Development and validation of an ultrasonography and clinicopathological features-based nomogram for non-sentinel lymph node metastasis
Highlight box
Key findings
• We constructed a nomogram model of non-sentinel lymph node metastasis (NSLNM) based on ultrasonic and clinicopathological features to accurately assess the risk of invasive breast cancer with NSLNM.
What is known and what is new?
• It is now known that in order to accurately estimate the risk of NSLNM and omit excessive axillary treatment, many national medical institutions have established NSLNM prediction models.
• This manuscript incorporated ultrasonic image features into the prediction model.
What are the implications, and what should change now?
• In the future, subsequent clinical validation studies of multi-center large-scale samples should be conducted, and the prediction model should be adjusted more precisely.
Introduction
The lymphatic metastasis of breast cancer (BC) mainly returns to the ipsilateral armpit through lymphatic vessels, so the management of the armpit is an important aspect of BC surgery. From the previous century, axillary lymph node dissection (ALND) has been the standard for the treatment of axillary lymph nodes (ALNs). However, the complications that are associated ALND, including limb lymphedema, local nerve injury, and shoulder joint dysfunction, negatively impact the patients’ quality of life (1). Following the proposal of the Milan trial (2) in recent years, many prospective clinical trials (3-5) with large samples have confirmed the safety and clinical practicability of sentinel lymph node biopsy (SLNB) as a replacement for ALND in patients with negative sentinel lymph nodes (SLNs). CBCSG-01 (5) prospective clinical trials revealed that patients with negative SLNs have five-year overall survival rates and disease-free survival rates of 98.2% and 94.2%, respectively. NSABP B-32 (4) clinical trials showed that the overall accuracy of SLNB in patients was 97.1% and false negative (FN) rates was 9.8%. ALMANAC (3) clinical trials found that the overall accuracy of SLNB in patients was 97.6% and FN rates were 6.7%.
Some previous studies suggested that patients with positive SLNs could be exempted from ALND in certain conditions. In study IBCSG 23-01 (6) found that BC patients with primary tumor ≤5 cm and one or more SLNs with micrometastases (≤2 mm) are liable to an exemption from ALND. A 10-year follow-up of ACOSOG Z0011 (7) further confirmed that patients with cT1-2N0 and one or two SLNs BC can be exempted from ALND under the condition of breast-conserving surgery and whole breast radiotherapy. According to the AMAROS (8) study, axillary radiotherapy can be used as an alternative to ALND for BC patients who have more than two SLNs after a simple mastectomy. The same applies to those who currently have no postoperative radiation therapy plans or a history of breast-conserving surgery. Moreover, 40–70% of patients with positive SLNs were observed to have no extra ALN metastasis, so ALND seems to be an overtreatment in such cases. Although these studies confirmed the safety of SLNB in cases of limited tumor burden without ALND, many scholars still have serious concerns about the intervention. Positive lymph nodes beyond SLNs that are not removed will result in a residual tumor, which can cause axillary recurrence (9). Therefore, the establishment of a model that efficiently predicts axillary non-sentinel lymph node metastasis (NSLNM) could better manage patients with positive SLNs.
The first NSLNM risk prediction model that was developed by Memorial Sloan-Kettering Cancer Center (MSKCC) (10) is the most widely used. This model, which is currently the most verified, provides a percentage corresponding to the risk of NSLNM in SLN-positive patients. Due to the variations in in surgical methods, performer levels, pathological diagnostic criteria, and characteristics of BC patients in different countries and regions, there are discrepancies in the prediction results of the MSKCC model in different countries, with an area under the curve (AUC) ranging from 0.58 and 0.86 (10-15).
In addition to the MSKCC model, there are numerous other NSLNM risk prediction models worldwide, such as the MD Anderson (MDA), Mayo, Tenon, Cambridge and Stanford model. All these models were based on the analysis of the morbidity and pathological characteristics, as well as other factors that are associated with domestic patients. This was done to establish a prediction model that has high accuracy. However, none of these prediction model applied to the Chinese population, in addition to the fact that they do not include ultrasound image features. A metastatic ALN is commonly characterized by a cortical thickness of ≥2.5–3.0 mm (16), a lobulated cortex, loss of the hilum, a round shape, and an abnormal cortical blood flow (17). Moreover, Jiang et al. (18) found that shear-wave elastography and ultrasound image features were associated with ALN metastasis. Also, the experimental results that were reported by Fu et al. (19) indicated that magnetic resonance imaging (MRI) is worthy of clinical promotion and usage, based on its ability to diagnose the ALN metastasis of BC. However, MRI was limited by factors such as high sensitivity and costs, low specificity, and the long time for preliminary diagnosis (20), so its diagnostic value was not discussed in this study. Therefore, an NSLNM risk prediction model based on the Chinese population needs to be developed to further guide clinical treatment.
In this study, a nomogram model for predicting the axillary NSLNM risk was established based on the ultrasonographical and clinicopathological characteristics of female patients with invasive BC. This helps to provide a reference for clinicians regarding the management of ALNs. We present the following article in accordance with the TRIPOD reporting checklist (available at https://gs.amegroups.com/article/view/10.21037/gs-23-58/rc).
Methods
Patient selection
This study retrospectively collected the data of patients with invasive BC who underwent SLNB and ALND in Ningbo Medical Treatment Center Lihuili Hospital from January 1, 2012, to May 1, 2022. The exclusion criteria were as follows: (I) pathologically T4 BC; (II) bilateral or occult BC; (III) metastatic BC (MBC); (IV) previous history of axillary surgery; (V) neoadjuvant treatment; and (VI) incomplete data. Figure 1 displays a flow chart of the study. The study was conducted in accordance with the Declaration of Helsinki (as revised in 2013). This study was approved by the Ethics Committee of Ningbo Medical Treatment Center Lihuili Hospital (No. 2022-261), and the requirement for written informed consent to participate was waived due to the retrospective nature of the study. The patients included in the study were randomly assigned to training and validation sets at a ratio of 7:3 for the model establishment and internal validation.
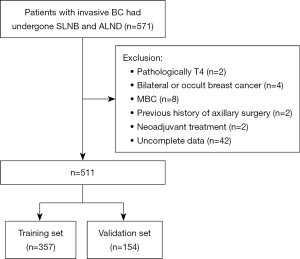
Collection of the ultrasonography and clinicopathological characteristics
The following information was collected: (I) general information of patients: age at onset, menstruation, body mass index (BMI), and surgical procedures; (II) the characteristics of ultrasound imaging: tumor location, tumor quadrant, maximum tumor diameter, tumor margin, blood flow signal, posterior echo, aspect ratio, Breast Imaging Reporting and Data System (BI-RADS) classification, ALNs, lymph node shape, focal cortical status, cortex thickness, and lymphatic hilum state; and (III) clinicopathological characteristics: histological type, histological grading, number of lesions, vascular invasion, nerve invasion, positive SLN number, negative SLN number, SLN metastasis rate, non-SLN status, hormone receptors, human epidermal growth factor receptor 2 (HER2), Ki-67, and other clinical and pathological data.
Statistical analysis
IBM SPSS Statistics (version 25.0, SPSS Inc, Chicago, IL, USA) and R Software (version 3.5.3, http://www.r-project.org) were used for data analysis. The hypothesis test level was set at an alpha of 0.05 for all data. Continuous variables were tested for normality using the Kolmogorov-Smimov test; if the distribution was normal, the t-test was used, otherwise, the Mann-Whitney U test was employed. Categorical variables were analyzed using the chi-square test or Fisher’s exact test. The related variables of NSLNM were selected by univariate and multivariate analyses. Then, a digital nomogram prediction model was established and a nomogram was drawn. The efficiency test of the model formula was assessed using a receiver operating characteristic (ROC) curve, correction curve, and Hosmer-Lemeshow (HL) goodness-of-fit test.
Results
Patients’ characteristics
This study included 511 patients diagnosed with BC, with 357 in the training set and 154 in the validation set based on a ratio of 7:3 using R software. The age of the enrolled patients ranged from 26 to 88 years old, with a median age of 52 years. We compared the features of patients in the training and validation sets (see Table 1).
Table 1
Factors | Classification | Training set (n=357) | Validation set (n=154) | χ2/Z | P |
---|---|---|---|---|---|
Age (years), median [range] | – | 51 [26–88] | 52 [27–82] | 0.03 | 0.613 |
BMI (kg/m2) | ≤18.5 | 6 (1.7) | 1 (0.6) | 0.64 | 0.909 |
18.5–24.9 | 116 (32.5) | 47 (30.5) | |||
25.0–29.9 | 96 (26.9) | 48 (31.2) | |||
>29.9 | 139 (38.9) | 58 (37.7) | |||
Menstruation | Menopausal | 212 (59.4) | 87 (56.5) | 0.37 | 0.558 |
Pre-menopausal | 145 (40.6) | 67 (43.5) | |||
Procedures | BCT | 121 (33.9) | 43 (27.9) | 1.76 | 0.185 |
MRM | 236 (66.1) | 111 (72.1) | |||
Location | Left | 188 (52.7) | 81 (52.6) | 0.00 | 1.000 |
Right | 169 (47.3) | 73 (47.4) | |||
Quadrant | Outer up | 159 (44.5) | 53 (34.4) | 6.18 | 0.102 |
Outer down | 70 (19.6) | 29 (18.8) | |||
Inner down | 58 (16.2) | 35 (22.7) | |||
Inner up | 70 (19.6) | 37 (24.0) | |||
Diameter (cm) | ≤2 | 204 (57.1) | 85 (55.2) | 5.81 | 0.052 |
2–5 | 147 (41.2) | 66 (42.9) | |||
>5 | 6 (1.7) | 3 (1.9) | |||
Histological type | IDC | 341 (95.5) | 144 (93.5) | 0.90 | 0.342 |
Other | 16 (4.5) | 10 (6.5) | |||
Grade | I | 13 (3.6) | 4 (2.6) | 1.03 | 0.631 |
II | 234 (65.5) | 108 (70.1) | |||
III | 110 (30.8) | 42 (27.3) | |||
Lesions | Single | 320 (89.6) | 144 (93.5) | 1.76 | 0.403 |
Two | 12 (3.4) | 2 (1.3) | |||
Multiple | 25 (7.0) | 8 (5.2) | |||
VI | No | 75 (21.0) | 39 (25.3) | 1.16 | 0.298 |
Infiltration | 282 (79.0) | 115 (74.7) | |||
NI | No | 301 (84.3) | 137 (89.0) | 1.90 | 0.215 |
Infiltration | 56 (15.7) | 17 (11.0) | |||
SLN+ | 1 | 247 (69.2) | 108 (70.1) | 3.36 | 0.340 |
2 | 63 (17.6) | 27 (17.5) | |||
3 | 29 (8.1) | 7 (4.5) | |||
≥4 | 18 (5.0) | 12 (7.8) | |||
SLN− | 0 | 34 (9.5) | 14 (9.1) | 1.80 | 0.773 |
1 | 64 (17.9) | 29 (18.8) | |||
2 | 88 (24.6) | 30 (19.5) | |||
3 | 70 (19.6) | 33 (21.4) | |||
≥4 | 101 (28.3) | 48 (31.2) | |||
SLN% | 0–25% | 121 (33.9) | 58 (37.7) | 0.24 | 0.462 |
26–50% | 157 (44.0) | 60 (39.0) | |||
51–75% | 32 (9.0) | 16 (10.4) | |||
76–100% | 47 (13.2) | 20 (13.0) | |||
ER | Positive | 273 (76.5) | 112 (72.7) | 0.81 | 0.373 |
Negative | 84 (23.5) | 42 (27.3) | |||
PR | Positive | 236 (66.1) | 104 (67.5) | 0.10 | 0.761 |
Negative | 121 (33.9) | 50 (32.5) | |||
HER2 | Positive | 87 (24.4) | 28 (18.2) | 2.36 | 0.135 |
Negative | 270 (75.6) | 126 (81.8) | |||
Ki-67 | ≤15% | 107 (30.0) | 47 (30.5) | 0.45 | 0.801 |
15–30% | 126 (35.3) | 58 (37.7) | |||
>30% | 124 (34.7) | 49 (31.8) | |||
Margins | Irregular | 336 (94.1) | 151 (98.1) | 4.44 | 0.067 |
Regular | 21 (5.9) | 3 (1.9) | |||
Flow signal | Detected | 185 (51.8) | 79 (51.3) | 0.01 | 0.923 |
No | 172 (48.2) | 75 (48.7) | |||
Posterior echo | Attenuation | 171 (47.9) | 80 (51.9) | 0.71 | 0.441 |
No | 186 (52.1) | 74 (48.1) | |||
Aspect ratio | >1 | 134 (37.5) | 71 (46.1) | 3.29 | 0.077 |
≤1 | 223 (62.5) | 83 (53.9) | |||
BI-RADS | 3 | 17 (4.8) | 6 (3.9) | 2.272 | 0.686 |
4A | 63 (17.4) | 33 (21.4) | |||
4B | 116 (32.5) | 55 (35.7) | |||
4C | 124 (34.7) | 46 (29.9) | |||
5 | 37 (10.4) | 14 (9.1) | |||
NSLN | Positive | 113 (31.7) | 49 (31.8) | 0.00 | 0.971 |
Negative | 244 (68.3) | 105 (68.2) | |||
ALN | Detected | 49 (13.7) | 19 (12.3) | 0.18 | 0.672 |
No | 308 (86.3) | 135 (87.7) | |||
LN shape | Blurred | 15 (4.2) | 3 (1.9) | 1.61 | 0.205 |
Clear | 342 (95.8) | 151 (98.1) | |||
Focal cortical status | Lobulation | 3 (0.8) | 1 (0.6) | 0.05 | 1.000 |
No | 354 (99.2) | 153 (99.4) | |||
Cortex thickness | Incrassated | 21 (5.9) | 5 (3.2) | 1.55 | 0.275 |
No | 336 (94.1) | 149 (96.8) | |||
Hilum | Disappeared | 16 (4.5) | 7 (4.5) | 0.00 | 1.000 |
No | 341 (95.5) | 147 (95.5) |
Except for age presented as median, all the others were presented as No. (%). BMI, body mass index; BCT, breast-conserving therapy; MRM, modified radical mastectomy; IDC, invasive ductal breast cancer; VI, vascular invasion; NI, neural invasion; SLN+, positive SLN number; SLN−, negative SLN number; SLN%, sentinel lymph node metastasis rate; ER, estrogen receptor; PR, progesterone receptor; HER2, human epidermal growth factor receptor 2; BI-RADS, breast imaging reporting and data system; NSLN, non-sentinel lymph node; ALN, axillary lymph node; LN, lymph node.
Establishment of the prediction model
Univariate analysis of NSLNM
Univariate analysis showed that the following variables were significantly associated with the incidence of NSLNM: vascular invasion, positive SLN number, negative SLN number, SLN metastasis rate, BI-RADS classification, ALN status, lymph node shape, focal cortical status, cortex thickness, lymphatic hilum state, HER2 status (P<0.05), and the relationships between NSLNM and clinicopathological characteristics (Table 2).
Table 2
Factors | Classification | Case | NSLN+ | NSLN− | χ2/Z | P |
---|---|---|---|---|---|---|
Age (years), median [range] | – | – | 51 [28–88] | 51 [28–88] | −1.22 | 0.221 |
BMI (kg/m2) | ≤18.5 | 6 | 1 | 5 | 2.27 | 0.518 |
18.5–24.9 | 116 | 32 | 84 | |||
25.0–29.9 | 96 | 34 | 62 | |||
>29.9 | 139 | 46 | 93 | |||
Menstruation | Menopausal | 212 | 69 | 143 | 0.19 | 0.660 |
Pre-menopausal | 145 | 44 | 101 | |||
Method | BCT | 121 | 35 | 86 | 0.63 | 0.428 |
MRM | 236 | 78 | 158 | |||
Location | Left | 188 | 66 | 122 | 2.19 | 0.139 |
Right | 169 | 47 | 122 | |||
Quadrant | Outer up | 159 | 51 | 108 | 3.49 | 0.322 |
Outer down | 70 | 22 | 48 | |||
Inner down | 58 | 23 | 35 | |||
Inner up | 70 | 17 | 53 | |||
Diameter (cm) | ≤2 | 204 | 60 | 144 | 1.11 | 0.574 |
2–5 | 147 | 51 | 96 | |||
>5 | 6 | 2 | 4 | |||
Histological type | IDC | 341 | 109 | 232 | 0.34 | 0.558 |
Other | 16 | 4 | 12 | |||
Grade | I | 13 | 4 | 9 | 2.34 | 0.311 |
II | 234 | 68 | 166 | |||
III | 110 | 41 | 69 | |||
Lesions | Single | 320 | 99 | 221 | 0.85 | 0.654 |
Two | 12 | 5 | 7 | |||
Multiple | 25 | 9 | 16 | |||
VI | No | 75 | 13 | 62 | 9.00 | 0.003 |
Infiltration | 282 | 100 | 182 | |||
NI | No | 301 | 93 | 208 | 0.51 | 0.477 |
Infiltration | 56 | 20 | 36 | |||
SLN+ | 1 | 247 | 51 | 196 | 102.36 | 0.000 |
2 | 63 | 32 | 31 | |||
3 | 29 | 16 | 13 | |||
≥4 | 18 | 14 | 4 | |||
SLN− | 0 | 34 | 23 | 11 | 33.14 | 0.000 |
1 | 64 | 27 | 37 | |||
2 | 88 | 27 | 61 | |||
3 | 70 | 17 | 53 | |||
≥4 | 101 | 19 | 82 | |||
SLN% | 0–25% | 121 | 20 | 101 | 47.70 | 0.000 |
26–50% | 157 | 45 | 112 | |||
51–75% | 32 | 19 | 13 | |||
76–100% | 47 | 29 | 18 | |||
ER | Positive | 274 | 88 | 186 | 0.12 | 0.732 |
Negative | 83 | 25 | 58 | |||
PR | Positive | 235 | 76 | 159 | 0.15 | 0.698 |
Negative | 122 | 37 | 85 | |||
HER2 | Positive | 87 | 45 | 42 | 21.42 | 0.000 |
Negative | 270 | 68 | 202 | |||
Ki-67 | ≤15% | 108 | 33 | 75 | 0.34 | 0.845 |
15–30% | 125 | 42 | 83 | |||
>30% | 124 | 38 | 86 | |||
Margins | Irregular | 335 | 108 | 227 | 0.86 | 0.353 |
Regular | 22 | 5 | 17 | |||
Flow signal | Detected | 185 | 57 | 128 | 0.13 | 0.723 |
No | 172 | 56 | 116 | |||
Posterior echo | Attenuation | 172 | 59 | 113 | 1.08 | 0.299 |
No | 185 | 54 | 131 | |||
Aspect ratio | >1 | 134 | 44 | 90 | 0.14 | 0.709 |
≤1 | 223 | 69 | 154 | |||
BI-RADS | 3 | 17 | 0 | 17 | 31.66 | 0.000 |
4A | 63 | 7 | 56 | |||
4B | 116 | 38 | 78 | |||
4C | 124 | 48 | 76 | |||
5 | 37 | 20 | 17 | |||
ALN | Positive | 49 | 31 | 18 | 26.24 | 0.000 |
Negative | 308 | 82 | 226 | |||
LN shape | Blurred | 15 | 14 | 1 | 27.54 | 0.000 |
Clear | 342 | 99 | 243 | |||
Focal cortical status | Lobulation | 3 | 3 | 0 | 6.53 | 0.008 |
No | 354 | 110 | 244 | |||
Cortex thickness | Incrassated | 21 | 15 | 6 | 16.63 | 0.000 |
No | 336 | 98 | 238 | |||
Hilum | Disappeared | 16 | 13 | 3 | 19.05 | 0.000 |
No | 341 | 100 | 241 |
SLN+, positive SLN number; SLN−, negative SLN number; BMI, body mass index; BCT, breast-conserving therapy; MRM, modified radical mastectomy; IDC, invasive ductal breast cancer; VI, vascular invasion; NI, neural invasion; SLN%, sentinel lymph node metastasis rate; ER, estrogen receptor; PR, progesterone receptor; HER2, human epidermal growth factor receptor 2; BI-RADS, breast imaging reporting and data system; NSLN, non-sentinel lymph node; ALN, axillary lymph node; LN, lymph node.
Multivariate analysis of NSLNM
Multivariate analysis showed that vascular infiltration (P=0.009), positive SLN number (P=0.004), HER2 overexpression (P<0.001), and lymph node shape (P=0.002) are independent statistically significant predictors of NSLNM, while negative SLN number (P=0.002) was an independent protective factor of NSLNM. The details are presented in Table 3.
Table 3
Variables | B | STD | Wald | P | OR | 95% CI |
---|---|---|---|---|---|---|
VI | 1.07 | 0.406 | 6.91 | 0.009 | 2.91 | 1.356 to 6.725 |
SLN+ | 0.59 | 0.167 | 12.69 | 0.004 | 1.80 | 1.310 to 2.516 |
SLN− | −0.42 | 0.114 | 13.59 | 0.002 | 0.66 | 0.524 to 0.819 |
HER2 | 1.23 | 0.321 | 14.72 | <0.001 | 3.42 | 1.836 to 6.483 |
LN shape | 4.28 | 1.355 | 9.99 | 0.002 | 72.40 | 6.661 to 1,924.124 |
STD, standard deviation; OR, odds ratio; CI, confidence interval; VI, vascular invasion; SLN+, positive sentinel lymph node number; SLN−, negative sentinel lymph node number; HER2, human epidermal growth factor receptor 2; LN, lymph node.
Preliminary establishment of the nomogram prediction model
R software was used to develop a nomogram prediction model (Figure 2) consisting of five variables: vascular invasion, positive SLN number, negative SLN number, HER2 status, and lymph node shape. A ROC of the subjects was drawn, which showed an AUC of 0.793 (Figure 3A).
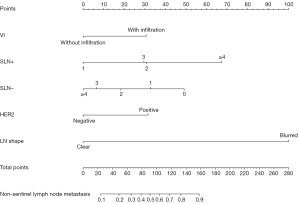
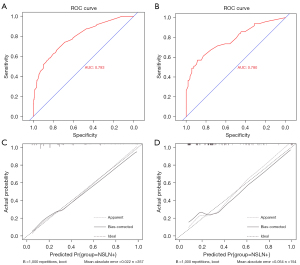
Evaluation of the prediction model
The data of 154 patients in the validation set were used to validate the predictive effect of the nomogram model, and the ROC curve was drawn. The AUC was 0.780, indicating that the differentiation ability of the model was good (Figure 3B). The calibration curves of training and validation sets were drawn (Figure 3C,3D). Meanwhile, the calibration of the model was assessed by the HL test (training set: χ2=11.79, P=0.161; validation set: χ2=4.904, P=0.768). At the same time, decision curve analysis (DCA) curve was drawn for all patients (Figure 4), and it was found that the curve threshold was 0–0.96, which proved that this model had high clinical application value. The P values were greater than 0.05 and the prediction model showed good calibration ability. At the cut-off points of 10% and 15% for NSLNM, the FN rates of the nomogram were 1.85% and 8.64%, and the negative predictive values (NPVs) of the nomogram were 96.59% and 89.89%, respectively (Table 4).
Table 4
Cut-off values | No. of patients | FN rate (%) | FN patients | NPV (%) | Sensitivity (%) | Specificity (%) |
---|---|---|---|---|---|---|
10% | 88 | 1.85 | 3 | 96.59 | 98.15 | 24.36 |
15% | 178 | 8.64 | 18 | 89.89 | 88.89 | 45.85 |
Specificity: the percentage of the truly negative patients labeled as “negative” by the model; Sensitivity: the percentage of the truly positive patients labeled as “positive”. NSLNM, non-sentinel lymph node metastasis; FN, false-negative; NPV, negative predictive value.
Discussion
After decades of clinical practice, SLNB has become the standard of axillary staging for early BC and clinical node-negative patients (21). Trials such as IBCSG 23-01 (6), ACOSOG Z0011 (7), and AMAROS (8) have coherently shown that ALND should not be performed on patients with positive SLNs under specific predetermined circumstances. On the other hand, there is ongoing debate regarding the safety and dangers that are associated with exempting patients with positive SLNs from receiving ALND. To accurately estimate the risk of NSLNM, medical institutions in various countries have established NSLNM prediction models [MDA (22), Mayo (23), Tenon (24), Cambridge (13), and Stanford (25)]. For patients with a low risk of metastasis, excessive axillary treatment can be omitted. It is important to note that, the indicators included in each prediction model are not identical, and factors such as poor repeatability ad well as stability and regional differences are a cause for concern.
Vascular infiltration is regarded as an independent predictor of NSLNM in patients with positive SLNs in the MSKCC (10), MDA (22), and Stanford (25) models, as is the case with some researchers’ own models (26,27). In this study, multivariate analysis in the training set showed that vascular infiltration was a risk factor of NSLNM [odds ratio (OR) =2.91; 95% confidence interval (CI): 1.356–6.725; P=0.009].
In previous studies, the number of positive SLNs was found to be associated with NSLNM in the MSKCC (10) and MDA (22) models, while the number of negative SLNs was found to be an independent protective factor for NSLNM. Similar conclusions were also made in this study (OR =1.80; 95% CI: 1.310–2.516; P=0.004). The SLN metastasis rates of patients with only one positive SLN and more than three positive SLNs were 20.6% and 77.7%, respectively. The risk of metastasis increased with the rise in the number of positive SLNs. Apparently, the number of negative SLN is an important factor for predicting NSLNM, and this has also been confirmed by a previous study (28).
The overexpression of HER2 is related to the occurrence and invasion of tumors. Although the MSKCC (10), MDA (22), Stanford (25), and Shanghai Cancer Hospital (SCH) (15) models did not include this factor in their studies, many subsequent domestic studies have demonstrated that HER2 overexpression is an independent risk factor for NSLNM (29,30). These findings are consistent with the results of the present study (OR =3.42; 95% CI: 1.836–6.482; P<0.001).
This study incorporated detailed ultrasound imaging, including primary tumors and lymph nodes. At present, ultrasonography is the preferred screening method for BC. Preoperative ultrasound is helpful in screening patients with a low risk of metastasis (31). Some studies showed that the ratio of the length to the diameter of the lymph node, cortical thickness, focal cortical lobulation, lymphatic hilum state, lymph node shape, and abnormal cortical blood flow were independent predictors of lymph node metastasis (17,32). In addition to these parameters, Qiu et al. (33) reported that the transverse diameter of the lymph node is associated with ALN metastasis. Additionally, Xiong et al. (34) showed that a spiculated margin and distance from the skin were also found to be associated with ALN metastasis. However, there is currently no prediction model for NSLNM that includes ultrasonic imaging characteristics, especially for more detailed analyses of lymph node characteristics. The present study analyzed the following four aspects: lymph node shape, focal cortical lobulation, cortical state, and lymphatic hilum state. These ultrasound features that were incorporated into our model were easily obtained in all BC patients, which guarantees the application of the model. In our study, the univariate analysis results suggested that the P values of the four ultrasonic parameters were all less than 0.05, suggesting that they were related to NSLNM. However, the multivariate analysis showed that only the blurred shape of the lymph node was an independent risk factor for NSLNM (P=0.002).
According to the findings from this study, the AUC of the nomogram prediction model was 0.793. Previous researchers investigated the application value of the MSKCC prediction model in Chinese BC patients, with an AUC of 0.688 (35). Liu et al. (36) verified the MSKCC and SOC models with AUC values of 0.624 and 0.679, respectively, in Chinese populations. The new model that is presented by this study had higher accuracy and discriminating ability. According to a previous study, AUC values between 0.5 and 0.7, 0.7 and 0.9, and equal or above 0.9 showed that the predictive effect was poor, generally accurate, and very good, respectively (37). Therefore, the predictive ability of our model is within acceptable ranges. In this study, the NSLNM rates in the training and validation sets were 31.7% and 31.8%, respectively, and this was consistent with previous literature (4,38,39). In our study, NSLNM was not found in patients with a metastatic probability of less than 6% when the predictive model was applied. According to a study by Poirier et al. (40), most surgeons omitted ALND in patients with positive SLNs if the predicted probability of NSLNM was 10% or less. When the predicted cut-off point was 10%, the FN rate of our model was only 1.85% and the NPV, sensitivity, and specificity were 96.59%, 98.15%, and 24.36%, respectively. When the cut-off point was 15%, the FN rate of the nomogram was 8.64%, and the NPV, sensitivity, and specificity were 89.89%, 88.89%, and 45.85%, respectively. Therefore, when the risk of metastasis that is obtained by applying our study model is less than 10%, omitting the ALND seems relatively acceptable, following discussion with patients.
However, our study also has some limitations that should be noted. Firstly, since our study is a single-center retrospective study with a relatively small sample size, external validation is needed to improve the applicability of the model. Secondly, multiple models have included the size of SLN metastasis among the predictive factors; however, data related to the size of SLN metastasis was lacking in this study. Moreover, the imaging data collected in the study are relatively simple, and mammography and breast MRI could have also been included. Finally, this study did not collect the comprehensive follow-up treatment and prognostic data of patients, which should be considered in future studies. In the future, subsequent clinical validation multi-center studies with large samples should be conducted, and the prediction model should be adjusted more precisely.
Conclusions
In conclusion, based on the samples collected from our center, an NSLNM risk prediction model was established and verified to provide a quantitative way for clinicians who exempt patients with positive SLNs due to ALND concerns to judge the NSLNM risk and make treatment decisions after integrating the risk and patient prognosis.
Acknowledgments
The authors thank the patients included in this study.
Funding: This study was supported by the Medical Scientific Research Foundation of Zhejiang Province, China (Nos. 2022KY1078 and 2023KY1030).
Footnote
Reporting Checklist: The authors have completed the TRIPOD reporting checklist. Available at https://gs.amegroups.com/article/view/10.21037/gs-23-58/rc
Data Sharing Statement: Available at https://gs.amegroups.com/article/view/10.21037/gs-23-58/dss
Conflicts of Interest: All authors have completed the ICMJE uniform disclosure form (available at https://gs.amegroups.com/article/view/10.21037/gs-23-58/coif). The authors have no conflicts of interest to declare.
Ethical Statement:
Open Access Statement: This is an Open Access article distributed in accordance with the Creative Commons Attribution-NonCommercial-NoDerivs 4.0 International License (CC BY-NC-ND 4.0), which permits the non-commercial replication and distribution of the article with the strict proviso that no changes or edits are made and the original work is properly cited (including links to both the formal publication through the relevant DOI and the license). See: https://creativecommons.org/licenses/by-nc-nd/4.0/.
References
- Lucci A, McCall LM, Beitsch PD, et al. Surgical complications associated with sentinel lymph node dissection (SLND) plus axillary lymph node dissection compared with SLND alone in the American College of Surgeons Oncology Group Trial Z0011. J Clin Oncol 2007;25:3657-63. [Crossref] [PubMed]
- Veronesi U, Paganelli G, Viale G, et al. Sentinel-lymph-node biopsy as a staging procedure in breast cancer: update of a randomised controlled study. Lancet Oncol 2006;7:983-90. [Crossref] [PubMed]
- Goyal A, Newcombe RG, Chhabra A, et al. Factors affecting failed localisation and false-negative rates of sentinel node biopsy in breast cancer--results of the ALMANAC validation phase. Breast Cancer Res Treat 2006;99:203-8. [Crossref] [PubMed]
- Krag DN, Anderson SJ, Julian TB, et al. Technical outcomes of sentinel-lymph-node resection and conventional axillary-lymph-node dissection in patients with clinically node-negative breast cancer: results from the NSABP B-32 randomised phase III trial. Lancet Oncol 2007;8:881-8. [Crossref] [PubMed]
- Wang YS, Ouyang T, Wang QT, et al. China multicenter study of sentinel node biopsy substituting axillary node dissection: CBCSG-01 trial. European Journal of Cancer Supplements 2008;6:170-1.
- Galimberti V, Cole BF, Viale G, et al. Axillary dissection versus no axillary dissection in patients with breast cancer and sentinel-node micrometastases (IBCSG 23-01): 10-year follow-up of a randomised, controlled phase 3 trial. Lancet Oncol 2018;19:1385-93. [Crossref] [PubMed]
- Giuliano AE, Ballman KV, McCall L, et al. Effect of Axillary Dissection vs No Axillary Dissection on 10-Year Overall Survival Among Women With Invasive Breast Cancer and Sentinel Node Metastasis: The ACOSOG Z0011 (Alliance) Randomized Clinical Trial. JAMA 2017;318:918-26. [Crossref] [PubMed]
- Donker M, van Tienhoven G, Straver ME, et al. Radiotherapy or surgery of the axilla after a positive sentinel node in breast cancer (EORTC 10981-22023 AMAROS): a randomised, multicentre, open-label, phase 3 non-inferiority trial. Lancet Oncol 2014;15:1303-10. [Crossref] [PubMed]
- Haffty BG, Hunt KK, Harris JR, et al. Positive sentinel nodes without axillary dissection: implications for the radiation oncologist. J Clin Oncol 2011;29:4479-81. [Crossref] [PubMed]
- Van Zee KJ, Manasseh DM, Bevilacqua JL, et al. A nomogram for predicting the likelihood of additional nodal metastases in breast cancer patients with a positive sentinel node biopsy. Ann Surg Oncol 2003;10:1140-51. [Crossref] [PubMed]
- Alran S, De Rycke Y, Fourchotte V, et al. Validation and limitations of use of a breast cancer nomogram predicting the likelihood of non-sentinel node involvement after positive sentinel node biopsy. Ann Surg Oncol 2007;14:2195-201. [Crossref] [PubMed]
- Klar M, Jochmann A, Foeldi M, et al. The MSKCC nomogram for prediction the likelihood of non-sentinel node involvement in a German breast cancer population. Breast Cancer Res Treat 2008;112:523-31. [Crossref] [PubMed]
- Pal A, Provenzano E, Duffy SW, et al. A model for predicting non-sentinel lymph node metastatic disease when the sentinel lymph node is positive. Br J Surg 2008;95:302-9. [Crossref] [PubMed]
- Zgajnar J, Perhavec A, Hocevar M, et al. Low performance of the MSKCC nomogram in preoperatively ultrasonically negative axillary lymph node in breast cancer patients. J Surg Oncol 2007;96:547-53. [Crossref] [PubMed]
- Chen JY, Chen JJ, Yang BL, et al. Predicting sentinel lymph node metastasis in a Chinese breast cancer population: assessment of an existing nomogram and a new predictive nomogram. Breast Cancer Res Treat 2012;135:839-48. [Crossref] [PubMed]
- Man V, Luk WP, Fung LH, et al. The role of pre-operative axillary ultrasound in assessment of axillary tumor burden in breast cancer patients: a systematic review and meta-analysis. Breast Cancer Res Treat 2022;196:245-54. [Crossref] [PubMed]
- Bedi DG, Krishnamurthy R, Krishnamurthy S, et al. Cortical morphologic features of axillary lymph nodes as a predictor of metastasis in breast cancer: in vitro sonographic study. AJR Am J Roentgenol 2008;191:646-52. [Crossref] [PubMed]
- Jiang M, Li CL, Luo XM, et al. Radiomics model based on shear-wave elastography in the assessment of axillary lymph node status in early-stage breast cancer. Eur Radiol 2022;32:2313-25. [Crossref] [PubMed]
- Fu X, Jiang B, Fu J, et al. MRI Diagnosis and Pathological Examination of Axillary Lymph Node Metastasis in Breast Cancer Patients. Contrast Media Mol Imaging 2022;2022:4519982. [Crossref] [PubMed]
- Iima M, Le Bihan D. The road to breast cancer screening with diffusion MRI. Front Oncol 2023;13:993540. [Crossref] [PubMed]
- Lyman GH, Giuliano AE, Somerfield MR, et al. American Society of Clinical Oncology guideline recommendations for sentinel lymph node biopsy in early-stage breast cancer. J Clin Oncol 2005;23:7703-20. [Crossref] [PubMed]
- Hwang RF, Krishnamurthy S, Hunt KK, et al. Clinicopathologic factors predicting involvement of nonsentinel axillary nodes in women with breast cancer. Ann Surg Oncol 2003;10:248-54. [Crossref] [PubMed]
- Degnim AC, Reynolds C, Pantvaidya G, et al. Nonsentinel node metastasis in breast cancer patients: assessment of an existing and a new predictive nomogram. Am J Surg 2005;190:543-50. [Crossref] [PubMed]
- Barranger E, Coutant C, Flahault A, et al. An axilla scoring system to predict non-sentinel lymph node status in breast cancer patients with sentinel lymph node involvement. Breast Cancer Res Treat 2005;91:113-9. [Crossref] [PubMed]
- Kohrt HE, Olshen RA, Bermas HR, et al. New models and online calculator for predicting non-sentinel lymph node status in sentinel lymph node positive breast cancer patients. BMC Cancer 2008;8:66. [Crossref] [PubMed]
- Öz B, Akcan A, Doğan S, et al. Prediction of nonsentinel lymph node metastasis in breast cancer patients with one or two positive sentinel lymph nodes. Asian J Surg 2018;41:12-9. [Crossref] [PubMed]
- Toshikawa C, Koyama Y, Nagahashi M, et al. Predictive Factors for Non-Sentinel Lymph Node Metastasis in the Case of Positive Sentinel Lymph Node Metastasis in Two or Fewer Nodes in Breast Cancer. J Clin Med Res 2015;7:620-6. [Crossref] [PubMed]
- Zhou Y, Huang X, Mao F, et al. Predictors of nonsentinel lymph node metastasis in patients with breast cancer with metastasis in the sentinel node. Medicine (Baltimore) 2019;98:e13916. [Crossref] [PubMed]
- Zheng J, Cai S, Song H, et al. Positive non-sentinel axillary lymph nodes in breast cancer with 1-2 sentinel lymph node metastases. Medicine (Baltimore) 2018;97:e13015. [Crossref] [PubMed]
- Guo F. Analysis of factors related to axillary non sentinel lymph node metastasis in breast cancer patients with positive sentinel lymph node. Chinese Journal of Practical Medicine 2019;42-5.
- Kim GR, Choi JS, Han BK, et al. Preoperative Axillary US in Early-Stage Breast Cancer: Potential to Prevent Unnecessary Axillary Lymph Node Dissection. Radiology 2018;288:55-63. [Crossref] [PubMed]
- Chen YY, Huang WJ, Xu QH, et al. Value of traditional ultrasound, color doppler and ultrasound elastography for prediction axillary lymph node metastasis of breast cancer. Journal of Molecular Imaging 2019;42:14-7.
- Qiu SQ, Zeng HC, Zhang F, et al. A nomogram to predict the probability of axillary lymph node metastasis in early breast cancer patients with positive axillary ultrasound. Sci Rep 2016;6:21196. [Crossref] [PubMed]
- Xiong J, Zuo W, Wu Y, et al. Ultrasonography and clinicopathological features of breast cancer in predicting axillary lymph node metastases. BMC Cancer 2022;22:1155. [Crossref] [PubMed]
- Cao YM, Liu M, Zhou B, et al. Comparative validation of MSKCC and SOC models for predicting non-sentinel lymph node metastasis in Chinese breast cancer patients. Chinese Journal of Clinical Oncology 2014;41:508-12.
- Liu M, Wang S, Pan L, et al. A new model for predicting non-sentinel lymph node status in Chinese sentinel lymph node positive breast cancer patients. PLoS One 2014;9:e104117. [Crossref] [PubMed]
- Greiner M, Pfeiffer D, Smith RD. Principles and practical application of the receiver-operating characteristic analysis for diagnostic tests. Prev Vet Med 2000;45:23-41. [Crossref] [PubMed]
- Sanjuán A, Escaramís G, Vidal-Sicart S, et al. Predicting non-sentinel lymph node status in breast cancer patients with sentinel lymph node involvement: evaluation of two scoring systems. Breast J 2010;16:134-40. [Crossref] [PubMed]
- Tan EY, Ho B, Chen JJ, et al. Predictors of nonsentinel nodal involvement to aid intraoperative decision making in breast cancer patients with positive sentinel lymph nodes. ISRN Oncol 2011;2011:539503. [Crossref] [PubMed]
- Poirier E, Sideris L, Dubé P, et al. Analysis of clinical applicability of the breast cancer nomogram for positive sentinel lymph node: the canadian experience. Ann Surg Oncol 2008;15:2562-7. [Crossref] [PubMed]
(English Language Editor: A. Kassem)