podder TK, Algohary A, et al. Radiomics based targeted radiotherapy planning (Rad-TRaP): a computational framework for prostate cancer treatment planning with MRI. Radiat Oncol 2016;11:148. [Crossref] [PubMed]
- Stoyanova R, Pollack A, Takhar M, et al. Association of multiparametric MRI quantitative imaging features with prostate cancer gene expression in MRI-targeted prostate biopsies. Oncotarget 2016;7:53362-76. [Crossref] [PubMed]
- Stoyanova R, Takhar M, Tschudi Y, et al. Prostate cancer radiomics and the promise of radiogenomics. Transl Cancer Res 2016;5:432-47. [Crossref] [PubMed]
- Li M, Chen T, Zhao W, et al. Radiomics prediction model for the improved diagnosis of clinically significant prostate cancer on biparametric MRI. Quant Imaging Med Surg 2020;10:368-79. [Crossref] [PubMed]
- Roest C, Fransen SJ, Kwee TC, et al. Comparative Performance of Deep Learning and Radiologists for the Diagnosis and Localization of Clinically Significant Prostate Cancer at MRI: A Systematic Review. Life (Basel) 2022;12:1490. [Crossref] [PubMed]
- Yu R, Jiang KW, Bao J, et al. PI-RADS(AI): introducing a new human-in-the-loop AI model for prostate cancer diagnosis based on MRI. Br J Cancer 2023;128:1019-29. [Crossref] [PubMed]
- Weinreb JC, Barentsz JO, Choyke PL, et al. PI-RADS Prostate Imaging - Reporting and Data System: 2015, Version 2. Eur Urol. 2016;69:16-40. [Crossref] [PubMed]
- Turkbey B, Rosenkrantz AB, Haider MA, et al. Prostate Imaging Reporting and Data System Version 2.1: 2019 Update of Prostate Imaging Reporting and Data System Version 2. Eur Urol 2019;76:340-51. [Crossref] [PubMed]
- Palumbo P, Manetta R, Izzo A, et al. Biparametric (bp) and multiparametric (mp) magnetic resonance imaging (MRI) approach to prostate cancer disease: a narrative review of current debate on dynamic contrast enhancement. Gland Surg 2020;9:2235-47. [Crossref] [PubMed]
- Scialpi M, Scialpi P, Martorana E, et al. Biparametric MRI with simplified PI-RADS (S-PI-RADS) for prostate cancer detection and management: what do radiologist need to know. Radiol Med 2021;126:1660-1. [Crossref] [PubMed]
- Gaudiano C, Bianchi L, De Cinque A, et al. The impact of multiparametric MRI features to identify the presence of prevalent cribriform pattern in the peripheral zone tumors. Radiol Med 2022;127:174-82. [Crossref] [PubMed]
- Nardone V, Boldrini L, Salvestrini V, et al. Are you planning to be a radiation oncologist? A survey by the young group of the Italian Association of Radiotherapy and Clinical Oncology (yAIRO). Radiol Med 2023;128:252-60. [Crossref] [PubMed]
- Kang Z, Min X, Weinreb J, et al. Abbreviated Biparametric Versus Standard Multiparametric MRI for Diagnosis of Prostate Cancer: A Systematic Review and Meta-Analysis. AJR Am J Roentgenol 2019;212:357-65. [Crossref] [PubMed]
- Xu L, Zhang G, Shi B, et al. Comparison of biparametric and multiparametric MRI in the diagnosis of prostate cancer. Cancer Imaging 2019;19:90. [Crossref] [PubMed]
- Zhang J, Xu L, Zhang G, et al. Comparison between biparametric and multiparametric MRI diagnosis strategy for prostate cancer in the peripheral zone using PI-RADS version 2.1. Abdom Radiol (NY) 2022;47:2905-16. [Crossref] [PubMed]
- Vicini S, Bortolotto C, Rengo M, et al. A narrative review on current imaging applications of artificial intelligence and radiomics in oncology: focus on the three most common cancers. Radiol Med 2022;127:819-36. [Crossref] [PubMed]
- Ahmed SA, Samy M, Ali AM, et al. Architectural distortion outcome: digital breast tomosynthesis-detected versus digital mammography-detected. Radiol Med 2022;127:30-8. [Crossref] [PubMed]
- Bruno F, Marrelli A, Tommasino E, et al. Advanced MRI imaging of nerve roots in lumbar radiculopathy due to discoradicular conflict: DWI, DTI, and T2 mapping with clinical and neurophysiological correlations. Radiol Med 2022;127:1270-6. [Crossref] [PubMed]
- Cicero G, Alibrandi A, Blandino A, et al. DWI ratios: New indexes for Crohn's disease activity at magnetic resonance enterography? Radiol Med 2023;128:16-26. [Crossref] [PubMed]
- Gao W, Wang W, Song D, et al. A predictive model integrating deep and radiomics features based on gadobenate dimeglumine-enhanced MRI for postoperative early recurrence of hepatocellular carcinoma. Radiol Med 2022;127:259-71. [Crossref] [PubMed]
- Renzulli M, Brandi N, Argalia G, et al. Morphological, dynamic and functional characteristics of liver pseudolesions and benign lesions. Radiol Med 2022;127:129-44. [Crossref] [PubMed]
- Shannon BA, Ahlawat S, Morris CD, et al. Do contrast-enhanced and advanced MRI sequences improve diagnostic accuracy for indeterminate lipomatous tumors? Radiol Med 2022;127:90-9. [Crossref] [PubMed]
- White NS, McDonald C, Farid N, et al. Diffusion-weighted imaging in cancer: physical foundations and applications of restriction spectrum imaging. Cancer Res 2014;74:4638-52. [Crossref] [PubMed]
- Pozzessere C, Boudiaf M, Cirigliano A, et al. MR-enterography: role in the assessment of suspected anastomotic recurrence of Crohn disease after ileocolic resection. Radiol Med 2022;127:238-50. [Crossref] [PubMed]
- Zerunian M, Pucciarelli F, Caruso D, et al. Artificial intelligence based image quality enhancement in liver MRI: a quantitative and qualitative evaluation. Radiol Med 2022;127:1098-105. [Crossref] [PubMed]
- Bellardita L, Colciago RR, Frasca S, et al. Breast cancer patient perspective on opportunities and challenges of a genetic test aimed to predict radio-induced side effects before treatment: Analysis of the Italian branch of the REQUITE project. Radiol Med 2021;126:1366-73. [Crossref] [PubMed]
- Cellina M, Gibelli D, Martinenghi C, et al. Non-contrast magnetic resonance lymphography (NCMRL) in cancer-related secondary lymphedema: acquisition technique and imaging findings. Radiol Med 2021;126:1477-86. [Crossref] [PubMed]
- Cervelli R, Cencini M, Cacciato Insilla A, et al. Ex-vivo human pancreatic specimen evaluation by 7 Tesla MRI: a prospective radiological-pathological correlation study. Radiol Med 2022;127:950-9. [Crossref] [PubMed]
- Chiti G, Grazzini G, Flammia F, et al. Gastroenteropancreatic neuroendocrine neoplasms (GEP-NENs): a radiomic model to predict tumor grade. Radiol Med 2022;127:928-38. [Crossref] [PubMed]
- Gitto S, Bologna M, Corino VDA, et al. Diffusion-weighted MRI radiomics of spine bone tumors: feature stability and machine learning-based classification performance. Radiol Med 2022;127:518-25. [Crossref] [PubMed]
- Granata V, Simonetti I, Fusco R, et al. Management of cutaneous melanoma: radiologists challenging and risk assessment. Radiol Med 2022;127:899-911. [Crossref] [PubMed]
- Lo Casto A, Cannella R, Taravella R, et al. Diagnostic and prognostic value of magnetic resonance imaging in the detection of tumor depth of invasion and bone invasion in patients with oral cavity cancer. Radiol Med 2022;127:1364-72. [Crossref] [PubMed]
- Romano A, Moltoni G, Guarnera A, et al. Single brain metastasis versus glioblastoma multiforme: a VOI-based multiparametric analysis for differential diagnosis. Radiol Med 2022;127:490-7. [Crossref] [PubMed]
- Dai JC, Morgan TN, Goueli R, et al. MRI Features Associated with Histology of Benign Prostatic Hyperplasia Nodules: Generation of a Predictive Model. J Endourol 2022;36:381-6. [Crossref] [PubMed]
- Liu X, Zhou L, Peng W, et al. Differentiation of central gland prostate cancer from benign prostatic hyperplasia using monoexponential and biexponential diffusion-weighted imaging. Magn Reson Imaging 2013;31:1318-24. [Crossref] [PubMed]
- Liu Y, Wu J, Shen Q, et al. Magnetic resonance imaging features of prostatic stromal tumour of uncertain malignant potential. J Med Imaging Radiat Oncol 2022;66:1065-72. [Crossref] [PubMed]
- Quon JS, Moosavi B, Khanna M, et al. False positive and false negative diagnoses of prostate cancer at multi-parametric prostate MRI in active surveillance. Insights Imaging 2015;6:449-63. [Crossref] [PubMed]
- Xiaohang L, Bingni Z, Liangping Z, et al. Differentiation of prostate cancer and stromal hyperplasia in the transition zone with histogram analysis of the apparent diffusion coefficient. Acta Radiol 2017;58:1528-34. [Crossref] [PubMed]
- Zhou B, Liu X, Gan H, et al. Differentiation of Prostate Cancer and Stromal Hyperplasia in the Transition Zone With Monoexponential, Stretched-Exponential Diffusion-Weighted Imaging and Diffusion Kurtosis Imaging in a Reduced Number of b Values: Correlation With Whole-Mount Pathology. J Comput Assist Tomogr 2022;46:545-50. [Crossref] [PubMed]
- Tamada T, Ueda Y, Ueno Y, et al. Diffusion-weighted imaging in prostate cancer. MAGMA 2022;35:533-47. [Crossref] [PubMed]
- Turner R, Le Bihan D, Maier J, et al. Echo-planar imaging of intravoxel incoherent motion. Radiology 1990;177:407-14. [Crossref] [PubMed]
- Le Bihan D, Turner R, MacFall JR. Effects of intravoxel incoherent motions (IVIM) in steady-state free precession (SSFP) imaging: application to molecular diffusion imaging. Magn Reson Med 1989;10:324-37. [Crossref] [PubMed]
- Agarwal HK, Mertan FV, Sankineni S, et al. Optimal high b-value for diffusion weighted MRI in diagnosing high risk prostate cancers in the peripheral zone. J Magn Reson Imaging 2017;45:125-31. [Crossref] [PubMed]
- Godley KC, Syer TJ, Toms AP, et al. Accuracy of high b-value diffusion-weighted MRI for prostate cancer detection: a meta-analysis. Acta Radiol 2018;59:105-13. [Crossref] [PubMed]
- Hu L, Zhou DW, Zha YF, et al. Synthesizing High-b-value Diffusion-weighted Imaging of the Prostate Using Generative Adversarial Networks. Radiol Artif Intell 2021;3:e200237. [Crossref] [PubMed]
- Katahira K, Takahara T, Kwee TC, et al. Ultra-high-b-value diffusion-weighted MR imaging for the detection of prostate cancer: evaluation in 201 cases with histopathological correlation. Eur Radiol 2011;21:188-96. [Crossref] [PubMed]
- Kim CK, Park BK, Kim B. High-b-value diffusion-weighted imaging at 3 T to detect prostate cancer: comparisons between b values of 1,000 and 2,000 s/mm2. AJR Am J Roentgenol 2010;194:W33-7. [Crossref] [PubMed]
- Kitajima K, Kaji Y, Kuroda K, et al. High b-value diffusion-weighted imaging in normal and malignant peripheral zone tissue of the prostate: effect of signal-to-noise ratio. Magn Reson Med Sci 2008;7:93-9. [Crossref] [PubMed]
- Mazaheri Y, Hötker AM, Shukla-Dave A, et al. Model selection for high b-value diffusion-weighted MRI of the prostate. Magn Reson Imaging 2018;46:21-7. [Crossref] [PubMed]
- Rosenkrantz AB, Parikh N, Kierans AS, et al. Prostate Cancer Detection Using Computed Very High b-value Diffusion-weighted Imaging: How High Should We Go? Acad Radiol 2016;23:704-11. [Crossref] [PubMed]
- Tamada T, Kanomata N, Sone T, et al. High b value (2,000 s/mm2) diffusion-weighted magnetic resonance imaging in prostate cancer at 3 Tesla: comparison with 1,000 s/mm2 for tumor conspicuity and discrimination of aggressiveness. PLoS One 2014;9:e96619. [Crossref] [PubMed]
- Tamura C, Shinmoto H, Soga S, et al. Diffusion kurtosis imaging study of prostate cancer: preliminary findings. J Magn Reson Imaging 2014;40:723-9. [Crossref] [PubMed]
- Ueno Y, Kitajima K, Sugimura K, et al. Ultra-high b-value diffusion-weighted MRI for the detection of prostate cancer with 3-T MRI. J Magn Reson Imaging 2013;38:154-60. [Crossref] [PubMed]
- Wang X, Qian Y, Liu B, et al. High-b-value diffusion-weighted MRI for the detection of prostate cancer at 3 T. Clin Radiol 2014;69:1165-70. [Crossref] [PubMed]
- Wetter A, Nensa F, Lipponer C, et al. High and ultra-high b-value diffusion-weighted imaging in prostate cancer: a quantitative analysis. Acta Radiol 2015;56:1009-15. [Crossref] [PubMed]
- Yamada Y, Sakamoto S, Amiya Y, et al. Treatment strategy for metastatic prostate cancer with extremely high PSA level: reconsidering the value of vintage therapy. Asian J Androl 2018;20:432-7. [Crossref] [PubMed]
- Metens T, Miranda D, Absil J, et al. What is the optimal b value in diffusion-weighted MR imaging to depict prostate cancer at 3T? Eur Radiol 2012;22:703-9. [Crossref] [PubMed]
- Tamada T, Prabhu V, Li J, et al. Assessment of prostate cancer aggressiveness using apparent diffusion coefficient values: impact of patient race and age. Abdom Radiol (NY) 2017;42:1744-51. [Crossref] [PubMed]
- Raab P, Hattingen E, Franz K, et al. Cerebral gliomas: diffusional kurtosis imaging analysis of microstructural differences. Radiology 2010;254:876-81. [Crossref] [PubMed]
- Hansen B. An Introduction to Kurtosis Fractional Anisotropy. AJNR Am J Neuroradiol 2019;40:1638-41. [Crossref] [PubMed]
- Vaccaro AR, Koerner JD, Radcliff KE, et al. AOSpine subaxial cervical spine injury classification system. Eur Spine J 2016;25:2173-84. [Crossref] [PubMed]
- Wu X, Reinikainen P, Vanhanen A, et al. Correlation between apparent diffusion coefficient value on diffusion-weighted MR imaging and Gleason score in prostate cancer. Diagn Interv Imaging 2017;98:63-71. [Crossref] [PubMed]
- Neil JJ. Diffusion imaging concepts for clinicians. J Magn Reson Imaging 2008;27:1-7. [Crossref] [PubMed]
- Barrett T, Lawrence EM, Priest AN, et al. Repeatability of diffusion-weighted MRI of the prostate using whole lesion ADC values, skew and histogram analysis. Eur J Radiol 2019;110:22-9. [Crossref] [PubMed]
- Boss MA, Snyder BS, Kim E, et al. Repeatability and Reproducibility Assessment of the Apparent Diffusion Coefficient in the Prostate: A Trial of the ECOG-ACRIN Research Group (ACRIN 6701). J Magn Reson Imaging 2022;56:668-79. [Crossref] [PubMed]
- Tamada T, Huang C, Ream JM, et al. Apparent Diffusion Coefficient Values of Prostate Cancer: Comparison of 2D and 3D ROIs. AJR Am J Roentgenol 2018;210:113-7. [Crossref] [PubMed]
- Ueno Y, Tamada T, Sofue K, et al. Diffusion and quantification of diffusion of prostate cancer. Br J Radiol 2022;95:20210653. [Crossref] [PubMed]
- Fehr D, Veeraraghavan H, Wibmer A, et al. Automatic classification of prostate cancer Gleason scores from multiparametric magnetic resonance images. Proc Natl Acad Sci U S A 2015;112:E6265-73. [Crossref] [PubMed]
- Le Bihan D. Apparent diffusion coefficient and beyond: what diffusion MR imaging can tell us about tissue structure. Radiology 2013;268:318-22. [Crossref] [PubMed]
- Steven AJ, Zhuo J, Melhem ER. Diffusion kurtosis imaging: an emerging technique for evaluating the microstructural environment of the brain. AJR Am J Roentgenol 2014;202:W26-33. [Crossref] [PubMed]
- Jensen JH, Helpern JA, Ramani A, et al. Diffusional kurtosis imaging: the quantification of non-gaussian water diffusion by means of magnetic resonance imaging. Magn Reson Med 2005;53:1432-40. [Crossref] [PubMed]
- Langkilde F, Kobus T, Fedorov A, et al. Evaluation of fitting models for prostate tissue characterization using extended-range b-factor diffusion-weighted imaging. Magn Reson Med 2018;79:2346-58. [Crossref] [PubMed]
- Granata V, Fusco R, Belli A, et al. Diffusion weighted imaging and diffusion kurtosis imaging in abdominal oncological setting: why and when. Infect Agent Cancer 2022;17:25. [Crossref] [PubMed]
- Grinberg F, Farrher E, Ciobanu L, et al. Non-Gaussian diffusion imaging for enhanced contrast of brain tissue affected by ischemic stroke. PLoS One 2014;9:e89225. [Crossref] [PubMed]
- Kartalis N, Manikis GC, Loizou L, et al. Diffusion-weighted MR imaging of pancreatic cancer: A comparison of mono-exponential, bi-exponential and non-Gaussian kurtosis models. Eur J Radiol Open 2016;3:79-85. [Crossref] [PubMed]
- Suo S, Chen X, Wu L, et al. Non-Gaussian water diffusion kurtosis imaging of prostate cancer. Magn Reson Imaging 2014;32:421-7. [Crossref] [PubMed]
- Yao W, Zheng J, Han C, et al. Integration of quantitative diffusion kurtosis imaging and prostate specific antigen in differential diagnostic of prostate cancer. Medicine (Baltimore) 2021;100:e27144. [Crossref] [PubMed]
- Panagiotaki E, Chan RW, Dikaios N, et al. Microstructural characterization of normal and malignant human prostate tissue with vascular, extracellular, and restricted diffusion for cytometry in tumours magnetic resonance imaging. Invest Radiol 2015;50:218-27. [Crossref] [PubMed]
- Glenn GR, Tabesh A, Jensen JH. A simple noise correction scheme for diffusional kurtosis imaging. Magn Reson Imaging 2015;33:124-33. [Crossref] [PubMed]
- Iima M, Yano K, Kataoka M, et al. Quantitative non-Gaussian diffusion and intravoxel incoherent motion magnetic resonance imaging: differentiation of malignant and benign breast lesions. Invest Radiol 2015;50:205-11. [Crossref] [PubMed]
- Rosenkrantz AB, Sigmund EE, Winnick A, et al. Assessment of hepatocellular carcinoma using apparent diffusion coefficient and diffusion kurtosis indices: preliminary experience in fresh liver explants. Magn Reson Imaging 2012;30:1534-40. [Crossref] [PubMed]
- Choi JS, Kim MJ, Chung YE, et al. Comparison of breathhold, navigator-triggered, and free-breathing diffusion-weighted MRI for focal hepatic lesions. J Magn Reson Imaging 2013;38:109-18. [Crossref] [PubMed]
- Veraart J, Poot DH, Van Hecke W, et al. More accurate estimation of diffusion tensor parameters using diffusion Kurtosis imaging. Magn Reson Med 2011;65:138-45. [Crossref] [PubMed]
- Ueno Y, Takahashi S, Kitajima K, et al. Computed diffusion-weighted imaging using 3-T magnetic resonance imaging for prostate cancer diagnosis. Eur Radiol 2013;23:3509-16. [Crossref] [PubMed]
- Chabert S, Meca C, Le Bihan D. Relevance of the information about the diffusion distribution in vivo given by kurtosis in q-space imaging. Proceedings of the 12th Annual Meeting of ISMRM. Kyoto, Japan; 2004:1238.
- Rosenkrantz AB, Sigmund EE, Johnson G, et al. Prostate cancer: feasibility and preliminary experience of a diffusional kurtosis model for detection and assessment of aggressiveness of peripheral zone cancer. Radiology 2012;264:126-35. [Crossref] [PubMed]
- Altok M, Troncoso P, Achim MF, et al. Prostate cancer upgrading or downgrading of biopsy Gleason scores at radical prostatectomy: prediction of "regression to the mean" using routine clinical features with correlating biochemical relapse rates. Asian J Androl 2019;21:598-604. [Crossref] [PubMed]
- John A, O'Callaghan M, Catterwell R, et al. Does Gleason score of positive surgical margin after radical prostatectomy affect biochemical recurrence and oncological outcomes? Protocol for systematic review. BMJ Open 2020;10:e034612. [Crossref] [PubMed]
- Keller EX, Bachofner J, Britschgi AJ, et al. Prognostic value of unifocal and multifocal positive surgical margins in a large series of robot-assisted radical prostatectomy for prostate cancer. World J Urol 2019;37:1837-44. [Crossref] [PubMed]
- Hectors SJ, Semaan S, Song C, et al. Advanced Diffusion-weighted Imaging Modeling for Prostate Cancer Characterization: Correlation with Quantitative Histopathologic Tumor Tissue Composition-A Hypothesis-generating Study. Radiology 2018;286:918-28. [Crossref] [PubMed]
- Wu CJ, Zhang YD, Bao ML, et al. Diffusion Kurtosis Imaging Helps to Predict Upgrading in Biopsy-Proven Prostate Cancer With a Gleason Score of 6. AJR Am J Roentgenol 2017;209:1081-7. [Crossref] [PubMed]
- Rosenkrantz AB, Prabhu V, Sigmund EE, et al. Utility of diffusional kurtosis imaging as a marker of adverse pathologic outcomes among prostate cancer active surveillance candidates undergoing radical prostatectomy. AJR Am J Roentgenol 2013;201:840-6. [Crossref] [PubMed]
- Park H, Kim SH, Lee Y, et al. Comparison of diagnostic performance between diffusion kurtosis imaging parameters and mono-exponential ADC for determination of clinically significant cancer in patients with prostate cancer. Abdom Radiol (NY) 2020;45:4235-43. [Crossref] [PubMed]
- Shen L, Zhou G, Tang F, et al. MR diffusion kurtosis imaging for cancer diagnosis: A meta-analysis of the diagnostic accuracy of quantitative kurtosis value and diffusion coefficient. Clin Imaging 2018;52:44-56. [Crossref] [PubMed]
- Quentin M, Blondin D, Klasen J, et al. Comparison of different mathematical models of diffusion-weighted prostate MR imaging. Magn Reson Imaging 2012;30:1468-74. [Crossref] [PubMed]
- Roethke MC, Kuder TA, Kuru TH, et al. Evaluation of Diffusion Kurtosis Imaging Versus Standard Diffusion Imaging for Detection and Grading of Peripheral Zone Prostate Cancer. Invest Radiol 2015;50:483-9. [Crossref] [PubMed]
- Tamada T, Prabhu V, Li J, et al. Prostate Cancer: Diffusion-weighted MR Imaging for Detection and Assessment of Aggressiveness-Comparison between Conventional and Kurtosis Models. Radiology 2017;284:100-8. [Crossref] [PubMed]
- Maurer MH, Heverhagen JT. Diffusion weighted imaging of the prostate-principles, application, and advances. Transl Androl Urol 2017;6:490-8. [Crossref] [PubMed]
- Si Y, Liu RB. Diagnostic Performance of Monoexponential DWI Versus Diffusion Kurtosis Imaging in Prostate Cancer: A Systematic Review and Meta-Analysis. AJR Am J Roentgenol 2018;211:358-68. [Crossref] [PubMed]
- Lawrence EM, Warren AY, Priest AN, et al. Evaluating Prostate Cancer Using Fractional Tissue Composition of Radical Prostatectomy Specimens and Pre-Operative Diffusional Kurtosis Magnetic Resonance Imaging. PLoS One 2016;11:e0159652. [Crossref] [PubMed]
- Pecoraro M, Messina E, Bicchetti M, et al. The future direction of imaging in prostate cancer: MRI with or without contrast injection. Andrology 2021;9:1429-43. [Crossref] [PubMed]