Transforming breast reconstruction: the pioneering role of artificial intelligence in preoperative planning
Autologous breast reconstruction surgery has become an integral part of recovery for patients with breast cancer, providing them with the opportunity to regain physical characteristics that were lost during their oncological procedure (1). Multiple reconstructive options exist, such as the transverse rectus abdominis musculocutaneous (TRAM) flap and the deep inferior epigastric artery perforator (DIEP) flap. The DIEP flap is often the preferred choice due to its ability to closely mimic the natural appearance and texture of the native breast tissue (2). However, successful execution of the DIEP flap often presents challenges for surgeons, due to the unpredictable nature of the vascular anatomy associated with the deep inferior epigastric artery (DIEA), which can vary in location, size, and number of perforators (3,4). As a countermeasure, surgeons often use preoperative radiographic imaging, such as computed tomography angiography (CTA) to better understand the vascular architecture prior to surgery which helps in selecting appropriate perforators and reducing operative times (4-10).
Despite its usefulness, the conventional reporting of CTA scans is a labour-intensive process that can often be challenging and requires specific expertise (9,11). Manual identification of each perforating branch of the DIEA results in inherent subjectivity in accurately determining its size and location in relation to the umbilicus, creating inter-reporter variability. In addition to preoperative imaging, over time, surgeons have explored various other adjuncts to help with surgical planning and efficiency including the use of three-dimensional (3D) reconstructions/models and virtual/augmented reality projections (Figure 1) (1,9,12). More recently, the advent of artificial intelligence (AI) in the field of medical imaging has exhibited impressive results in improving diagnostic accuracy, reporting speed, and prediction of clinical outcomes (13-15). With respect to the preoperative planning of free flap surgery, AI undoubtedly has the potential to make considerable advancements to current techniques resulting in potentially improved accuracy, reliability, and efficiency. Yet, whilst the potential benefits are attractive, there remain certain risks that must also be considered. This study delves into the role of AI in preoperative planning of breast reconstruction. The study was conducted in accordance with the Declaration of Helsinki (as revised in 2013). No institutional ethics of this report was required. Full informed consent was obtained from the patient whose abdominal CTA reconstruction was used in Figure 1 of this article.
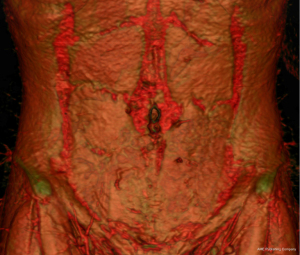
AI is a multifaceted field of computer science focused on creating systems that perform tasks requiring human intelligence, including pattern recognition, decision-making, and problem-solving. A subset of AI, machine learning (ML), deep learning (DL), and radiomics, can extract quantitative data from radiological images, hold significant potential in addressing existing challenges in radiology (16,17). These technologies can enhance CTA through improved data acquisition, image post-processing, and interpretation. Currently, CTA coupled with computer-generated 3D segmentation is the ‘gold standard’ for identifying DIEA perforators (9). The selection of optimal perforators is crucial, given that they serve as the exclusive blood supply to the flap. Implementing AI could expedite operation times and enhance reproducibility, reducing the time-consuming human input required in perforator selection. Incorporating AI into preoperative mapping of perforators through CTA could result in substantial advancements. These algorithms learn from large datasets and develop mathematical models that can make predictions or classifications based on new input data. ML systems can be trained to detect and classify relevant features in images, such as anatomical landmarks, or pertinent lesions apparent on the scan (16-18). The models analyse image features such as texture, shape, or intensity patterns using statistical techniques to identify patterns indicative of specific anatomy or conditions. DL, a subset of ML utilizes artificial neural networks mirroring the human brain’s structure and function (14,19). DL algorithms automatically learn hierarchical representations from raw data through an iterative optimization involving multiple layers of interconnected neurons. Initially, the model generates predictions on training images using random parameters. These predictions are compared to the known true labels of the images, and the parameters of the model are adjusted based on the differences identified by the algorithm. This process is repeated over multiple iterations, allowing the model to learn and refine its internal representations to better match the training data. Once trained, the model can be deployed to analyse new, unseen medical images. Furthermore, AI models have the potential to offer advanced perforator selection that could allow for enhanced predictive capabilities when planning operations. With the inclusion of perioperative and postoperative surgical data (e.g., complication rates, operative times, flap failure rates) into the AI models, there is the possibility for the systems to learn to identify which perforators (based on characteristics such as size, shape or location) would lead to reduced operative times, improved operative success and a lower rate of postoperative complications such as failure or infection. This has already been seen to be done by AI models basing predictions for flap failure and postoperative outcomes on patient characteristics (20,21). In the context of preoperative planning of breast reconstruction, the development of this technology will be dependent on the available and quantity/quality of training data, as opposed to the quality of technical programming. Nevertheless, while this is an exciting prospect, it remains the case that operative success or failure remains largely dictated by patient factors and surgical expertise and AI models will not replace the importance of patient selection and surgeon competence. The field of AI is growing rapidly, and the potential applications of ML and other AI technologies in medical imaging are yet to be fully realized.
The application of AI in medical imaging, particularly in breast surgery dates back to 1992, where a computer system was used to detect microcalcifications in mammography (22). Overtime, AI has advanced to the point where AI systems are now being reported to outperform human radiologists with years of experience in reporting mammograms, displaying fewer false negatives and false positives (23). Moreover, in 2017, an AI-based radiology reporting algorithm was approved by the Food and Drug Administration in the USA for use in clinical practice (24). Numerous other developments of AI technology have been seen across several medical specialties, including retinal imaging in ophthalmology, cardiac imaging in cardiology and neurological imaging in stroke care (25-27). Furthermore, augmented reality projections have been developed to allow real-time intraoperative surgical planning (28). Despite the growing prevalence of AI in medical imaging and the notable advancements witnessed in recent years, the current role of AI for preoperative planning of free flaps, including the DIEP flap, remains relatively less well established. Mavioso and colleagues in 2020 conducted a study evaluating the efficacy of a semi-automatic image processing algorithm (relying on computer vision technology) in identifying and analysing perforators in the preoperative planning of DIEP flaps (29,30). The authors compared this AI algorithm to traditional, manual evaluation, assessing the accuracy in estimating size and location of perforators, and the time-efficiency of each method. In comparison to traditional manual reporting, the AI algorithm reduced the time spent on the analysis of perforators from 2–3 hours to approximately 30 minutes per scan (29). This led to an estimated total saving of about 80 hours for the 40 patients involved in the study. Yet, the software had mixed results regarding the assessment of perforator size. It performed significantly better in estimating calibres of perforators larger than 1.5 mm, however, it led to a significant increase in error when estimating perforators of 1.5 mm or less (29). The software’s ability to identify the location of perforators was comparable to manual evaluation, but it did introduce a significant vertical error, albeit with a small effect size (29). Despite these limitations, the AI algorithm could be a valuable tool in optimising efficiency in the preoperative planning of DIEP flaps. Similarly, in 2022, Saxena successfully developed a DL model for the automatic segmentation of vessels in synthetic images of vascular trees (31). Despite the models being trained on a small dataset of synthetic images, they generalized well as indicated by the high sensitivity and specificity on the test set (31). The authors plan to utilize transfer learning to fine-tune the model on real images, anticipating that the synthetic pre-training will assist the model in identifying features associated with vessel structures, and hence perform better with more complex, real cases (31). Therefore AI’s pattern recognition capabilities allow for the analysis of image attributes such as texture, shape, and intensity, enabling the identification of patterns potentially missed in human interpretation (32). This level of detailed analysis can result in a more accurate and consistent selection of perforators, potentially even leading to improved surgical outcomes (33). Furthermore, by automating the perforator selection process, AI applications can substantially reduce the time traditionally invested in preoperative imaging analysis. These studies highlight the potential utility of AI in optimising efficiency, accuracy and reliability in preoperative planning of breast reconstruction (34).
The application of AI in the preoperative planning of free flap breast reconstruction is currently in its infancy, with limited integration into clinical practice. The future direction of using AI in the preoperative planning of free flap breast reconstruction offers exciting potential to advance the field, with the capability to quickly analyse large volumes of data, predict outcomes, and accurately plan for complex surgical procedures. However, this prospect also presents notable challenges and ethical limitations (35,36). Given the reliance of AI models on datasets, the risk of potential bias emerges—bias that could stem from skewed datasets due to socioeconomic, racial, or geographic disparities in healthcare access. Therefore, ensuring fairness and equality in AI algorithms and in the data, they’re trained on is crucial. Such biases could lead to inaccurate predictions and assessments for patients that do not fit the parameters on which the model was based—potentially leading to inaccurate decision making preoperatively. Additionally, questions of patient consent and privacy arise with the utilization of health data for AI training. The transparency of AI decision-making also poses challenges. Though AI could be highly accurate, the so-called “black box” problem—wherein AI processes data and makes recommendations without a clear, understandable rationale—can lead to ethical dilemmas. AI’s potential to hasten preoperative planning and, perhaps operative durations, may lower healthcare costs, yet upfront investment in technology and training could offset these savings. It’s critical to perform cost-benefit analyses and ensure equitable care access, preventing disparities that could arise from the implementation of AI in healthcare settings. Therefore, AI implementation in healthcare must be accompanied by robust guidelines for data use, mechanisms to identify and mitigate bias, and efforts to enhance algorithmic transparency.
Despite the challenges, AI presents novel opportunities in preoperative planning of breast reconstruction, promising to advance this aspect of healthcare. The power of AI necessitates thoughtful implementation, safeguarded by robust regulations and ethical guidelines to ensure benefits are fairly distributed and potential misuse is mitigated. While there is still much research to be done to realize the full potential of AI in this area, the early signs are promising. The integration of AI in breast reconstruction surgery exemplifies the incredible potential for AI in medicine.
Acknowledgments
Funding: None.
Footnote
Peer Review File: Available at https://gs.amegroups.com/article/view/10.21037/gs-23-265/prf
Conflicts of Interest: All authors have completed the ICMJE uniform disclosure form (available at https://gs.amegroups.com/article/view/10.21037/gs-23-265/coif). WMR serves as an unpaid Associate Editor of Gland Surgery from March 2023 to February 2028. IS serves as an unpaid editorial board member of Gland Surgery from September 2023 to August 2025. The other author has no conflicts of interest to declare.
Ethical Statement: The authors are accountable for all aspects of the work in ensuring that questions related to the accuracy or integrity of any part of the work are appropriately investigated and resolved. The study was conducted in accordance with the Declaration of Helsinki (as revised in 2013). No institutional ethics of this report was required. Full informed consent was obtained from the patient whose abdominal CTA reconstruction was used in
Open Access Statement: This is an Open Access article distributed in accordance with the Creative Commons Attribution-NonCommercial-NoDerivs 4.0 International License (CC BY-NC-ND 4.0), which permits the non-commercial replication and distribution of the article with the strict proviso that no changes or edits are made and the original work is properly cited (including links to both the formal publication through the relevant DOI and the license). See: https://creativecommons.org/licenses/by-nc-nd/4.0/.
References
- Cevik J, Hunter-Smith DJ, Rozen WM. Current Advances in Breast Reconstruction. J Clin Med 2022;11:3328. [Crossref] [PubMed]
- Allen RJ, Treece P. Deep inferior epigastric perforator flap for breast reconstruction. Ann Plast Surg 1994;32:32-8. [Crossref] [PubMed]
- Hembd A, Teotia SS, Zhu H, et al. Optimizing Perforator Selection: A Multivariable Analysis of Predictors for Fat Necrosis and Abdominal Morbidity in DIEP Flap Breast Reconstruction. Plast Reconstr Surg 2018;142:583-92. [Crossref] [PubMed]
- Colakoglu S, Tebockhorst S, Freedman J, et al. CT angiography prior to DIEP flap breast reconstruction: a randomized controlled trial. J Plast Reconstr Aesthet Surg 2022;75:45-51. [Crossref] [PubMed]
- Rozen WM, Ashton MW, Grinsell D, et al. Establishing the case for CT angiography in the preoperative imaging of abdominal wall perforators. Microsurgery 2008;28:306-13. [Crossref] [PubMed]
- Canizares O, Mayo J, Soto E, et al. Optimizing Efficiency in Deep Inferior Epigastric Perforator Flap Breast Reconstruction. Ann Plast Surg 2015;75:186-92. [Crossref] [PubMed]
- Fitzgerald O'Connor E, Rozen WM, Chowdhry M, et al. Preoperative computed tomography angiography for planning DIEP flap breast reconstruction reduces operative time and overall complications. Gland Surg 2016;5:93-8. [Crossref] [PubMed]
- Haddock NT, Dumestre DO, Teotia SS. Efficiency in DIEP Flap Breast Reconstruction: The Real Benefit of Computed Tomographic Angiography Imaging. Plast Reconstr Surg 2020;146:719-23. [Crossref] [PubMed]
- Cevik J, Rozen W. A Novel optimization technique of Computed Tomography Angiographic 3D-reconstructions for pre-operative planning of DIEP flaps. JPRAS Open 2023;35:38-41. [Crossref] [PubMed]
- Cevik J, Seth I, Hunter-Smith DJ, et al. A History of Innovation: Tracing the Evolution of Imaging Modalities for the Preoperative Planning of Microsurgical Breast Reconstruction. J Clin Med 2023;12:5246. [Crossref] [PubMed]
- Rozen WM, Bhullar HK, Hunter-Smith D. How to assess a CTA of the abdomen to plan an autologous breast reconstruction. Gland Surg 2019;8:S291-6.
- Cevik J, Hunter-Smith DJ, Rozen WM. The importance of perioperative team familiarity and its contribution to surgical efficiency and outcomes in microsurgical breast reconstruction. Gland Surg 2023;12:1-4. [Crossref] [PubMed]
- Shi YC, Li J, Li SJ, et al. Flap failure prediction in microvascular tissue reconstruction using machine learning algorithms. World J Clin Cases 2022;10:3729-38. [Crossref] [PubMed]
- Aggarwal R, Sounderajah V, Martin G, et al. Diagnostic accuracy of deep learning in medical imaging: a systematic review and meta-analysis. NPJ Digit Med 2021;4:65. [Crossref] [PubMed]
- Geras KJ, Wolfson S, Shen Y, et al. High-resolution breast cancer screening with multi-view deep convolutional neural networks. arXiv:1703.07047. 2017. Available online: https://arxiv.org/abs/1703.07047
- Jordan MI, Mitchell TM. Machine learning: Trends, perspectives, and prospects. Science 2015;349:255-60. [Crossref] [PubMed]
- Varoquaux G, Cheplygina V. Machine learning for medical imaging: methodological failures and recommendations for the future. NPJ Digit Med 2022;5:48.
- Oren O, Gersh BJ, Bhatt DL. Artificial intelligence in medical imaging: switching from radiographic pathological data to clinically meaningful endpoints. Lancet Digit Health 2020;2:e486-8.
- Kim M, Yun J, Cho Y, et al. Deep Learning in Medical Imaging. Neurospine 2019;16:657-68. [Crossref] [PubMed]
- O'Neill AC, Yang D, Roy M, et al. Development and Evaluation of a Machine Learning Prediction Model for Flap Failure in Microvascular Breast Reconstruction. Ann Surg Oncol 2020;27:3466-75. [Crossref] [PubMed]
- Asaad M, Lu SC, Hassan AM, et al. The Use of Machine Learning for Predicting Complications of Free-Flap Head and Neck Reconstruction. Ann Surg Oncol 2023;30:2343-52. [Crossref] [PubMed]
- Driver CN, Bowles BS, Bartholmai BJ, et al. Artificial Intelligence in Radiology: A Call for Thoughtful Application. Clin Transl Sci 2020;13:216-8. [Crossref] [PubMed]
- McKinney SM, Sieniek M, Godbole V, et al. International evaluation of an AI system for breast cancer screening. Nature 2020;577:89-94. [Crossref] [PubMed]
- Arterys. Available online: https://www.arterys.com
- Zhao MY, Fan AP, Chen DY, et al. Cerebrovascular reactivity measurements using simultaneous (15)O-water PET and ASL MRI: Impacts of arterial transit time, labeling efficiency, and hematocrit. Neuroimage 2021;233:117955. [Crossref] [PubMed]
- Raimondi F, Martins D, Coenen R, et al. Prevalence of Venovenous Shunting and High-Output State Quantified with 4D Flow MRI in Patients with Fontan Circulation. Radiol Cardiothorac Imaging 2021;3:e210161. [Crossref] [PubMed]
- Armstrong GW, Lorch AC A. (eye): A Review of Current Applications of Artificial Intelligence and Machine Learning in Ophthalmology. Int Ophthalmol Clin 2020;60:57-71. [Crossref] [PubMed]
- Masterton G, Miller R, Patel J, et al. HoloLens in Breast Reconstruction: What Is the Future? Plast Reconstr Surg 2023;151:915e-7e. [Crossref] [PubMed]
- Mavioso C, Araújo RJ, Oliveira HP, et al. Automatic detection of perforators for microsurgical reconstruction. Breast 2020;50:19-24. [Crossref] [PubMed]
- Mavioso C, Anacleto JC, Vasconcelos MA, et al. The development of an automatic tool to improve perforators detection in Angio CT in DIEAP flap breast reconstruction. Eur J Cancer 2018;92:S74.
- Saxena E. Deep Learning for Personalized Preoperative Planning of Microsurgical Free Tissue Transfers. Proceedings of the AAAI Conference on Artificial Intelligence. 2022;36:13140-1.
- Litjens G, Kooi T, Bejnordi BE, et al. A survey on deep learning in medical image analysis. Med Image Anal 2017;42:60-88. [Crossref] [PubMed]
- Lambin P, Leijenaar RTH, Deist TM, et al. Radiomics: the bridge between medical imaging and personalized medicine. Nat Rev Clin Oncol 2017;14:749-62. [Crossref] [PubMed]
- Zhao L, Patel PK, Cohen M. Application of virtual surgical planning with computer assisted design and manufacturing technology to cranio-maxillofacial surgery. Arch Plast Surg 2012;39:309-16. [Crossref] [PubMed]
- Cabitza F, Rasoini R, Gensini GF. Unintended Consequences of Machine Learning in Medicine. JAMA 2017;318:517-8. [Crossref] [PubMed]
- Leslie D. Understanding artificial intelligence ethics and safety. arXiv:1906.05684. 2019. Available online: https://arxiv.org/abs/1906.05684