Development of a nomogram for predicting survival of breast cancer patients with neoadjuvant chemotherapy: a dynamic analysis for systemic inflammation response index
Highlight box
Key findings
• Baseline systemic inflammation response index (SIRI) has a negative effect on disease-free survival (DFS) of breast cancer patients who receive neoadjuvant chemotherapy (NACT). Steady SIRI change after NACT is associated with prolonged DFS.
What is known and what is new?
• As an important inflammatory biomarker, SIRI has been reported to associate with survival in many cancers.
• The effects of baseline SIRI and SIRI change after NACT on survival outcomes in breast cancer have not been totally investigated, and our study not only evaluated the prognostic value of baseline SIRI, but also performed a dynamic analysis of SIRI after NACT.
What is the implication, and what should change now?
• The SIRI change after NACT might act as potential biomarkers for predicting survival outcomes in breast cancer. Future studies should pay more attention to the effects of the dynamic change of inflammatory biomarkers on survival in cancer.
Introduction
Breast cancer is the most prevalent malignant tumor in women worldwide, which causes hundreds of thousands of deaths per year (1). Neoadjuvant chemotherapy (NACT), as the standard treatment for locally advanced breast cancer, has been increasingly adopted. NACT not only facilitates locoregional treatment options, but also provides information on individual sensitivity to chemotherapy regimens (2). Identifying factors to predict the subgroup of breast cancer patients who would likely experience recurrence after NACT would improve stratification of patients for more appropriate and personalized treatment.
Pathological complete response (pCR) is a well-known and useful prognostic factor after NACT, and patients who achieve pCR have improved survival, especially for triple-negative or human epidermal growth factor receptor 2 (HER2)-positive breast cancer (3). Other common clinicopathological factors, such as tumor size, axillary lymph node status, and nuclear grade, have also been used to prognosticate patient survival outcomes (4-6). Besides, the systemic inflammatory biomarkers have been found to be of diagnostic value, including systemic immune-inflammation index (SIII), neutrophil to lymphocyte ratio (NLR), platelet to lymphocyte ratio (PLR), and lymphocyte to monocyte ratio (LMR) (7-11). Recently, the systemic inflammation response index (SIRI), which is based on neutrophil (N), monocyte (M), platelet (P), and lymphocyte (L) counts, was reported to be a prognostic factor in several types of cancer (12-14). Furthermore, SIRI has also been reported to be a predictive factor for pCR (15), with a low SIRI being associated with better survival than a high SIRI in breast cancer patients receiving NACT (16).
Intriguingly, the systemic inflammation and immune response during NACT are not static, and changes in the biomarkers between specific time points may be important for prognostication (17). However, the role of the change in SIRI after NACT in predicting breast cancer prognosis has not been investigated. This study aimed to further validate the role of baseline SIRI and explore the value of its change after NACT in predicting survival outcomes, and to establish a nomogram to help identify the high-risk patients who need more intensive treatment. We present this article in accordance with the TRIPOD reporting checklist (available at https://gs.amegroups.com/article/view/10.21037/gs-23-226/rc).
Methods
Patients
The study was conducted in accordance with the Declaration of Helsinki (as revised in 2013). The study was approved by the Ethics Committee of Sun Yat-sen University Cancer Center (No. B2021-268-01). The informed consent from patients was waived in this retrospective study. A total of 260 female patients diagnosed with breast carcinoma confirmed by preoperative biopsy between January 2014 and December 2018 at the Sun Yat-sen University Cancer Center were included. The inclusion criteria were as follows: (I) clinically non-metastatic disease at diagnosis; (II) patients accepted NACT followed by radical or breast conserving surgery, and surgery was performed approximately 2–3 weeks after the last cycle of NACT; (III) at least 4 NACT cycles were completed; and (IV) complete records of blood test before the NACT started and the operation. The exclusion criteria included the presence of active or chronic infection, hematological disorders, or autoimmune diseases.
According to the guideline of the Chinese Society of Clinical Oncology or the guideline of the National Comprehensive Cancer Network, the NACT regimens were based on anthracycline and taxanes. AC/EC→TH, which consisted of 4 cycles of doxorubicin (60 mg/m2 every 21 days) or epirubicin (90–100 mg/m2 every 21 days) and cyclophosphamide (600 mg/m2 every 21 days), followed by 2–4 cycles of docetaxel (100 mg/m2 every 21 days), and trastuzumab (8 mg/kg followed by 6 mg/kg every 21 days) was administered in patients with HER2 positivity; A/EC consisted of 4 cycles of doxorubicin (60 mg/m2 every 21 days) or epirubicin (100 mg/m2 every 21 days) and cyclophosphamide (600 mg/m2 every 21 days); TAC/TEC consisted of 4–6 cycles docetaxel (75 mg/m2 every 21 days), doxorubicin (50 mg/m2 every 21 days) plus cyclophosphamide (500 mg/m2 every 21 days).
Clinicopathologic characteristics
All the information was extracted from the electronic case management system of Sun Yat-sen University Cancer Center. The tumor-node-metastasis (TNM) stage was evaluated before starting NACT according to the 8th edition of the American Joint Committee on Cancer guidelines (18). The pre-NACT TNM stage was confirmed by the imaging examination before initiation of NACT. A pathological examination was conducted after surgery to confirm the pathological response, histological type, tumor grade, lymph vascular invasion (LVI), and lymph node status. pCR was defined as the absence of residual invasive tumor in the breast and lymph node (ypT0/TisN0).
Estrogen receptor (ER) and progestogen receptor (PR) were considered positive if immunohistochemistry (IHC) showed at least 1% tumor cells displaying nuclear staining. HER2 was considered as positive if IHC staining was 3+ or in situ hybridization was positive, androgen receptor (AR) was defined as positive when at least 1% tumor cells were stained. The molecular subtypes were divided into four groups: triple negative breast cancer (TNBC), ER, PR, and HER2 negative; HER2 positive type, ER and PR negative, HER2 positive; luminal A type, ER and/or PR positive with HER2 negative and Ki-67 <30%; luminal B type, ER and/or PR positive with HER2 positive or Ki-67 ≥30%.
The results of blood examination performed before and after NACT were collected respectively, the later blood examination was usually taken 2 weeks after the last cycle of NACT but before the surgery. The SIRI was calculated through the number of neutrophils multiplied by the number of monocytes and divided by the number of lymphocytes. The SIII was calculated through the number of neutrophils multiplied by the number of platelets and divided by the number of lymphocytes. The NLR was calculated through dividing the number of neutrophils by the number of lymphocytes. The PLR was calculated through dividing the number of platelets by the number of lymphocytes. The LMR was calculated through dividing the number of lymphocytes by the number of monocytes. The SIRI change was calculated as the SIRI after NACT minus baseline SIRI and divided by the baseline SIRI.
Follow-up
Disease free survival (DFS) was defined as the time from breast surgery to the cancer recurrence or the last follow-up of no evidence of cancer recurrence. The survival information was collected by searching the electronic follow-up recording system of our hospital.
Statistical analysis
The best cut-off values of baseline inflammatory biomarkers after NACT for predicting DFS were confirmed by the X-tile software (Yale School of Medicine, New Haven, CT, USA), which determines the cutoff value with the minimum P value from log-rank χ2 statistics for the categorical variables in terms of DFS (19). The calculated best cut-off values of baseline SIRI, baseline SIII, baseline NLR, baseline PLR, baseline MLR, and SIRI after NACT were 1.6×109, 376.4×109, 4.0, 94.1, 3.0, and 0.4×109, respectively. Chi-squared test or Fisher’s exact test was used to evaluate the relationship between clinicopathologic characteristics and baseline SIRI level, and the relationship between clinicopathologic characteristics and pCR status. Only P<0.05 was considered statistically significant. Univariate Cox regression analyses were performed to evaluate the effect of characteristics on DFS, and multivariate Cox regression analysis was then performed on the significant variables in the univariate analyses. A nomogram was established based on the significant factors in the multivariate Cox regression analysis, and the concordance index (C-index) was calculated to estimate the performance of the model. The consistency between the predicted probability of DFS and the actual probability of DFS was reflected by calibration curve plots. Statistical calculations were performed by the software SPSS 26.0 (IBM Corp., Armonk, NY, USA) and R (version 4.3.0; The R Foundation for Statistical Computing, Vienna, Austria). Kaplan-Meier survival analyses were also performed by GraphPad Prism 9 (GraphPad Software, San Diego, CA, USA).
Results
Patient characteristics
In total, 260 breast cancer patients were included. As shown in Table 1, the median age at diagnosis was 46.5 (range, 25–76) years, 176 (67.7%) were premenopausal, and 84 (32.3%) were postmenopausal. The pre-NACT clinical TNM stage of I–III was 1.9%, 20.0%, and 78.1%, respectively. Patients with molecular subtypes of TNBC, HER2 positive, luminal A, and luminal B were 40 (15.4%), 49 (18.8%), 71 (27.3%), and 100 (38.5%), respectively. After NACT, 242 (92.7%) patients underwent modified radical mastectomy, and others underwent breast conserving surgery. Among all participants, 25.0% had pCR and 75.0% had non-pCR.
Table 1
Characteristics | Number (percentage) or median [range] |
---|---|
Total | 260 (100.0) |
Age, years | 46.5 [25–76] |
Menopausal | |
Premenopausal | 176 (67.7) |
Postmenopausal | 84 (32.3) |
Pre-NACT T stage | |
T1 | 22 (8.5) |
T2 | 123 (47.3) |
T3 | 67 (25.7) |
T4 | 48 (18.5) |
Pre-NACT N stage | |
N0 | 28 (10.8) |
N1 | 51 (19.6) |
N2 | 90 (34.6) |
N3 | 91 (35.0) |
Pre-NACT TNM stage | |
I | 5 (1.9) |
II | 52 (20.0) |
III | 203 (78.1) |
ER | |
Negative | 96 (36.9) |
Positive | 164 (63.1) |
PR | |
Negative | 126 (48.5) |
Positive | 134 (51.5) |
HER2 | |
Negative | 149 (57.3) |
Positive | 111 (42.7) |
AR | |
Negative | 18 (6.9) |
Positive | 130 (50.0) |
Missing | 112 (43.1) |
Molecular subtype | |
TNBC | 40 (15.4) |
HER2 positive | 49 (18.8) |
Luminal A | 71 (27.3) |
Luminal B | 100 (38.5) |
Ki-67 | |
≥30% | 137 (52.7) |
<30% | 116 (44.6) |
Missing | 7 (2.7) |
Surgery | |
Mastectomy | 242 (92.7) |
Breast conserving | 18 (7.3) |
pCR | |
Yes | 65 (25.0) |
No | 195 (75.0) |
Chemotherapy regimen | |
AC/EC | 34 (13.1) |
TAC | 47 (18.1) |
AC/EC-T | 179 (68.8) |
pre-NACT, pre-neoadjuvant chemotherapy; TNM, tumor-node-metastasis; ER, estrogen receptor; PR, progesterone receptor; HER2, human epidermal growth factor receptor 2; AR, androgen receptor; TNBC, triple negative breast cancer; pCR, pathological complete response; A, doxorubicin; C, cyclophosphamide; E, epirubicin; T, docetaxel.
Comparison of patients with low SIRI versus high SIRI
According to the best cut-off value of SIRI, patients were divided into a low SIRI group (n=217, SIRI ≤1.6×109) and a high SIRI group (n=43, SIRI >1.6×109). The association between characteristics and the baseline SIRI level is shown in Table 2. In this study, baseline SIRI level was significantly correlated with tumor grade, ER and PR status, and molecular subtypes. Patients with a tumor grade of III tended to have higher proportion of high SIRI (22.5%) than those with a tumor grade of I–II (10.2%, P=0.028). Patients with ER/PR negativity had a higher proportion of high SIRI (25.0%, 23.0%) than those with ER/PR positivity (11.6%, 10.4%; P=0.005, P=0.006, respectively).
Table 2
Characteristics | Low SIRI, n (%) | High SIRI, n (%) | P value |
---|---|---|---|
Age | 0.072 | ||
≤50 years | 141 (80.6) | 34 (19.4) | |
>50 years | 76 (89.4) | 9 (10.6) | |
Menopausal | 1.000 | ||
Premenopausal | 132 (75.0) | 44 (25.0) | |
Postmenopausal | 63 (75.0) | 21 (25.0) | |
Pre-NACT T stage | 0.506 | ||
T1–2 | 123 (84.8) | 22 (15.2) | |
T3–4 | 94 (81.7) | 21 (18.3) | |
Pre-NACT N stage | 0.126 | ||
N0–1 | 71 (88.8) | 9 (11.2) | |
N2–3 | 146 (81.1) | 34 (18.9) | |
Pre-NACT TNM stage | 0.167 | ||
IA–IIB | 51 (89.5) | 6 (10.5) | |
IIIA–IIIC | 166 (81.8) | 37 (18.2) | |
Tumor grade | 0.028 | ||
I–II | 88 (89.8) | 10 (10.2) | |
III | 55 (77.5) | 16 (22.5) | |
LVI | 0.054 | ||
No | 121 (79.6) | 31 (20.4) | |
Yes | 82 (89.1) | 10 (10.9) | |
Pathological N stage | 0.081 | ||
N0–1 | 142 (80.7) | 34 (19.3) | |
N2–3 | 75 (89.3) | 9 (10.7) | |
ER | 0.005 | ||
Negative | 72 (75.0) | 24 (25.0) | |
Positive | 145 (88.4) | 19 (11.6) | |
PR | 0.006 | ||
Negative | 97 (77.0) | 29 (23.0) | |
Positive | 120 (89.6) | 14 (10.4) | |
HER2 | 0.579 | ||
Negative | 126 (84.6) | 23 (15.4) | |
Positive | 91 (82.0) | 20 (18.0) | |
Molecular subtype | 0.023 | ||
Luminal A | 61 (85.9) | 10 (14.1) | |
Luminal B | 90 (90.0) | 10 (10.0) | |
HER2 positive | 35 (71.4) | 14 (28.6) | |
TNBC | 31 (77.5) | 9 (22.5) | |
AR | 0.10 | ||
Negative | 13 (72.2) | 5 (27.8) | |
Positive | 116 (89.2) | 14 (10.8) | |
Ki-67 | 0.10 | ||
≥30% | 110 (80.3) | 27 (19.7) | |
<30% | 102 (87.9) | 14 (12.1) | |
pCR | 0.772 | ||
No | 162 (83.1) | 33 (16.9) | |
Yes | 55 (84.6) | 10 (15.4) |
SIRI, systemic inflammation response index; pre-NACT, pre-neoadjuvant chemotherapy; TNM, tumor-node-metastasis; LVI, lymph vascular invasion; ER, estrogen receptor; PR, progesterone receptor; HER2, human epidermal growth factor receptor 2; TNBC, triple negative breast cancer; AR, androgen receptor; pCR, pathological complete response.
Correlation between the clinicopathologic characteristics and pCR
Since pathological response after NACT was a crucial indicator for prognosis, we analyzed the relationship between some clinicopathologic factors and pCR. In our study, 65 (25%) patients achieved pCR after NACT. As shown in Table 3, patients with pre-NACT T stage of T1/T2 had a higher pCR rate than those with T stage of T3/T4 (31.0% and 17.4%, respectively; P=0.012). ER/PR negativity, and HER2 positivity were significantly associated with higher pCR rate (P<0.001, P=0.001, and P=0.001, respectively). The pCR rate of patients with TNBC, HER2 positivity, luminal A, and luminal B molecular subtype were 37.5%, 38.8%, 5.6%, and 27.0%, respectively (P<0.001). Patients with Ki-67 ≥30% had a higher pCR rate than those with Ki-67 <30% (30.7% and 15.5%, respectively; P=0.005). Unexpectedly, no significant correlation was found between the inflammatory biomarkers and pCR. When tested as continuous variables or categorical variables, none of the baseline SIRI/SIII/NLR/PLR/LMR values showed a statistically significant association with pCR (all P>0.05).
Table 3
Characteristics | Non-pCR, n (%) | pCR, n (%) | P value |
---|---|---|---|
Age | 0.939 | ||
≤50 years | 131 (74.9) | 44 (25.1) | |
>50 years | 64 (75.3) | 21 (24.7) | |
Menopausal | 1.000 | ||
Premenopausal | 132 (75.0) | 44 (25.0) | |
Postmenopausal | 63 (75.0) | 21 (25.0) | |
Pre-NACT T stage | 0.012 | ||
T1–2 | 100 (69.0) | 45 (31.0) | |
T3–4 | 95 (82.6) | 20 (17.4) | |
Pre-NACT N stage | 0.352 | ||
N0–1 | 57 (71.3 ) | 23 (28.8) | |
N2–3 | 138 (76.7) | 42 (23.3) | |
Pre-NACT TNM stage | 0.545 | ||
I–II | 41 (71.9) | 16 (28.1) | |
III | 154 (75.9) | 49 (24.1) | |
ER | <0.001 | ||
Negative | 58 (60.4) | 38 (39.6) | |
Positive | 137 (83.5) | 27 (16.5) | |
PR | 0.001 | ||
Negative | 83 (65.9) | 43 (34.1) | |
Positive | 112 (83.6) | 22 (16.4) | |
HER2 | 0.001 | ||
Negative | 123 (82.6) | 26 (17.4) | |
Positive | 72 (64.9) | 39 (35.1) | |
Molecular subtype | <0.001 | ||
TNBC | 25 (62.5) | 15 (37.5) | |
HER2 positive | 30 (61.2) | 19 (38.8) | |
Luminal A | 67 (94.4) | 4 (5.6) | |
Luminal B | 73 (73.0) | 27 (27.0) | |
AR | 0.526 | ||
Negative | 16 (88.9) | 2 (11.1) | |
Positive | 121 (93.1) | 9 (6.9) | |
Ki-67 | 0.005 | ||
≥30% | 95 (69.3) | 42 (30.7) | |
<30% | 98 (84.5) | 18 (15.5) | |
Baseline SIII | 0.846 | ||
≤376.4 | 32 (76.2) | 10 (23.8) | |
>376.4 | 163 (74.8) | 55 (25.2) | |
Baseline NLR | 0.725 | ||
≤4.0 | 174 (74.7) | 59 (25.3) | |
>4.0 | 21 (77.8) | 6 (22.2) | |
Baseline PLR | 0.191 | ||
≤94.1 | 21 (65.6) | 11 (34.4) | |
>94.1 | 174 (76.3) | 54 (23.7) | |
Baseline LMR | 0.922 | ||
<3.0 | 31 (75.6) | 10 (24.4) | |
≥3.0 | 164 (74.9) | 55 (25.1) |
pCR, pathological complete response; pre-NACT, pre-neoadjuvant chemotherapy; TNM, tumor-node-metastasis; ER, estrogen receptor; PR, progesterone receptor; HER2, human epidermal growth factor receptor 2; TNBC, triple negative breast cancer; AR, androgen receptor; SIII, systemic immune inflammation index; NLR, neutrophil to lymphocyte ratio; PLR, platelet to lymphocyte ratio; LMR, lymphocyte to monocyte ratio.
Univariate and multivariate Cox regression analyses
The median follow-up time was 40 months (range, 1–94 months); 74 (28.5%) patients had local or distant cancer recurrence during the follow-up. In univariate analysis, pre-NACT T stage, pre-NACT N stage, Ki-67, pCR, baseline SIRI, baseline NLR, and baseline LMR showed significant correlations with DFS (Table 4). The Kaplan-Meier survival analysis showed that patients with low SIRI had longer DFS than those with high SIRI (P=0.004, Figure 1A). Patients with lower NLR, higher LMR had better DFS than patients with higher NLR, lower LMR (P=0.029 and P=0.026, respectively, Figure 1B,1C).
Table 4
Characteristics | Univariate analysis | Multivariate analysis | |||||
---|---|---|---|---|---|---|---|
HR | 95% CI | P value | HR | 95% CI | P value | ||
Age (>50 vs. ≤50 years) | 1.084 | 0.66–1.77 | 0.749 | ||||
Menopausal status (premenopausal vs. postmenopausal) | 1.088 | 0.66–1.77 | 0.736 | ||||
Pre-NACT T stage (T1–2 vs. T3–4) | 0.614 | 0.39–0.97 | 0.036 | 0.801 | 0.50–1.29 | 0.363† | |
Pre-NACT N stage (N0–1 vs. N2–3) | 0.532 | 0.30–0.94 | 0.029 | 0.627 | 0.34–1.15 | 0.128† | |
Pre-NACT TNM stage (I–II vs. III) | 0.751 | 0.55–1.04 | 0.080 | ||||
ER (negative vs. positive) | 1.237 | 0.77–1.98 | 0.375 | ||||
PR (negative vs. positive) | 1.130 | 0.71–1.78 | 0.599 | ||||
HER2 (positive vs. negative) | 1.138 | 0.71–1.80 | 0.584 | ||||
AR (negative vs. positive) | 1.948 | 0.86–4.38 | 0.107 | ||||
Ki-67 (≥30% vs. <30%) | 1.647 | 1.02–2.65 | 0.041 | 0.606 | 0.37–0.99 | 0.049† | |
pCR (no vs. yes) | 2.243 | 1.18–4.26 | 0.014 | 2.390 | 1.19–4.79 | 0.014† | |
Baseline SIRI (≤1.6 vs. >1.6) | 0.473 | 0.28–0.80 | 0.005 | 0.545 | 0.32–0.94 | 0.028† | |
Baseline SIII (≤376.4 vs. >376.4) | 1.622 | 0.94–2.79 | 0.081 | ||||
Baseline NLR (≤4.0 vs. >4.0) | 0.508 | 0.27–0.94 | 0.032 | 0.585 | 0.30–1.14 | 0.114‡ | |
Baseline PLR (≤94.1 vs. >94.1) | 0.636 | 0.28–1.47 | 0.288 | ||||
Baseline LMR (<3.0 vs. ≥3.0) | 1.820 | 1.07–3.10 | 0.027 | 1.641 | 0.94–2.85 | 0.079§ |
†, the factors (pre-NACT T stage, pre-NACT N stage, Ki-67, pCR, and baseline SIRI) were evaluated in multivariate Cox regression analysis; ‡, the factors (pre-NACT T stage, pre-NACT N stage, Ki-67, pCR, and baseline NLR) were evaluated in multivariate Cox regression analysis; §, the factors (pre-NACT T stage, pre-NACT N stage, Ki-67, pCR, and baseline LMR) were evaluated in multivariate Cox regression analysis. DFS, disease-free survival; NACT, neoadjuvant chemotherapy; HR, hazard ratio; CI, confidence interval; pre-NACT, pre-neoadjuvant chemotherapy; TNM, tumor-node-metastasis; ER, estrogen receptor; PR, progesterone receptor; HER2, human epidermal growth factor receptor 2; AR, androgen receptor; pCR, pathological complete response; SIRI, systemic inflammatory response index; SIII, systemic immune inflammation index; NLR, neutrophil to lymphocyte ratio; PLR, platelet to lymphocyte ratio; LMR, lymphocyte to monocyte ratio.
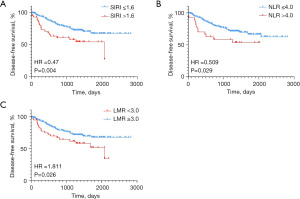
Pre-NACT T stage, Pre-NACT N stage, Ki-67, and pCR and SIRI, or NLR or LMR were tested in the multivariate Cox regression analysis separately. Remarkably, among the 3 inflammatory biomarkers, only SIRI was significantly associated with DFS both in the univariate and multivariate analysis [hazard ratio (HR) =0.545, 95% confidence interval (CI): 0.32–0.94, P=0.028].
Since SIRI was associated with breast cancer molecular subtypes, we further explored whether SIRI had a different influence on DFS in different molecular subtypes (Figure 2A-2D). The stratification analyses of molecular subtypes showed that SIRI only had statistically significant influence on DFS in TNBC.
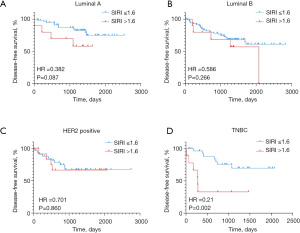
The relationship between SIRI after NACT and DFS
In consideration of the predictive value of baseline SIRI in survival outcomes of breast cancer patients, we further explored the role of SIRI after NACT and its change in predicting DFS. The Kaplan-Meier survival analysis showed that SIRI after NACT (≤0.4 vs. >0.4) was not significantly associated with DFS (HR =1.517, 95% CI: 0.758–3.0, P=0.169, Figure 3A). The minor SIRI change after NACT was defined as SIRI change ≤50% and ≥−30%, and the major SIRI change was defined as SIRI change >50% or <−30%. Intriguingly, the Kaplan-Meier survival analysis showed that patients with minor SIRI after NACT had longer DFS than those with major SIRI change after NACT (HR =1.758, 95% CI: 1.106–2.793, P=0.026, Figure 3B). Accordingly, the significant factors in the univariate analysis (Table 4) were included in the multivariate Cox regression analysis with SIRI change (Table 5). It showed that Ki-67, pCR, and SIRI change after NACT were independently associated with DFS.
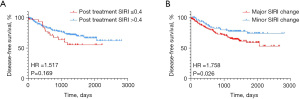
Table 5
Characteristics | Multivariate analysis | ||
---|---|---|---|
HR | 95% CI | P value | |
Pre-NACT T stage (T1–2 vs. T3–4) | 0.774 | 0.47–1.24 | 0.294 |
Pre-NACT N stage (N0–1 vs. N2–3) | 0.609 | 0.33–1.11 | 0.106 |
Ki-67 (<30% vs. ≥30%) | 0.546 | 0.33–0.89 | 0.016 |
pCR (no vs. yes) | 2.360 | 1.18–4.70 | 0.015 |
SIRI change after NACT (major vs. minor) | 1.721 | 1.03–2.86 | 0.037 |
SIRI, systemic inflammatory response index; DFS, disease free survival; HR, hazard ratio; CI, confidence interval; pre-NACT, pre-neoadjuvant chemotherapy; pCR, pathological complete response.
Nomogram to predict the probability of DFS
According to multivariate Cox regression analyses, statistically significant factors including Ki-67, pCR, and baseline SIRI or SIRI change were incorporated to build a nomogram for predicting the probability of 1-, 3-, and 5-year DFS in breast cancer patients (Figures 4,5). The C-index of the nomogram A based on Ki-67, pCR, and baseline SIRI was 0.665 (95% CI: 0.657–0.673), and the calibration plots showed relatively good consistency, especially for 3- and 5-year DFS (Figure 6A-6C). In addition, the C-index of the nomogram B based on Ki-67, pCR, and SIRI change after NACT was 0.663 (95% CI: 0.655–0.671), and the calibration plots showed relatively good consistency for 1- and 3-year DFS (Figure 6D-6F). To examine the critical role of SIRI, we further analyzed the prediction model without SIRI or SIRI change as predictive factors, and the C-index of the nomogram based on Ki-67 and pCR was 0.637 (95% CI: 0.63–0.644), which was lower than the nomograms based on Ki-67, pCR, and SIRI or SIRI change.
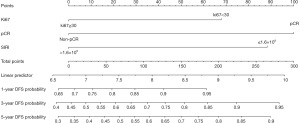
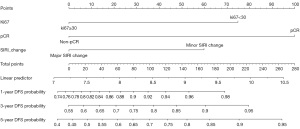
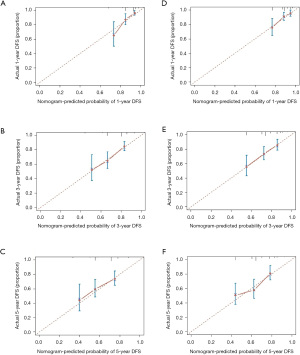
Discussion
The systemic inflammatory response and immune response have potential effects on cancer progression (20). Cancer-related inflammation has been considered a cancer hall marker (21). Previous studies suggested that systemic inflammatory markers, such as SIRI, SIII, NLR, PLR, and MLR, have prognostic or predictive roles in cancer (12,22,23). Particularly, SIRI was proposed to be a prognostic factor in many types of cancer, such as pancreatic cancer, cervical cancer, and gastric cancer (13,14,24,25). For breast cancer, SIRI was recently reported to associate with the pathological response and the survival outcome in neoadjuvant setting. In our study, baseline SIRI was further shown to be a predictive factor for DFS in breast cancer patients who received NACT. In addition, the role of SIRI change after NACT was explored, and it showed that patients with minor SIRI change had longer DFS than patients with major SIRI change.
A nomogram was then established based on the independent factors, namely Ki-67, pCR status, and baseline SIRI or SIRI change, which were low-cost, practical, and convenient for clinical use. Although the C-index of nomograms A and B were 0.665 and 0.663 respectively, concluding as not highly reliable, the calibration plots of nomogram A showed good consistency between the predicted and actual 3- and 5-year DFS, and nomogram B showed good consistency between the predicted and actual 1- and 3-year DFS. The nomogram could be a reference for clinicians in evaluating the patients’ probability of DFS after NACT.
The achievement of pCR has been clearly demonstrated to associate with better survival, and pathological response helps to evaluate the patients’ sensitivity to chemotherapy drugs. Therefore, it is meaningful to investigate the relationship between the clinical factors and pCR. We first analyzed the common factors and found that the earlier pre-NACT T stage and Ki-67 ≥30 associated with higher pCR rate. It was understandable that a smaller tumor would have better regression after NACT, and tumors with higher Ki-67 possessing active proliferative capacity were vulnerable to chemotherapy drugs. Tumors with ER/PR negativity, and HER2 positivity had a higher pCR rate. This may be explained by the intrinsic property of TNBC which is highly proliferative, and the use of trastuzumab improved the pathological response of HER2-positive patients. Regarding the inflammatory biomarkers, although previous work had reported the connections between them and pCR, we did not find any statistically significant correlation between pCR and baseline SIRI, SIII, NLR, PLR, or LMR in the current study.
Dong et al. reported that patients with SIRI lower than 0.72×109 had a higher pCR rate (15), and Chen et al. reported that patients with low SIRI (<0.85×109) had longer DFS and overall survival (OS) than those with high SIRI (≥0.85×109) (16). In our study, patients who received NACT with lower baseline SIRI (≤1.6×109) had longer DFS. Despite the different cut-off value of SIRI, these trends were consistent with our results. In addition, patients with minor SIRI change (≤50% and ≥−30%) had longer DFS than those with major SIRI change (>50% or <−30%). We initially supposed that lower and decreased SIRI level during NACT would be a favorable factor for patients’ survival. However, subtle increase of SIRI did not impact the survival. On the contrary, radical decreased or increased SIRI was associated with poor survival. Similarly, in Lee’s study, patients with modest NLR change after NACT (−30%< NLR change <100%) showed prolonged DFS (26). In Choi’s study, it was indicated that the change between baseline NLR and NLR after 2 cycles of NACT was a prognostic factor, and patients with changes in NLR over 0.1258 had a tendency to poorer prognosis (17). Radical changes of SIRI might be unfavorable for anti-tumor activity; further studies are needed to confirm the hypothesis.
Indeed, the underlying mechanisms behind the role of SIRI levels during treatment process and survival outcomes in cancer patients are poorly understood. SIRI is calculated as N*M/L and that N represents peripheral neutrophil, M represents peripheral monocyte, and L represents peripheral lymphocyte. Peripheral neutrophil was an important contributor for defending against infection and regulating innate and adaptive immunity (27). The neutrophils in the tumor micro-environment have been reported to exert both pro-tumor and anti-tumor effects (28). In most cases, a high level of neutrophils in the tumor micro-environment or neutrophils in the peripheral blood were associated with poor prognosis in cancer (27). Peripheral monocytes have been reported to be associated with the tumor-associated macrophages, which contributed to the progression of tumor (29). Peripheral lymphocytes have been shown to activate the adaptive immune response against tumors (30). In addition, a high level of tumor-infiltrating lymphocytes has been reported to associate with favorable survival in breast cancer (31). In general, SIRI reflects the balance between host inflammatory response and immune response. This could in part explain that the low level of baseline SIRI was associated with prolonged DFS. However, elevated neutrophils or monocytes, or decreased lymphocytes during treatment cannot be considered as a universal unfavorable sign for cancer progression, because the general inflammatory state can be caused by some pathological or constitutional conditions (32). Since chemotherapy drugs and symptomatic therapy during NACT could stimulate complex and diverse responses of the organism. Conversely, the relationship between peripheral blood cells and tumor-associated infiltrating cells is not clear. Currently, the results still need to be verified in additional studies.
Previous articles have reported the different roles of inflammatory biomarkers in predicting pCR and survival outcome in the different breast cancer molecular subtypes (33). A meta-analysis found that NLR was only significantly associated with pCR in patients with TNBC, but not in luminal subtype (34). However, statistically significant correlations between NLR level and DFS have been observed in TNBC and luminal subtypes (35,36). In our study, we explored the role of baseline SIRI in predicting DFS in 4 molecular subtypes. Similarly, patients in our study with lower SIRI tended to have prolonged DFS, but statistical significance was only observed in the TNBC group. This would be explained by the limitation of statistical analyses due to the small number of patients in each subtype in our study. This could possibly be related to the intrinsic features of TNBC. The exact functions of baseline SIRI in different molecular subtypes deserve further validation.
There are several limitations in our study. Firstly, this was a retrospective study, so the population and data collection may have been biased. Additional prospective studies are warranted to verify the results. Secondly, due to the limited follow-up period, the correlation between the parameters and OS could not be analyzed. Thirdly, the uses of granulocyte colony-stimulating factor (GCSF) during NACT were not recorded completely. Thus, we could not evaluate the effects of GCSF on the blood cells’ value.
Conclusions
In this study, we found increased associations between low level of baseline SIRI and prolonged DFS; the minor SIRI change after NACT was associated with prolonged DFS. This indicated that baseline SIRI and SIRI change after NACT were potential prognostic biomarkers in breast cancer patients who received NACT. In addition, the nomogram could provide references for clinicians in evaluating the patients’ probability of DFS after NACT. Further work is warranted to validate its authenticity and reliability in clinical practice.
Acknowledgments
Funding: None.
Footnote
Reporting Checklist: The authors have completed the TRIPOD reporting checklist. Available at https://gs.amegroups.com/article/view/10.21037/gs-23-226/rc
Data Sharing Statement: Available at https://gs.amegroups.com/article/view/10.21037/gs-23-226/dss
Peer Review File: Available at https://gs.amegroups.com/article/view/10.21037/gs-23-226/prf
Conflicts of Interest: All authors have completed the ICMJE uniform disclosure form (available at https://gs.amegroups.com/article/view/10.21037/gs-23-226/coif). The authors have no conflicts of interest to declare.
Ethical Statement: The authors are accountable for all aspects of the work in ensuring that questions related to the accuracy or integrity of any part of the work are appropriately investigated and resolved. The study was conducted in accordance with the Declaration of Helsinki (as revised in 2013). The study was approved by the Ethics Committee of Sun Yat-sen University Cancer Center, approval code: B2021-268-01. The informed consent from patients was waived in this retrospective study.
Open Access Statement: This is an Open Access article distributed in accordance with the Creative Commons Attribution-NonCommercial-NoDerivs 4.0 International License (CC BY-NC-ND 4.0), which permits the non-commercial replication and distribution of the article with the strict proviso that no changes or edits are made and the original work is properly cited (including links to both the formal publication through the relevant DOI and the license). See: https://creativecommons.org/licenses/by-nc-nd/4.0/.
References
- Sung H, Ferlay J, Siegel RL, et al. Global Cancer Statistics 2020: GLOBOCAN Estimates of Incidence and Mortality Worldwide for 36 Cancers in 185 Countries. CA Cancer J Clin 2021;71:209-49. [Crossref] [PubMed]
- von Minckwitz G, Blohmer JU, Costa SD, et al. Response-guided neoadjuvant chemotherapy for breast cancer. J Clin Oncol 2013;31:3623-30. [Crossref] [PubMed]
- Cortazar P, Zhang L, Untch M, et al. Pathological complete response and long-term clinical benefit in breast cancer: the CTNeoBC pooled analysis. Lancet 2014;384:164-72. [Crossref] [PubMed]
- Vila J, Teshome M, Tucker SL, et al. Combining Clinical and Pathologic Staging Variables Has Prognostic Value in Predicting Local-regional Recurrence Following Neoadjuvant Chemotherapy for Breast Cancer. Ann Surg 2017;265:574-80. [Crossref] [PubMed]
- Mittendorf EA, Vila J, Tucker SL, et al. The Neo-Bioscore Update for Staging Breast Cancer Treated With Neoadjuvant Chemotherapy: Incorporation of Prognostic Biologic Factors Into Staging After Treatment. JAMA Oncol 2016;2:929-36. [Crossref] [PubMed]
- Sheri A, Smith IE, Johnston SR, et al. Residual proliferative cancer burden to predict long-term outcome following neoadjuvant chemotherapy. Ann Oncol 2015;26:75-80. [Crossref] [PubMed]
- Li Y, Shao Y, Bai L, et al. Increased derived neutrophil-to-lymphocyte ratio and Breast Imaging-Reporting and Data System classification predict poor survival in patients with non-distant metastatic HER2+ breast cancer treated with neoadjuvant chemotherapy. Cancer Manag Res 2018;10:3841-7. [Crossref] [PubMed]
- Lusho S, Durando X, Mouret-Reynier MA, et al. Platelet-to-Lymphocyte Ratio Is Associated With Favorable Response to Neoadjuvant Chemotherapy in Triple Negative Breast Cancer: A Study on 120 Patients. Front Oncol 2021;11:678315. [Crossref] [PubMed]
- Muñoz-Montaño W, Cabrera-Galeana P, Alvarado-Miranda A, et al. Prognostic Value of the Pretreatment Neutrophil-to-Lymphocyte Ratio in Different Phenotypes of Locally Advanced Breast Cancer During Neoadjuvant Systemic Treatment. Clin Breast Cancer 2020;20:307-316.e1. [Crossref] [PubMed]
- Savioli F, Morrow ES, Dolan RD, et al. Prognostic role of preoperative circulating systemic inflammatory response markers in primary breast cancer: meta-analysis. Br J Surg 2022;109:1206-15. [Crossref] [PubMed]
- Chen L, Kong X, Wang Z, et al. Pre-treatment systemic immune-inflammation index is a useful prognostic indicator in patients with breast cancer undergoing neoadjuvant chemotherapy. J Cell Mol Med 2020;24:2993-3021. [Crossref] [PubMed]
- Yu L, Zheng S, Yang J, et al. Association between the systemic immune inflammation index and recurrence or metastasis after interventional therapy in patients with primary liver cancer- a retrospective cohort study. J Gastrointest Oncol 2023;14:780-8. [Crossref] [PubMed]
- Kim JS, Choi M, Kim SH, et al. Systemic inflammation response index correlates with survival and predicts oncological outcome of resected pancreatic cancer following neoadjuvant chemotherapy. Pancreatology 2022;22:987-93. [Crossref] [PubMed]
- Chao B, Ju X, Zhang L, et al. A Novel Prognostic Marker Systemic Inflammation Response Index (SIRI) for Operable Cervical Cancer Patients. Front Oncol 2020;10:766. [Crossref] [PubMed]
- Dong J, Sun Q, Pan Y, et al. Pretreatment systemic inflammation response index is predictive of pathological complete response in patients with breast cancer receiving neoadjuvant chemotherapy. BMC Cancer 2021;21:700. [Crossref] [PubMed]
- Chen L, Kong X, Wang Z, et al. Pretreatment Systemic Inflammation Response Index in Patients with Breast Cancer Treated with Neoadjuvant Chemotherapy as a Useful Prognostic Indicator. Cancer Manag Res 2020;12:1543-67. [Crossref] [PubMed]
- Choi H, Noh H, Cho IJ, et al. Changes in neutrophil to lymphocyte ratio (NLR) during neoadjuvant treatment correlated with patients' survival. Breast Cancer 2020;27:871-9. [Crossref] [PubMed]
- Giuliano AE, Connolly JL, Edge SB, et al. Breast Cancer-Major changes in the American Joint Committee on Cancer eighth edition cancer staging manual. CA Cancer J Clin 2017;67:290-303.
- Camp RL, Dolled-Filhart M, Rimm DL. X-tile: a new bio-informatics tool for biomarker assessment and outcome-based cut-point optimization. Clin Cancer Res 2004;10:7252-9. [Crossref] [PubMed]
- Balkwill F, Mantovani A. Inflammation and cancer: back to Virchow? Lancet 2001;357:539-45. [Crossref] [PubMed]
- Mantovani A, Allavena P, Sica A, et al. Cancer-related inflammation. Nature 2008;454:436-44. [Crossref] [PubMed]
- Gu Q, Zhao J, Liu Y, et al. Association between the systemic immune-inflammation index and the efficacy of neoadjuvant chemotherapy, prognosis in HER2 positive breast cancer-a retrospective cohort study. Gland Surg 2023;12:609-18. [Crossref] [PubMed]
- Han R, Tian Z, Jiang Y, et al. Prognostic significance of systemic immune-inflammation index and platelet-albumin-bilirubin grade in patients with pancreatic cancer undergoing radical surgery. Gland Surg 2022;11:576-87. [Crossref] [PubMed]
- Li S, Lan X, Gao H, et al. Systemic Inflammation Response Index (SIRI), cancer stem cells and survival of localised gastric adenocarcinoma after curative resection. J Cancer Res Clin Oncol 2017;143:2455-68. [Crossref] [PubMed]
- Zhang Y, Liu F, Wang Y. Evidence of the Prognostic Value of Pretreatment Systemic Inflammation Response Index in Cancer Patients: A Pooled Analysis of 19 Cohort Studies. Dis Markers 2020;2020:8854267. [Crossref] [PubMed]
- Lee J, Kim DM, Lee A. Prognostic Role and Clinical Association of Tumor-Infiltrating Lymphocyte, Programmed Death Ligand-1 Expression with Neutrophil-Lymphocyte Ratio in Locally Advanced Triple-Negative Breast Cancer. Cancer Res Treat 2019;51:649-63. [Crossref] [PubMed]
- Jaillon S, Ponzetta A, Di Mitri D, et al. Neutrophil diversity and plasticity in tumour progression and therapy. Nat Rev Cancer 2020;20:485-503. [Crossref] [PubMed]
- Carnevale S, Ghasemi S, Rigatelli A, et al. The complexity of neutrophils in health and disease: Focus on cancer. Semin Immunol 2020;48:101409. [Crossref] [PubMed]
- Yamamoto T, Kawada K, Obama K. Inflammation-Related Biomarkers for the Prediction of Prognosis in Colorectal Cancer Patients. Int J Mol Sci 2021;22:8002. [Crossref] [PubMed]
- Notarbartolo S, Abrignani S. Human T lymphocytes at tumor sites. Semin Immunopathol 2022;44:883-901. [Crossref] [PubMed]
- Denkert C, von Minckwitz G, Darb-Esfahani S, et al. Tumour-infiltrating lymphocytes and prognosis in different subtypes of breast cancer: a pooled analysis of 3771 patients treated with neoadjuvant therapy. Lancet Oncol 2018;19:40-50. [Crossref] [PubMed]
- Truffi M, Sottotetti F, Gafni N, et al. Prognostic Potential of Immune Inflammatory Biomarkers in Breast Cancer Patients Treated with Neoadjuvant Chemotherapy. Cancers (Basel) 2022;14:5287. [Crossref] [PubMed]
- Zenan H, Zixiong L, Zhicheng Y, et al. Clinical prognostic evaluation of immunocytes in different molecular subtypes of breast cancer. J Cell Physiol 2019;234:20584-602. [Crossref] [PubMed]
- Zhou Q, Dong J, Sun Q, et al. Role of neutrophil-to-lymphocyte ratio as a prognostic biomarker in patients with breast cancer receiving neoadjuvant chemotherapy: a meta-analysis. BMJ Open 2021;11:e047957. [Crossref] [PubMed]
- Koh YW, Lee HJ, Ahn JH, et al. Prognostic significance of the ratio of absolute neutrophil to lymphocyte counts for breast cancer patients with ER/PR-positivity and HER2-negativity in neoadjuvant setting. Tumour Biol 2014;35:9823-30. [Crossref] [PubMed]
- Asano Y, Kashiwagi S, Onoda N, et al. Predictive Value of Neutrophil/Lymphocyte Ratio for Efficacy of Preoperative Chemotherapy in Triple-Negative Breast Cancer. Ann Surg Oncol 2016;23:1104-10. [Crossref] [PubMed]
(English Language Editor: J. Jones)