Analysis of CD4+ T-helper-associated hub gene signature and immune dysregulation via RNA-sequencing data in a mouse tail model of lymphedema
Highlight box
Key findings
• Biological pathways associated with T-helpers were significantly enriched in a mouse tail model of lymphedema by bioinformatics analysis.
• The study identified eight T-helper-associated hub genes, including Cd4, Foxp3, Irf4, Ccr6, Il12rb1, Batf, Il1b, and Cd74.
What is known and what is new?
• It was already known that T-helpers played essential roles in lymphedema.
• The present study identified a novel list of T-helper-associated hub genes with the ability to diagnose lymphedema and characterize immunological alterations in lymphedema.
What is the implication, and what should change now?
• T-helper-associated biological regulators are targets of immunoregulatory drugs for lymphedema.
Introduction
Secondary lymphedema is a chronic, disabling condition that impairs patients’ quality of life in addition to causing swelling, pain, and susceptibility to infection (1). Currently, lymphatic insufficiency brought on by tumor excision and tumor-associated therapies like chemotherapy and radiotherapy is the pivot reason for lymphedema (2,3). It is well established that complete decongestive therapy (4) and physical lymphatic surgery like lymph-venous anastomosis (5), and vascularized lymph node transfer (6) show partial therapeutic effects on lymphedema; there is, however, no known cure for it. Inflammation, epidermal thickness, lipid accumulation, tissue fibrosis, and lymphatic dysfunction are the pathologies of secondary lymphedema (7-9). High interstitial protein levels in lymph fluid do not directly cause these pathological alterations (10), indicating that further processes are needed for lymphedema to progress. The critical condition for early diagnosis and better treatment relies on the in-depth clarity of the basic pathology of secondary lymphedema.
Recent evidence suggests that changes in the local immunological environment play a central role in these pathological processes, of which CD4+ T-helper cells (Th) are considered to be crucial immune activation mediators (11-14). T-helpers could be further subclassified into T-helper 1 (Th1), T-helper 2 (Th2), T-helper 17 (Th17), Regulatory T cells (Tregs), and T follicular helper cells (Tfh) (15). Mounting evidence has accumulated that Th2 cells promote fibrosis, and down-regulate lymphangiogenesis, and lymphatic function in lymphedema while Th1 cells co-regulate the fibrotic process and lymphangiogenesis (16-19). Besides, adoptive Tregs could relieve the dysfunctional lymphatics in mouse lymphedema (14). Interleukin (IL)-17A, secreted mainly by Th17 cells, suppressed the expression of lymphatic endothelial markers and negatively regulated the formation of lymphangiogenesis (20). However, how and why the helper T cells were activated was beyond our knowledge. Thus, it is significant to systematically investigate Th-associated biological alterations, hub genes, and transcription factors (TFs) in lymphedema, which can provide an in-depth understanding of the underlying mechanisms in secondary lymphedema.
To further study the Th-associated pathogenesis of lymphedema, we systematically conducted a bioinformatics investigation of RNA-sequencing (RNA-seq) data of mouse tail lymphedema, including the transcriptomic profile of mouse tail lymphedema, which was induced by surgical destruction of tail lymphatics of the mouse, at 2 and 6 weeks (n=5, n=4, separately) post-operatively versus control un-operated mice (n=5). Initially, the T-helper cell-associated genes (THAGs) were identified and T-helper-associated hub genes (THAHGs) were constructed. Single-sample gene set enrichment analysis (ssGSEA) showcased the differences in immune cell infiltration. The immune processes of T-helpers, macrophages, B cells, and gamma-delta T cells were next analyzed by GSEA (gene set enrichment analysis). In addition, TFs targeting THAHGs and the relative immune-regulatory drugs were predicted in lymphedema. We present this article in accordance with the STREGA reporting checklist (available at https://gs.amegroups.com/article/view/10.21037/gs-23-48/rc).
Methods
Public data collection and processing
Briefly, we downloaded the raw data profile with the project number PRJEB15150 from the European Nucleotide Archive (ENA) website (https://www.ebi.ac.uk/ena/browser/). The collecting lymphatic veins and a 3–4 mm circular section of tail tissue at 2 cm from the base of the tail were removed to construct the mouse model of lymphedema (21), which lasted for at least 6 weeks. The datasets contained 5 samples of tail skin from normal mice (unoperated), and 5 from lymphedema mice at 2 weeks after surgical lymphatic obstruction) and 4 from lymphedema mice at 6 weeks post-surgically. To obtain the cleaned counts of RNA data, the raw data was first quality-controlled using the “fastqc” software, then trimmed using “trim-galore”, mapped using “hisat2” and “qualimap”, and last quantified using “featureCounts” on a Linux environment. The study design is illustrated in Figure 1.
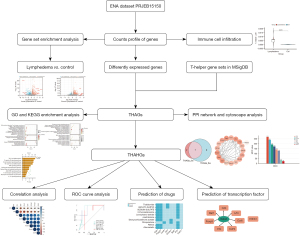
Differentially expressed genes (DEGs)
The “DESeq2” package of R software (version 4.2.1) was used to screen for DEGs in acute lymphedema group and the control group, as well as chronic lymphedema group and the control group (22). The selection criteria were |log2 [fold change (FC)]| >1.5, and false discovery rate (FDR) <0.05. The volcano plots were generated by the ‘ggplot2’package (version 3.4.0).
Gene Ontology (GO) analysis and Kyoto Encyclopedia of Genes and Genomes (KEGG) pathway enrichment analyses of genes
GO analysis and KEGG pathway enrichment analysis were conducted using the ‘ClusterProfiler’ R package (version 4.4.4) (23). GO analysis included three criteria: the biological process (BP), cellular component (CC), and molecular function (MF) categories. The “adjusted P value (from the Benjamini-Hochberg method) <0.05” was considered statistically significant.
THAG set collection and construction of THAGs list
To explore the helper T cell-associated biological characteristics in lymphedema, we searched the Molecular Signatures Database (http://www.gsea-msigdb.org/gsea/msigdb/mouse/search.jsp) with “T_helper” as the keyword without additional filtering condition, which returned 31 THAGs sets containing 129 THAGs. The 129 genes overlapping with the DEGs were defined as THAGs in lymphedema.
Generation of THAHGs from protein-protein interaction (PPI) network analysis
The PPI network of the THAGs was constructed via the STRING database (https://www.string-db.org/). The critical subnetwork and hub genes in the PPI network were further identified utilizing the Molecular Complex Detection (MCODE) plug-in application of Cytoscape software (version 3.9.1) (24,25).
Immune cell infiltration
Based on gene expression profiles, the immune cell composition of the samples was inferred using the Immune Cell Abundance Identifier for Mouse (ImmuCellAI-mouse) tool (26). Thirty-six immune cells from three layers can be analyzed by ImmuCellAI-mouse to determine their relative abundance. Three different lymphoid cell types (B cell, NK cell, and T cell), as well as four different myeloid cell types, were present in layer 1 including macrophage, DC, monocyte, and granulocyte. Cells in layer 2 were subtypes of cells in layer 1 whereas cells in layer 3 were subtypes of CD4+ T and CD8+ T cells.
GSEA analysis of pathways associated with T-helpers, macrophages, B cells, and gamma-delta T cells
To pathways of T-helpers, macrophages, B cells, and gamma-delta T cells were searched and downloaded in the Molecular Signatures Database (27,28). GSEA was performed on gene sets associated with the four distinct immune cells via GSEA software (29,30) to identify markedly enriched biological pathways in lymphedema. Permutations were set to 10,000 to obtain normalized enrichment scores (NESs) in GSEA. Gene sets with NES >1, nominal P value <0.05, and FDR <0.25 were considered significantly enriched.
Correlation analysis of THAHGs
We used the R “ggstatsplot” package to display a correlation matrix between THAHGs, THAHGs, and immune cell infiltration (31). P value <0.05 was considered to be statistically significant.
Receiver operating characteristic (ROC) curve analysis of THAHGs
The diagnostic value of the THAHGs was estimated via ROC curve and area under curve (AUC) analysis, which was performed via the “pROC” package (32). AUC (0.5–0.7) means low accuracy, AUC (0.7–0.9) meant a certain accuracy, and AUC (> 0.9) means high accuracy.
Prediction of TF and therapeutic drugs related to THAHGs
The iRegulon (Version: 1.3) plugin was utilized to screen key TF with the default cutoff criteria (33). Protein-drug interaction data from the DSigDB (http://tanlab.ucdenver.edu/DSigDB) database were used to predict potential therapeutic agents for lymphedema, with FDR <0.05 and composite score >5,000 used as thresholds.
Statistical analysis
R 4.2.1 software was used for data processing, statistical analysis, and graphing. For comparisons of two groups, significance was determined using the Student’s t-test or the Wilcoxon rank-sum test, and the Kruskal-Wallis test for comparisons of three groups or more. Pearson correlations were used to detect the relationships between two continuous variables. The statistical significance level was set at P<0.05 for all two-tailed statistical tests.
Results
Identification of DEGs and functional-enriched pathways
Normalization and differential gene analysis of mouse lymphedema RNA-seq data from PRJEB15150 were performed to screen for DEGs in lymphedema samples. The lymphedema RNA-seq data can be classified into three groups: control group (normal tail tissue, n=5), acute lymphedema group (2 weeks after lymph stasis, acute lymphedema, n=5), as well as chronic lymphedema group (6 weeks after lymph stasis, chronic lymphedema, n=4). There were 454 upregulated and 173 downregulated genes identified in the acute-control comparison, 482 and 22 in the chronic-control comparison with the threshold of |log2(FC)| >1.5 and adjusted P value <0.05. The results are visualized in Figure 2A,2B.
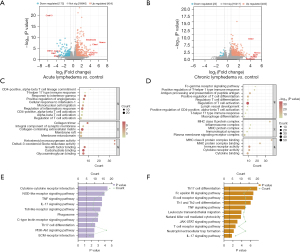
We then performed GO and KEGG analysis to elucidate the biological significance of DEGs. GO terms were further categorized into three aspects, namely BP, CC, and MF. In biological functions, GO results presented that activation and migration of T-helper-related functions were prominent in GO terms. Regulation of T cell activation, CD4-positive, Th17 type immune response, CD4-positive, alpha-beta T cell activation was significantly different both in the acute lymphedema group and chronic lymphedema group compared with the control group. Positive regulation of Th1 type immune response, regulatory T cell differentiation, and macrophage differentiation were significantly pronounced in chronic lymphedema (Figure 2C,2D). In the CC category, collagen-containing extracellular matrix, and integral component of the synaptic membrane were noticeable both in acute lymphedema and chronic lymphedema. The DEGs of chronic lymphedema were related to the MHC class II protein complex, MHC protein complex, and immunological synapse. In the MF category, the DEGs were primarily associated with growth factor binding, immune receptor activity, and MHC protein complex binding.
The KEGG analysis showed that T-helper cell-associated inflammation pathways were enriched in the lymphedema state. In the acute lymphedema group, the tumor necrosis factor (TNF) signaling pathway, IL-17 signaling pathway, Th17 cell differentiation, and PI3K-Akt signaling pathway were accumulated (Figure 2E); while the functional pathways of chronic lymphedema were Th17 cell differentiation, Th1, and Th2 cell differentiation, TNF signaling pathway, JAK-STAT signaling pathway, T cell receptor signaling pathway, IL-17 signaling pathway (Figure 2F).
Immune cell infiltration analysis
We applied the ImmuCellAI-mouse method based on ssGSEA to decode the relative infiltration abundance of 36 immune cell subpopulations in lymphedema groups (n=9) and normal controls (n=5), as illustrated in Figure 3. The relative infiltration abundance of follicular B cells (P<0.01), memory B cells (P=0.02), M1 macrophage (P=0.03), CD4+ Tm cells (P=0.02), and T-helper cells (P=0.019) while the decreased immune abundance of granulocytes (P=0.01), neutrophils (P=0.02), and plasma cells (P=0.01). Notably, the relative infiltration abundance of T cells (control group =0.0236, lymphedema group =0.0782, P=0.3), CD4+ T cells (control group =0.0005, lymphedema group =0.0154, P=0.2), and Tregs (control group =0.000, lymphedema group =0.0014, P=0.13) were higher in the lymphedema group than in the control group, but without statistical significance. These results implied that multiple immune cells were involved in the formation of tissue inflammation in lymphedema, particularly the immune cells involved in the activation of T-helpers.
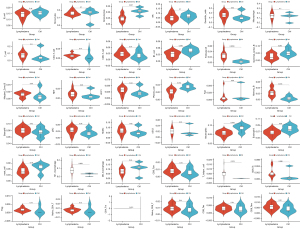
GSEA results of T-helper, macrophage, B cells, and gamma-delta T-associated functions
Since the immune infiltration results suggested that CD4+ T helpers, macrophages, and B cells were implicated in the progression of lymphedema and T cell receptor signaling was activated, the pathways associated with T-helpers, macrophage, B cells, and gamma-delta T cells were downloaded from the Molecular Signatures Database and the difference in the pathways between control group, acute group and the chronic group were compared by GSEA, as shown in Appendix 1.
The levels of Th1 immune response enrichment, type 2 immune response, Th17 cell differentiation, and Th17 immune response, as well as the regulation of T-helper cell differentiation, were all significantly higher for T-helpers in the acute group when compared to the control group, as well as in the chronic group when compared to the acute group and the control group. However, macrophage migration, macrophage chemotaxis, macrophage activation, and macrophage cytokine production were significantly up-regulated in both the chronic group and the acute group compared to the control group. It is interesting to note that no macrophage-associated pathways were significantly enriched in the acute group compared to the control group. In the acute group compared to the control group, in the chronic group compared to the control group, and in the chronic group compared to the acute group, B cell activation, differentiation, and proliferation were significantly higher. No gamma-delta pathways were more highly expressed in the chronic or acute groups compared to the control group by the present RNA-seq data.
Construction of T-helper-associated gene signature (THAGS)
The functional enrichment results showed that the differentiation, and activation of CD4+ T-associated pathways accounted prominently for the immunological alternations, mirroring the changes of immune cells in immune infiltration analysis and also testified in previous animal studies. With “T helper” as the keyword, we searched the MSigDB database and generated 31 relevant pathways containing 129 THAGs. By the intersection of THAGs and DEGs, we constructed THAGs in lymphedema, containing 18 genes listed in Table 1.
Table 1
Symbol | Ensembl | Entrezid |
---|---|---|
Batf | ENSMUSG00000034266 | 53314 |
Ccr2 | ENSMUSG00000049103 | 12772 |
Ccr6 | ENSMUSG00000040899 | 12458 |
Cd4 | ENSMUSG00000023274 | 12504 |
Cd74 | ENSMUSG00000024610 | 16149 |
Clec4n | ENSMUSG00000023349 | 56620 |
Foxp3 | ENSMUSG00000039521 | 20371 |
H2-Ab1 | ENSMUSG00000073421 | 14961 |
Il12rb1 | ENSMUSG00000000791 | 16161 |
Il18r1 | ENSMUSG00000026070 | 16182 |
Il1b | ENSMUSG00000027398 | 16176 |
Il27ra | ENSMUSG00000005465 | 50931 |
Irf4 | ENSMUSG00000021356 | 16364 |
Lilrb4a | ENSMUSG00000062593 | 14728 |
Rsad2 | ENSMUSG00000020641 | 58185 |
Slamf1 | ENSMUSG00000015316 | 27218 |
Slamf6 | ENSMUSG00000015314 | 30925 |
Spn | ENSMUSG00000051457 | 20737 |
THAHGs, T-helper-associated hub genes.
PPI network analysis and selection of THAHGs signature
By the combination of THAGs in the acute lymphedema group (n=9) and chronic lymphedema group (n=16), we formed the list of THAGs with 18 genes (Figure 4A). The String database calculated that the PPI networks included 18 genes/nodes with 56 edges, which are visualized in Figure 4B. The most core PPI module was selected by MCODE, which contains 8 nodes and 21 edges, as shown in Figure 4C. Therefore, the THAHGs included in total eight THAGs, namely Cd4, Foxp3, Irf4, Ccr6, Il12rb1, Batf, Il1b, and Cd74 (Figure 4C,4D).
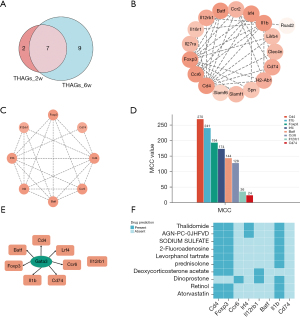
TF prediction
Eight hub genes correlated with lymphedema were tested for TF binding motifs using the iRegulon plugin. The results indicated that seven genes, excluding Il12rb1, were regulated by Gata3 (Figure 4E).
Potential drug prediction
We used the DSigDB database to predict potential target drugs associated with key genes that may be useful for treating lymphedema by modulating T-helper cell immunity. The results indicated that thalidomide might be the potent drug for relieving lymphedema by targeting Cd4, Foxp3, Irf4 and Il1b (P<0.01, combined score >5,000, Figure 4F). The top 10 drugs were listed in Table 2.
Table 2
Term | Adjusted P value | Odds ratio | Combined score | Genes |
---|---|---|---|---|
Thalidomide BOSS | 7.01E−06 | 316.3333 | 5,898.919843 | CD4; IRF4; IL1B; FOXP3 |
AGN-PC-0JHFVD BOSS | 2.41E−04 | 105.9091 | 1,526.975503 | CD4; IRF4; IL1B; FOXP3 |
SODIUM SULFATE BOSS | 5.32E−04 | 192.871 | 2,549.807655 | CD4; IL1B; FOXP3 |
2-Fluoroadenosine BOSS | 7.20E−04 | 157.2316 | 1,985.731623 | CD4; IL1B; FOXP3 |
Levorphanol tartrate BOSS | 9.20E−04 | 132.68 | 1,610.295217 | CD4; IL1B; FOXP3 |
Prednisolone BOSS | 9.20E−04 | 125.6653 | 1,505.324472 | CD4; IL1B; FOXP3 |
Deoxycorticosterone acetate BOSS | 0.001451 | 101.9231 | 1,158.834927 | CD4; IRF4; FOXP3 |
Dinoprostone CTD 00007049 | 0.001505 | 96.13548 | 1,076.672586 | IL1B; CCR6; IL12RB1 |
Retinol BOSS | 0.001607 | 90.27273 | 994.4765346 | CD4; IL1B; FOXP3 |
Atorvastatin BOSS | 0.001622 | 85.08 | 922.5983899 | CD4; IL1B; FOXP3 |
Correlation analysis of THAHGs
Correlation analysis between eight THAHGs indicated that Batf, Ccr6, Cd4, Il12rb1, Foxp3, and Cd74 were positively associated, while these six genes were negatively associated with Il1b and Irf4 (Figure 5). Pearson correlation between THAHGS and immune abundance in lymphedema was further calculated. Batf, Ccr6, Cd4, Il12rb1, Foxp3, and Cd74 were negatively related to granulocytes, neutrophils, and plasma cells; besides, they positively correlated with CD4+ T cells, M1 macrophages, CD4+ Tm, T-helpers, Tregs (Figure 6A-6F). Il1b and Irf4 were negatively related to CD4+ T cells, memory CD4+ T cells, follicular B cells, M1 macrophages, memory B cells, Naïve CD4+ T cells, T-helper cells, and Tregs, all of which were up-regulated immune cells in lymphedema (Figure 6G,6H). This suggested that Il1b and Irf4 were negatively related to CD4+ T-type immune alternations in lymphedema. Such correlation showcased the changes of Batf, Ccr6, Cd4, Il12rb1, Foxp3, and Cd74 mirrored the alternation of immune cell populations in lymphedema.
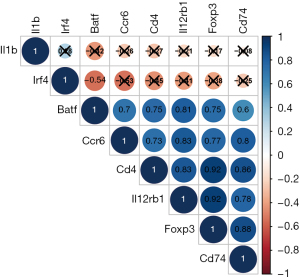
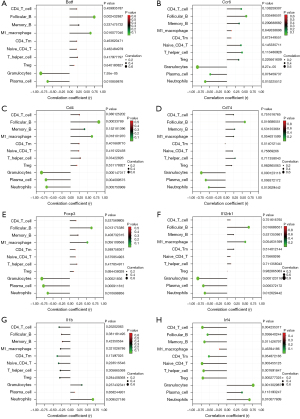
ROC curve analysis
The AUCs of Cd4, Foxp3, Ccr6, Irf4, Il12rb1, Batf, Il1b, and Cd74 for lymphedema diagnosis were 0.911, 0.844, 0.844, 0.756, 0.956, 0.867, 0.822, 0.711, respectively (Figure 7). The AUC value of THAHGs was larger than 0.70, which indicated that THAHGs were potential biomarkers for molecular diagnosis in lymphedema. The AUC value of Il12rb1 was the highest (AUC =0.956), followed by Cd4 (AUC =0.911).
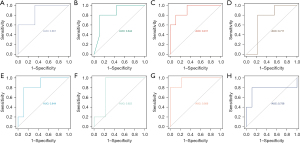
Discussion
Mounting literature has accumulated that CD4+ T helpers are involved in fibrosis, lymphangiogenesis, and lymphatic dysfunction, which play an essential role in the pathological progression of secondary lymphedema (12,34). CD4+ naïve cells were activated in the local lymph nodes (11), where they encountered antigen-presenting cells and started differentiation into Th1 (35), Th2 (36), Th17 (37), and Tregs (14) to the lymphatic limbs. CD4+ T cells are necessary for lymphatic dysfunction, and tissue fibrosis in lymphedema; thus, we could infer that the disturbed balance of Th1/Th2 and Th17/Treg participates in the progression of lymphedema (38). Therefore, bioinformatics exploration of CD4+ THAGs expression profiles and identification of key genes associated with T helpers are particularly beneficial to advances in molecular diagnosis and therapy for lymphedema.
The functional enrichment analysis and immune infiltration showed that T-helper-related immunological microenvironments were highly active in lymphedema progress. GO-BP results showed that DEGs of acute lymphedema were mostly enriched in CD4+, alpha-beta T cell lineage commitment and activation, Th17 type immune response, and response to interferon-gamma (IFN-gamma) while the DEGs of lymphedema-6w were closely related to positive regulation of type I immune response, positive regulation of T cell differentiation, regulation of T cell differentiation, Th17 type immune response, and macrophage differentiation. The KEGG pathways of DEGs of the acute lymphedema group were cytokine-cytokine receptor interaction, TNF signaling pathway, IL-17 signaling pathway, and Th17 cell differentiation, suggesting the inflammation process and activation of Th17 immune response. Besides, the KEGG pathway of the chronic lymphedema group demonstrated that Th17 cell differentiation, Th1, and Th2 cell differentiation, leukocyte trans-endothelial migration, and T cell receptor signaling pathway were enriched in chronic lymphedema. The immune infiltration of lymphedema data also showed there was a significantly higher infiltration abundance of follicular B cells, M1 macrophage, CD4+ Tm cells, and T-helpers; besides CD4+ T cells, and Tregs were of higher infiltration abundance in lymphedema group, which were parallel to the enrichment analysis. The above bioinformatics consequences indicated the special T-helper-related immune response in lymphedema. Th17 immune response was activated in 2 weeks after lymph stasis, which was also proved in Ogata’s study (35). Interestingly, the present results showed that Th17 cell and IL-17 pathway were activated throughout the 2 and 6 weeks after lymph obstruction, suggesting the crucial roles of Th17 cells both in the acute and chronic phase of lymphedema. Th17 secreted pro-inflammatory cytokines and inhibited the tube formation of lymphatic endothelial cells in vitro (20), suggesting that Th17 inhibited lymphangiogenesis and promoted inflammation in lymphedema. The mix of Th1 and Th2 type immune response was noticed also in Mehrara’s animal study and the inhibition of IL-4/IL-13 could relieve edema, fibrosis, and lymphatic function (18). Therefore, the present bioinformatics results further proved that CD4+ T-helpers are significant regulators in secondary lymphedema.
Except for CD4+ T helpers, the GSEA results also indicated that macrophages and B cells participated in the pathological changes of mouse tail lymphedema. Macrophages have been reported to be involved in the development of lymphedema in previous studies (39-41). In the hindlimb model of rats’ secondary lymphedema, macrophages gathered around the adipocytes in the enlarged lymphedema tissue (40). The immune infiltration analysis suggested that the relative immune abundance of M1 pro-inflammatory was higher in the lymphedema tissue. In line with this, M1 macrophages produce inducible nitric oxide synthase (iNOS) to attenuate lymphatic vessel pumping in overweight mice (42). It is noteworthy that in the GSEA results, macrophage-associated biological pathways were not significantly enriched in the acute phase of lymphedema but significantly expressed in the chronic phase of the lymphedema, suggesting that macrophages especially M1 macrophages, were involved in aggravating chronic lymphedema. Moreover, the gamma-delta cells and B cells have not been evaluated in the state of lymphedema for now in the scientific world of lymphedema. The GSEA results showed that B cell pathways were enriched both in the acute and chronic lymphedema but gamma-delta T cells were not implicated in the present analysis but needed to test in the future.
Using PPI analysis, eight key genes including Cd4, Foxp3, Irf4, Ccr6, Il12rb1, Batf, Il1b, and Cd74 were identified as THAHGs in secondary lymphedema. According to the PPI network and correlation analysis, these eight genes formed an interaction network with 8 nodes and 21 edges and were associated with immune cell infiltration. Cd4 was the up-regulated gene in lymphedema compared with the control, suggesting the activation of CD4+ T cells. CD4 was the cellular marker of T helpers. The number of CD4+ T cells was augmented in lymphedema, which was well testified in a variety of in vivo studies (11,12,16,43). Foxp3, encoding the TF of Tregs, was also up-regulated in the acute lymphedema group and the chronic lymphedema group in comparison with the control group, which was parallel to the immune infiltration results. The activation of Tregs might partly counterbalance the immunological disturbance caused by other pro-inflammation CD4+ T-helpers because transplantation of Tregs could improve the lymph vessel function (14). Irf4 is also a TF participating in the differentiation of Th17 and Tregs (44,45); Batf, encoding a nuclear basic leucine zipper protein, belongs to the AP-1 family TF that controls, particularly, the differentiation of Th17 (46,47). Batf regulates IL-17 expression (48) and several other genes expressed by Th17 cells (49). Ccr6 encodes the receptor of CCL20 and the binding of CCL20 and CCR6 protein is responsible for the chemotaxis of dendritic cells, and the recruitment of Th17 as well as the Tregs to inflammatory sites (50). IL-12-receptor β1, encoded by Il12rb1, functions as the IL receptor binding to IL-12/IL-23. IL-12 and IL-23 pathways are generally accepted as pro-inflammatory cytokines (51) and are related to the response Th1 and Th17. Interestingly, the IL-12 pathway was also upregulated in podoconiosis, volcanic-soil-induced leg lymphedema (52). Cd74 plays an essential role in MHC class II antigen processing, suggesting the activation of antigen-presenting cells (APCs). Il1B protein, produced by macrophages, supports Th17 differentiation and cooperates with IL-12 mediating the Th1 cells to IFN-gamma synthesis (53). To sum it up, the THAHGs stand for the participation of APCs, macrophages, Th1, Th2, Th17, and Tregs in lymphedema.
The diagnostic values of THAHGs were tested by ROC curves and the results showed that all THAHGs harbored a good diagnostic value. The timely diagnosis of peripheral lymphedema is of clinical significance because most breast cancer-related lymphedema cases happen several years after axillary lymph node dissection and radiotherapy. Notably, the immune dysfunction was not confined to the lymphedematous region but also influenced the whole body. The CD4+ cells were also increased in the contralateral limbs (43). Such results provide clues that biomarkers associated with the activation of T-helpers may serve as diagnostic molecules in the early stages of lymphedema.
With the iRegulon plugin in Cytoscape, Gata3 was the most potential TF (Figure 4E) in the lymphedema state by targeting THAHGs. In the present analysis, Gata3 was stable in the lymphedema groups compared with the control group. However, Gata3 was associated with the regulation of seven hub genes in lymphedema, namely, Cd4, Foxp3, Irf4, Ccr6, Batf, Il1b, and Cd74. As a TF, Gata3 is essential for CD4+ T cell differentiation, homeostasis, activation, proliferation, and effector functions (54). Gata3 promotes the differentiation of Th2 but inhibited the differentiation of Th1 cells; Gata3 also functions in the balance of Th17/Treg because Gata3 supported the Treg differentiation while suppressing the Th17 differentiation via binding to Il17 and Rorc loci (55,56). From mouse lymphedema experiments, Th1, Th2, Th17, and Tregs were all up-regulated in the lymphedema state, which could explain the relatively stable level of Gata3. The nuanced regulation details of Gata3 in lymphedema state requires further demonstration.
The biological pathways of the Th1 and Th2 response were evaluated further using the GSEA method. The results showed that multiple pathways associated with Th1 cell and type 2 immune response (production of IL-4, IL-10 mostly by Th2 cells) were significant both in the acute and chronic phase compared with the control group. Besides, Th1 and Th2 pathways were all activated and strengthened throughout the process of lymphedema as the enrichment levels of Th1, and Th2 pathways were higher in the chronic group compared with the acute group. Therefore, it would be of benefit to curb the Th1, Th2, or Th17 or all pathways to slow the progression of lymphedema. Several medications have been proposed and investigated to target the T-helpers in line with the RNA-seq results. Firstly, tacrolimus binds to FK-506-binding protein 12 in CD4+ T helper cells, and the resulting complex prevents calcineurin’s phosphatase activity, thus decreasing the production of IL-2 (57). Tacrolimus prevents adequate IL-2 signaling, which is necessary for effector CD4+ T cells to avoid apoptosis and maintain persistence as memory cells. As a result, CD4+ T cells cannot survive in the presence of tacrolimus (58). In a mouse tail model of lymphedema, local administration of tacrolimus reduced tissue thickness curbed the inflammatory cytokine production, and thus showed promise in treating lymphedema (59). In a single-arm clinical trial, tacrolimus significantly lowered the arm volume and improved the health-related quality scores of eighteen BCRL patients (60). The verification of tacrolimus further highlights that preventing the activation of Th1 as well as Th2 response is useful in treating lymphedema. Besides, neutralizing antibodies have been applied for treating lymphedema both in animal models (16) and BCRL patients (61). However, neutralizing antibodies targeting the Th2 immune response did not reduce the limb volume for BCRL patients, suggesting down-regulating the function of Th2 cells alone was not enough to improve all symptoms of lymphedema. The most prominent drug in the lymphedema state was thalidomide according to DSigDB with a combined score of 5,899. Thalidomide has many immunomodulatory properties and has been applied to treat pediatric Crohn’s disease (62). GSEA results indicated that pathways of Th1, Th2, and Th17 were all enriched throughout the development of lymphedema and immune cell abundance results suggested that there were higher immune abundance levels of CD4+ Tm cells and lower immune abundance levels of Tregs in the lymphedema state. In line with the RNA-data analysis, thalidomide modulated the Th1 response and regulated the Th17/Treg balance. It inhibited the Th1 response via the inhibition of TNF, IFN-c, and IL-12 (63,64). Recent studies showed that thalidomide could relieve skin fibrosis in a mouse model of systemic sclerosis by inhibiting transforming growth factor beta1 (TGF-β1)/Smad3 signaling and regulating the Th17/Treg balance (65,66). Others also reported that thalidomide reduced CD4+ T helper cell infiltration in skin inflammation and Th1- and Th17-associated genes (67). Since upregulation of Th1 and Th17 response and distortion of Th17/Treg balance was also notable in the lymphedema state, thalidomide might provide some relief for lymphedema patients. Of note, the tenth potential drug was atorvastatin. Statins, a type of HMG-CoA reductase inhibitor, have been found to regulate the balance of Th17 and Treg by downregulating MHC-II expression of APCs (68), curbing the function of ICAM-1 (69), as well as preventing Ras and Rho signaling (70). Thus, stains show promise in Th17-induced diseases such as multiple sclerosis and rheumatoid arthritis (71). These above results indicate that immunomodulatory drugs targeting the balance of T-helper immune response might benefit secondary lymphedema.
The present study was not without limitations. For one thing, data and results generated in the study were from bioinformatics calculations of public datasets. Secondly, RNA-seq data of lymphedema verifying these results were inadequate. Surprisingly, previous findings of animal and humane basic research make the present results plausible. However, this is the first study by our knowledge to construct a THAHGs signature for lymphedema, which is significantly related to alternations of immune response in lymphedema. Moreover, it is the first time that TFs and immunoregulatory drugs have been predicted. Such results update the knowledge of T helper cells in lymphedema and provide clues for future lymphedema research.
Conclusions
Biological pathways associated with T-helpers were significantly enriched in mouse lymphedema tissue by bioinformatics analysis. The relative immune infiltration abundance of M1 macrophage, CD4+ Tm cells, and T-helper cells was higher in the lymphedema group. Besides, we identified a novel list of THAHGs containing eight genes, namely, Cd4, Foxp3, Irf4, Ccr6, Il12rb1, Batf, Il1b, and Cd74. The THAHGs were closely correlated with immune infiltration results and with good diagnostic properties.
Acknowledgments
Funding: This work was supported by
Footnote
Reporting Checklist: The authors have completed the STREGA reporting checklist. Available at https://gs.amegroups.com/article/view/10.21037/gs-23-48/rc
Peer Review File: Available at https://gs.amegroups.com/article/view/10.21037/gs-23-48/prf
Conflicts of Interest: Both authors have completed the ICMJE uniform disclosure form (available at https://gs.amegroups.com/article/view/10.21037/gs-23-48/coif). The authors have no conflicts of interest to declare.
Ethical Statement: The authors are accountable for all aspects of the work in ensuring that questions related to the accuracy or integrity of any part of the work are appropriately investigated and resolved.
Open Access Statement: This is an Open Access article distributed in accordance with the Creative Commons Attribution-NonCommercial-NoDerivs 4.0 International License (CC BY-NC-ND 4.0), which permits the non-commercial replication and distribution of the article with the strict proviso that no changes or edits are made and the original work is properly cited (including links to both the formal publication through the relevant DOI and the license). See: https://creativecommons.org/licenses/by-nc-nd/4.0/.
References
- Rockson SG. Lymphedema. Am J Med 2001;110:288-95.
- DiSipio T, Rye S, Newman B, et al. Incidence of unilateral arm lymphoedema after breast cancer: a systematic review and meta-analysis. Lancet Oncol 2013;14:500-15. [Crossref] [PubMed]
- Cormier JN, Askew RL, Mungovan KS, et al. Lymphedema beyond breast cancer: a systematic review and meta-analysis of cancer-related secondary lymphedema. Cancer 2010;116:5138-49. [Crossref] [PubMed]
- Sanal-Toprak C, Ozsoy-Unubol T, Bahar-Ozdemir Y, et al. The efficacy of intermittent pneumatic compression as a substitute for manual lymphatic drainage in complete decongestive therapy in the treatment of breast cancer related lymphedema. Lymphology 2019;52:82-91.
- Chang DW, Suami H, Skoracki R. A prospective analysis of 100 consecutive lymphovenous bypass cases for treatment of extremity lymphedema. Plast Reconstr Surg 2013;132:1305-14. [Crossref] [PubMed]
- Aschen SZ, Farias-Eisner G, Cuzzone DA, et al. Lymph node transplantation results in spontaneous lymphatic reconnection and restoration of lymphatic flow. Plast Reconstr Surg 2014;133:301-10. [Crossref] [PubMed]
- Cuadrado GA, de Andrade MFC, Ariga SK, et al. Inflammation Precedes Fat Deposition in an Experimental Model of Lymphedema. Lymphat Res Biol 2021;19:116-25. [Crossref] [PubMed]
- Zampell JC, Aschen S, Weitman ES, et al. Regulation of adipogenesis by lymphatic fluid stasis: part I. Adipogenesis, fibrosis, and inflammation. Plast Reconstr Surg 2012;129:825-34. [Crossref] [PubMed]
- Avraham T, Clavin NW, Daluvoy SV, et al. Fibrosis is a key inhibitor of lymphatic regeneration. Plast Reconstr Surg 2009;124:438-50. [Crossref] [PubMed]
- Markhus CE, Karlsen TV, Wagner M, et al. Increased interstitial protein because of impaired lymph drainage does not induce fibrosis and inflammation in lymphedema. Arterioscler Thromb Vasc Biol 2013;33:266-74. [Crossref] [PubMed]
- García Nores GD, Ly CL, Cuzzone DA, et al. CD4(+) T cells are activated in regional lymph nodes and migrate to skin to initiate lymphedema. Nat Commun 2018;9:1970. [Crossref] [PubMed]
- Zampell JC, Yan A, Elhadad S, et al. CD4(+) cells regulate fibrosis and lymphangiogenesis in response to lymphatic fluid stasis. PLoS One 2012;7:e49940. [Crossref] [PubMed]
- Ly CL, Cuzzone DA, Kataru RP, et al. Small Numbers of CD4+ T Cells Can Induce Development of Lymphedema. Plast Reconstr Surg 2019;143:518e-26e. [Crossref] [PubMed]
- Gousopoulos E, Proulx ST, Bachmann SB, et al. Regulatory T cell transfer ameliorates lymphedema and promotes lymphatic vessel function. JCI Insight 2016;1:e89081. [Crossref] [PubMed]
- Saravia J, Chapman NM, Chi H. Helper T cell differentiation. Cell Mol Immunol 2019;16:634-43. [Crossref] [PubMed]
- Avraham T, Zampell JC, Yan A, et al. Th2 differentiation is necessary for soft tissue fibrosis and lymphatic dysfunction resulting from lymphedema. FASEB J 2013;27:1114-26. [Crossref] [PubMed]
- Savetsky IL, Ghanta S, Gardenier JC, et al. Th2 cytokines inhibit lymphangiogenesis. PLoS One 2015;10:e0126908. [Crossref] [PubMed]
- Ly CL, Nores GDG, Kataru RP, et al. T helper 2 differentiation is necessary for development of lymphedema. Transl Res 2019;206:57-70. [Crossref] [PubMed]
- Cho S, Roh K, Park J, et al. Hydrolysis of Hyaluronic Acid in Lymphedematous Tissue Alleviates Fibrogenesis via T(H)1 Cell-Mediated Cytokine Expression. Sci Rep 2017;7:35. [Crossref] [PubMed]
- Park HJ, Yuk CM, Shin K, et al. Interleukin-17A negatively regulates lymphangiogenesis in T helper 17 cell-mediated inflammation. Mucosal Immunol 2018;11:590-600. [Crossref] [PubMed]
- Gousopoulos E, Proulx ST, Scholl J, et al. Prominent Lymphatic Vessel Hyperplasia with Progressive Dysfunction and Distinct Immune Cell Infiltration in Lymphedema. Am J Pathol 2016;186:2193-203. [Crossref] [PubMed]
- Love MI, Huber W, Anders S. Moderated estimation of fold change and dispersion for RNA-seq data with DESeq2. Genome Biol 2014;15:550. [Crossref] [PubMed]
- Wu T, Hu E, Xu S, et al. clusterProfiler 4.0: A universal enrichment tool for interpreting omics data. Innovation (Camb) 2021;2:100141. [Crossref] [PubMed]
- Shannon P, Markiel A, Ozier O, et al. Cytoscape: a software environment for integrated models of biomolecular interaction networks. Genome Res 2003;13:2498-504. [Crossref] [PubMed]
- Bader GD, Hogue CW. An automated method for finding molecular complexes in large protein interaction networks. BMC Bioinformatics 2003;4:2. [Crossref] [PubMed]
- Miao YR, Xia M, Luo M, et al. ImmuCellAI-mouse: a tool for comprehensive prediction of mouse immune cell abundance and immune microenvironment depiction. Bioinformatics 2022;38:785-91. [Crossref] [PubMed]
- Liberzon A, Subramanian A, Pinchback R, et al. Molecular signatures database (MSigDB) 3.0. Bioinformatics 2011;27:1739-40. [Crossref] [PubMed]
- Liberzon A, Birger C, Thorvaldsdóttir H, et al. The Molecular Signatures Database (MSigDB) hallmark gene set collection. Cell Syst 2015;1:417-25. [Crossref] [PubMed]
- Subramanian A, Tamayo P, Mootha VK, et al. Gene set enrichment analysis: a knowledge-based approach for interpreting genome-wide expression profiles. Proc Natl Acad Sci U S A 2005;102:15545-50. [Crossref] [PubMed]
- Mootha VK, Lindgren CM, Eriksson KF, et al. PGC-1alpha-responsive genes involved in oxidative phosphorylation are coordinately downregulated in human diabetes. Nat Genet 2003;34:267-73. [Crossref] [PubMed]
- Patil I. Visualizations with statistical details: The 'ggstatsplot' approach. Journal of Open Source Software 2021;6.
- Robin X, Turck N, Hainard A, et al. pROC: an open-source package for R and S+ to analyze and compare ROC curves. BMC Bioinformatics 2011;12:77. [Crossref] [PubMed]
- Janky R, Verfaillie A, Imrichová H, et al. iRegulon: from a gene list to a gene regulatory network using large motif and track collections. PLoS Comput Biol 2014;10:e1003731. [Crossref] [PubMed]
- Li CY, Kataru RP, Mehrara BJ. Histopathologic Features of Lymphedema: A Molecular Review. Int J Mol Sci 2020;21:2546. [Crossref] [PubMed]
- Ogata F, Fujiu K, Matsumoto S, et al. Excess Lymphangiogenesis Cooperatively Induced by Macrophages and CD4(+) T Cells Drives the Pathogenesis of Lymphedema. J Invest Dermatol 2016;136:706-14. [Crossref] [PubMed]
- Shin K, Kataru RP, Park HJ, et al. TH2 cells and their cytokines regulate formation and function of lymphatic vessels. Nat Commun 2015;6:6196. [Crossref] [PubMed]
- Wang Q, Yang Y, Wu F, et al. Predominant T-helper 17 skewing in elephantiasis nostras verrucosa. J Dermatol 2019;46:e288-90.
- Brown S, Dayan JH, Kataru RP, et al. The Vicious Circle of Stasis, Inflammation, and Fibrosis in Lymphedema. Plast Reconstr Surg 2023;151:330e-41e. [Crossref] [PubMed]
- Liu X, Yuan M, Xiang Q, et al. Single-cell RNA sequencing of subcutaneous adipose tissues identifies therapeutic targets for cancer-associated lymphedema. Cell Discov 2022;8:58. [Crossref] [PubMed]
- Sano M, Hirakawa S, Sasaki T, et al. Role of Subcutaneous Adipose Tissues in the Pathophysiology of Secondary Lymphedema. Lymphat Res Biol 2022;20:593-9. [Crossref] [PubMed]
- Liu Z, Li J, Bian Y, et al. Low-intensity pulsed ultrasound reduces lymphedema by regulating macrophage polarization and enhancing microcirculation. Front Bioeng Biotechnol 2023;11:1173169. [Crossref] [PubMed]
- Torrisi JS, Hespe GE, Cuzzone DA, et al. Inhibition of Inflammation and iNOS Improves Lymphatic Function in Obesity. Sci Rep 2016;6:19817. [Crossref] [PubMed]
- Wolf S, von Atzigen J, Kaiser B, et al. Is Lymphedema a Systemic Disease? A Paired Molecular and Histological Analysis of the Affected and Unaffected Tissue in Lymphedema Patients. Biomolecules 2022;12:1667. [Crossref] [PubMed]
- Xiao F, Rui K, Han M, et al. Artesunate suppresses Th17 response via inhibiting IRF4-mediated glycolysis and ameliorates Sjog̈ren's syndrome. Signal Transduct Target Ther 2022;7:274. [Crossref] [PubMed]
- Yu W, Ji N, Gu C, et al. IRF4 is Correlated with the Conversion to a Th17-Like Phenotype in Regulatory T Cells from the Malignant Pleural Effusion. Int J Gen Med 2021;14:6009-19. [Crossref] [PubMed]
- Hasan Z, Koizumi SI, Sasaki D, et al. JunB is essential for IL-23-dependent pathogenicity of Th17 cells. Nat Commun 2017;8:15628. [Crossref] [PubMed]
- Carr TM, Wheaton JD, Houtz GM, et al. JunB promotes Th17 cell identity and restrains alternative CD4(+) T-cell programs during inflammation. Nat Commun 2017;8:301. [Crossref] [PubMed]
- Ciofani M, Madar A, Galan C, et al. A validated regulatory network for Th17 cell specification. Cell 2012;151:289-303. [Crossref] [PubMed]
- Shaulian E. AP-1--The Jun proteins: Oncogenes or tumor suppressors in disguise? Cell Signal 2010;22:894-9.
- Röhrl J, Yang D, Oppenheim JJ, et al. Specific binding and chemotactic activity of mBD4 and its functional orthologue hBD2 to CCR6-expressing cells. J Biol Chem 2010;285:7028-34. [Crossref] [PubMed]
- Ullrich KA, Schulze LL, Paap EM, et al. Immunology of IL-12: An update on functional activities and implications for disease. EXCLI J 2020;19:1563-89. [Crossref] [PubMed]
- Alcantara DR, Jones CI, Altmann DM, et al. Multiplexed gene expression analysis of HLA class II-associated podoconiosis implicates chronic immune activation in its pathogenesis. Trans R Soc Trop Med Hyg 2020;114:926-36. [Crossref] [PubMed]
- Tominaga K, Yoshimoto T, Torigoe K, et al. IL-12 synergizes with IL-18 or IL-1beta for IFN-gamma production from human T cells. Int Immunol 2000;12:151-60. [Crossref] [PubMed]
- Wan YY. GATA3: a master of many trades in immune regulation. Trends Immunol 2014;35:233-42.
- Wohlfert EA, Grainger JR, Bouladoux N, et al. GATA3 controls Foxp3+ regulatory T cell fate during inflammation in mice. J Clin Invest 2011;121:4503-15. [Crossref] [PubMed]
- Wang Y, Su MA, Wan YY. An essential role of the transcription factor GATA-3 for the function of regulatory T cells. Immunity 2011;35:337-48. [Crossref] [PubMed]
- Jørgensen KA, Koefoed-Nielsen PB, Karamperis N. Calcineurin phosphatase activity and immunosuppression. A review on the role of calcineurin phosphatase activity and the immunosuppressive effect of cyclosporin A and tacrolimus. Scand J Immunol 2003;57:93-8. [Crossref] [PubMed]
- McKinstry KK, Strutt TM, Bautista B, et al. Effector CD4 T-cell transition to memory requires late cognate interactions that induce autocrine IL-2. Nat Commun 2014;5:5377. [Crossref] [PubMed]
- Gardenier JC, Kataru RP, Hespe GE, et al. Topical tacrolimus for the treatment of secondary lymphedema. Nat Commun 2017;8:14345. [Crossref] [PubMed]
- Gulmark Hansen FC, Jørgensen MG, Sørensen JA. Treatment of Breast Cancer-Related Lymphedema With Topical Tacrolimus: A Prospective, Open-Label, Single-Arm, Phase II Pilot Trial. J Breast Cancer 2023;26:46-59. [Crossref] [PubMed]
- Mehrara BJ, Park HJ, Kataru RP, et al. Pilot Study of Anti-Th2 Immunotherapy for the Treatment of Breast Cancer-Related Upper Extremity Lymphedema. Biology (Basel) 2021;10:934. [Crossref] [PubMed]
- Yang C, Singh P, Singh H, et al. Systematic review: thalidomide and thalidomide analogues for treatment of inflammatory bowel disease. Aliment Pharmacol Ther 2015;41:1079-93. [Crossref] [PubMed]
- Moller DR, Wysocka M, Greenlee BM, et al. Inhibition of IL-12 production by thalidomide. J Immunol 1997;159:5157-61.
- McHugh SM, Rifkin IR, Deighton J, et al. The immunosuppressive drug thalidomide induces T helper cell type 2 (Th2) and concomitantly inhibits Th1 cytokine production in mitogen- and antigen-stimulated human peripheral blood mononuclear cell cultures. Clin Exp Immunol 1995;99:160-7. [Crossref] [PubMed]
- Lu Y, Zhao C, Lei L, et al. Effects of thalidomide on Th17, Treg cells and TGF-β1/Smad3 pathway in a mouse model of systemic sclerosis. Int J Rheum Dis 2020;23:406-19. [Crossref] [PubMed]
- Lei L, Zhao C, Qin F, et al. Th17 cells and IL-17 promote the skin and lung inflammation and fibrosis process in a bleomycin-induced murine model of systemic sclerosis. Clin Exp Rheumatol 2016;34:14-22.
- Chen M, Xie H, Chen Z, et al. Thalidomide ameliorates rosacea-like skin inflammation and suppresses NF-κB activation in keratinocytes. Biomed Pharmacother 2019;116:109011. [Crossref] [PubMed]
- Kwak B, Mulhaupt F, Myit S, et al. Statins as a newly recognized type of immunomodulator. Nat Med 2000;6:1399-402. [Crossref] [PubMed]
- Weitz-Schmidt G, Welzenbach K, Brinkmann V, et al. Statins selectively inhibit leukocyte function antigen-1 by binding to a novel regulatory integrin site. Nat Med 2001;7:687-92. [Crossref] [PubMed]
- Greenwood J, Walters CE, Pryce G, et al. Lovastatin inhibits brain endothelial cell Rho-mediated lymphocyte migration and attenuates experimental autoimmune encephalomyelitis. FASEB J 2003;17:905-7. [Crossref] [PubMed]
- Ulivieri C, Baldari CT. Statins: from cholesterol-lowering drugs to novel immunomodulators for the treatment of Th17-mediated autoimmune diseases. Pharmacol Res 2014;88:41-52. [Crossref] [PubMed]