The added value of S-detect in the diagnostic accuracy of breast masses by senior and junior radiologist groups: a systematic review and meta-analysis
Highlight box
Key findings
• The pooled sensitivity, pooled specificity, and the AUC of senior radiologists + S-detect were all higher than that of junior radiologists + S-detect.
What is known and what is new?
• A previous meta-analysis reported that the pooled sensitivity and specificity of S-detect in diagnosing breast cancer were 0.82 and 0.86
• We assessed the value of S-detect combined with the US outcomes from senior and junior radiologists for the diagnosis of breast cancer.
What is the implication, and what should change now?
• S-detect can assist radiologists to diagnose breast cancer and reduce the subjectivity between different radiologists.
Introduction
The incidence and mortality of breast cancer among women worldwide have been increasing in recent years, and in 2020, breast cancer has surpassed lung cancer as the most common cancer worldwide. Breast cancer mortality accounts for 6.9% of all tumor-related deaths (1), which seriously endangers the health of women. Early diagnosis and treatment of breast cancer are key to reducing the mortality rate and improving the survival quality of patients (2,3). Ultrasound (US) is an important imaging tool for breast cancer screening. However, the diagnostic results are easily affected by the operation and experience level of radiologists, and there is a risk of misdiagnosis and missed diagnosis (4-7).
The proposed breast imaging reporting and data system (BI-RADS) has standardized breast US examinations (8), but the influence of inter-observer variability on US diagnostic results still exists, especially in primary hospitals, due to the large number of junior radiologists, the accuracy of BI-RADS classification in the diagnosis of the nature of breast lesions and the final pathological results is low. In recent years, with the rise of artificial intelligence and the rapid development of digital healthcare, the application of computer-aided diagnosis (CAD) in medical imaging has become a research hotspot (9-11). S-detect is a CAD system based on deep learning algorithms grafted onto US instruments, which is based on the BI-RADS interpretation of the benignity and malignancy of lesions, which indicates that the breast lesion is “probably benign” or “probably malignant”. This technique can help to improve diagnostic efficiency and reduce subjective variability and has broad application prospects (12), Some studies have shown that US + S-detect can improve the diagnostic accuracy of junior radiologists more than senior radiologists, but the results are inconsistent in various studies (13,14). Because the results of previous studies were variable, there are no relevant meta-analyses examining the results of this technique in combination with senior and junior radiologists. The present meta-analysis included several domestic and international articles for quantitative synthesis, aiming to evaluate the usefulness of applying S-detect to breast US and examining whether there are differences between senior and junior radiologists, exploring how the S-detect technique can help radiologists of different levels of seniority in the clinical setting. We present the following article in accordance with the PRISMA-DTA reporting checklist (available at https://gs.amegroups.com/article/view/10.21037/gs-22-643/rc).
Methods
Data sources and search strategy
On August 20, 2022, we searched the PubMed, Cochrane Library, Embase, Web of Science, Wanfang databases, China Biology Medicine disc, China National Knowledge Infrastructure (CNKI), and VIP database for studies evaluating the accuracy of US + S-detect in breast cancer. Taking PubMed as an example, the search strategy used the following formula: “(ultrasound) AND (S-detect) AND (breast)”. Similar search formulas were used for the Cochrane Library, Embase, Web of Science, Wanfang databases, China Biology Medicine disc, China National Knowledge Infrastructure (CNKI), and VIP database.
Eligibility criteria
We relied on the population, intervention, comparison, outcome and study design principle (PICOS) to define study eligibility.
Population: patients with S-detect diagnosis of breast masses were included.
Intervention: S-detect diagnosis combined with the diagnosis of senior and junior radiologists.
Comparison: the gold diagnosis of the breast masses (final histopathological examination)
Outcome: diagnostic accuracy of breast lesions by S-detect combined with senior and junior radiologists (sensitivity, specificity, accuracy).
Study design: any type of study design, such as retrospective, prospective, or case-control, were eligible for inclusion provided the study assessed the diagnostic accuracy of breast lesions by S-detect combined with senior and junior radiologists.
Exclusion criteria: (I) case reports, conference proceedings, reviews, animal studies, etc., for which the original data were unavailable or lacking; (II) articles with small sample sizes (<20 cases); (III) articles written using the same data; and (IV) repeated articles from different databases.
Literature screening and data extraction
Two researchers (Chen PJ and Tong JH) independently completed the literature screening and data extraction. In cases of disagreement and those in which no consensus could be reached after discussion, a third researcher determined the data for inclusion. The following data were extracted from the literature: (I) basic information including the first author, year of article publication, author’s country, reference standard, study design, age of patients, number of patients and lesions, tumor diameter, type of US equipment, BI-RADS edition, radiologist’s seniority level, BI-RADS diagnostic threshold, and method of US + S-detection; and (II) 2×2 table information including true positive (TP), false positive (FP), false negative (FN), and true negative (TN). If there are multiple sets of data in one article, take the average. If the 2×2 table information was not directly obtained from the original literature, it could be calculated based on the sample size, sensitivity, and specificity provided in the article.
Assessment of study quality
The quality of the included studies was evaluated using the quality assessment of diagnostic accuracy studies-2 (QUADAS-2) scale after a thorough reading of all selected articles (15), and each of the 14 items was rated according to the actual content of each original study. Determine “yes”, “no”, “uncertain” according to the relevant signature questions included in each part, the risk of bias and applicability of the scale were assessed as “high”, “low”, or “unclear”. If the answer to the signature question is “yes”, the risk of bias is judged to be low. “No” is judged as a high risk of bias. High quality articles are randomized controlled trials (RCTs), high-quality case control or cohort studies with a very low risk of confounding or bias. The quality evaluation chart of the included literature was obtained using Review Manager 5.4 software (https://training.cochrane.org/online-learning/core-software-cochrane-reviews/revman). Quality evaluation was performed independently by two researchers; in cases of inconsistency and those in which no consensus could be reached after discussion, a third researcher made the final decision.
Statistical analysis of data
We inputted TP, FP, FN, and TN into the STATASE 15.1 software (StataCorp, College Station TX, USA) and calculated the estimated pooled sensitivity and specificity of US + S-detect for breast cancer by senior and junior radiologists, respectively. The area under the curve (AUC) was used to evaluate the diagnostic value of US + S-detect for breast cancer by radiologists of different seniority levels. The closer the AUC was to 1, the higher the diagnostic accuracy; however, the closer the AUC was to 0.5, the lower the diagnostic accuracy. Z-test was conducted to compare sensitivity, specificity, and AUC between senior and junior radiologists, and a P value ≤0.05 was considered to indicate statistical significance.
Heterogeneity analysis
Spearman’s correlation coefficient was calculated using Meta-Disc 1.4 software (https://meta-disc.software.informer.com/1.4/) to test for a threshold effect and assess whether there was heterogeneity due to a threshold effect. A threshold effect was considered to exist when Spearman’s correlation coefficient was >0 and P<0.05.
The Cochrane Q test was applied to obtain P values to quantify the heterogeneity between original studies. When the I2 value was <50%, heterogeneity was considered insignificant, and the data were directly combined for analysis. Meanwhile, when the I2 value was >50%, heterogeneity was considered significant, and a random-effects model was used to merge the data and explore the potential factors contributing to heterogeneity (16,17).
Subgroup analysis and sensitivity analysis
Subgroup analysis was performed to search for factors that might contribute to heterogeneity. The original studies were divided into two subgroups based on the possible factors, when the heterogeneity of a group decreased to <50% in subgroup analysis, it was considered that the source of heterogeneity was found through subgroup analysis. In this study, reference standard (one standard vs. two standards), number of included lesions (≥200 vs. <200), BI-RADS diagnostic threshold (BI-RADS 4a and 4b vs. BI-RADS 3 and others), the method of US + S-detect (objective binding vs. based on radiologist subjective binding or others), the methodological quality of the study (high-quality vs. acceptable-quality) were used as independent variables to delineate the subgroups, respectively.
We performed a sensitivity analysis to further explore the sources of heterogeneity and assess the reliability of the combined effect size results, and determine if there were original studies that had a large effect on heterogeneity.
Clinical application analysis
Fagan plots were created, pre-test probabilities were set, and post-test probabilities were calculated using the combined positive likelihood ratios to analyze the clinical value of using US + S-detect to diagnose breast cancer.
Results
Literature screening process
According to the formulated search strategy, eight databases were searched sequentially, and a total of 177 articles were initially obtained. After importing these articles into Endnote software (Clarivate, Philadelphia, PA, USA), 72 identical articles were excluded, and 46 articles that were deemed inconsistent based on their titles and abstracts were further checked. The remaining relevant articles were read and the inclusion and exclusion criteria were strictly implemented. Finally, a total of 19 diagnostic research articles were included in this meta-analysis (Figure 1).
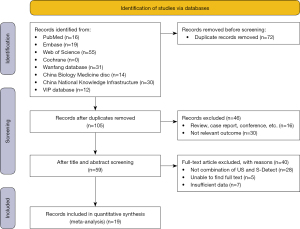
Basic characteristics of the included literature
This meta-analysis included 19 original studies (18-36), including eight English articles (19-26) and eleven Chinese studies (18,27-36). We extracted and summarized the basic characteristics of the included literature. Four included studies were prospective (20,21,23,26), seven were retrospective (18,19,25,29-31,33), and the remaining articles were not reported. Also, a total of 3,349 patients with 3,895 lesions were included. The average lesion diameter of the included patients was 1.10–1.93 cm, and the average age of the patients was 42.6–51.0 years. Eleven articles evaluated the diagnostic performance of US + S-detect for breast masses by both senior and junior radiologists’, six articles evaluated only senior radiologists, and two article evaluated only junior radiologists. The basic characteristics of the included literature are shown in Table 1.
Table 1
First author | Year | Country | Reference standard | Study design | Age (years) (mean ± SD) | No. of patients | No. of masses (benign/malignant) | Size (cm) | US equipment | BI-RADS edition | Seniority of doctor | BI-RADS threshold | Method of US + S-detect |
---|---|---|---|---|---|---|---|---|---|---|---|---|---|
Boyuan Xing (27) | 2022 | China | Biopsy, excision | NA | 44.0±11.5 | 151 | 190 (136/54) | 1.2±0.4 | RS80A | 5th | Senior | BI-RADS 4a | S-detect malignant, BI-RADS upgrades, S-detect benign, BI-RADS degrades |
Xin-Yi Wang (22) | 2021 | China | Biopsy, excision | NA | 50.8±15.5 | 167 | 173 (95/78) | 1.6±0.9 | RS80A | 5th | Senior and junior | BI-RADS 3 | S-detect benign, BI-RADS 4 and 5 degrade, if not, the original BI-RADS maintain |
Yongping Liang (26) | 2020 | China | Excision | Prospective | 43.11±12.55 | 261 | 398 (248/150) | 1.92±1.26 | RS80A | 5th | Senior and junior | BI-RADS 4a | One side of S-detect malignant, BI-RADS upgrades, both sides of S-detect benign, BI-RADS degrades |
Min Young Kim (21) | 2021 | Korea | Biopsy | Prospective | 46±10 | 146 | 156 (146/10) | 1.1±0.5 | RS85 | NA | Senior and junior | BI-RADS 3 | Subjective judgment |
Chenyang Zhao (25) | 2020 | China | Biopsy, excision | Retrospective | 45.7 | 195 | 195 (113/82) | NA | RS85 | NA | Junior | BI-RADS 3 | S-detect benign, BI-RADS 4a, indicates benign, S-detect malignant, BI-RADS 3 indicates benign; others maintain original BI-RADS of doctor |
Qun Xia (24) | 2021 | China | Biopsy | NA | 50.9±13.9 | 40 | 40 (16/24) | NA | RS80A | 5th | Senior and junior | BI-RADS 4a | Subjective judgment |
Qi Wei (23) | 2022 | China | Biopsy, excision | Prospective | 43±12 | 248 | 266 (197/69) | 1.48±0.92 | RS80A | 5th | Senior and junior | Clinical experience | Subjective judgment |
Eun Cho (19) | 2018 | Korea | Biopsy, excision | Retrospective | 48.5±12.2 | 116 | 119 (65/54) | 1.69±1.07 | RS80A | 5th | Senior and junior | BI-RADS 3 | Subjective judgment |
Fang He (18) | 2018 | China | Excision | Retrospective | 42.6±6.2 | 42 | 54 (30/24) | 1.423±0.447 | RS80A | NA | Senior | BI-RADS 4b | S-detect benign, BI-RADS ≥4b indicates malignant, S-detect malignant, BI-RADS 3 indicates benign |
Ji-Hye Choi (20) | 2018 | Korea | Biopsy, 2 years of follow-up | Prospective | 49.5±11.8 | 200 | 200 (188/12) | 1.2±0.8 | RS80A | NA | Senior and junior | BI-RADS 3 | Subjective, conjunctive, disjunctive |
Wenjun Xu (28) | 2020 | China | Biopsy, excision | NA | 46.15±12.61 | 136 | 145 (87/58) | 1.71±0.78 | RS80A | 5th | Senior | BI-RADS 4a | S-detect malignant, BI-RADS upgrades, S-detect benign, BI-RADS degrades |
Hong Yan (33) | 2020 | China | Excision | Retrospective | 43.4±12.2 | 453 | 581 (411/170) | 1.57±0.85 | RS80A | 5th | Junior | BI-RADS 4a | Subjective judgment |
Jiazhen Pan (32) | 2021 | China | Excision | NA | 46.64±13.88 | 169 | 175 (87/88) | 1.87±0.93 | RS80A | NA | Senior and junior | BI-RADS 4a | S-detect malignant, BI-RADS upgrades, S-detect benign, BI-RADS degrades |
Xue Ge (35) | 2020 | China | Biopsy, excision | NA | 51±15 | 168 | 168 (152/16) | NA | SW80A | NA | Senior and junior | BI-RADS 4b | Either S-detect and BI-RADS method is malignant, then malignant, both are benign, then benign |
Xiang LI (36) | 2019 | China | Biopsy, excision | NA | 44.02±12.13 | 435 | 563 (403/160) | 1.93±0.93 | RS80A | 5th | Senior and junior | BI-RADS 4a | S-detect malignant, BI-RADS upgrades, S-detect benign, BI-RADS degrades |
Yanyan Ge (34) | 2020 | China | Biopsy, excision | NA | 44.2±12.0 | 88 | 98 (56/42) | 1.77±1.14 | RS80A | NA | Senior | BI-RADS 4a | Either S-detect and BI-RADS method is malignant, then malignant, both are benign, then benign |
Feng Zhao (30) | 2021 | China | Biopsy, excision | Retrospective | 44.2±12.0 | 120 | 120 (70/50) | 1.25±0.53 | RS80A | 5th | Senior | BI-RADS 4a | Subjective, conjunctive, disjunctive |
Jinhong Wang (29) | 2019 | China | Excision | Retrospective | 46.02±12.53 | 79 | 92 (59/33) | 1.73±0.88 | RS80A | NA | Senior and junior | BI-RADS 4a | S-detect malignant, BI-RADS upgrades, S-detect benign, BI-RADS degrades |
Lan Shen (31) | 2022 | China | Excision | Retrospective | 42.69±12.34 | 135 | 162 (107/55) | 1.87±0.51 | RS80A | 5th | Senior | NA | Either S-detect and BI-RADS method is malignant, then malignant, both are benign, then benign |
US, ultrasound; BI-RADS, breast imaging reporting and data system; NA, not available.
Methodologic quality evaluation
Review Manager 5.4 software (https://training.cochrane.org/online-learning/core-software-cochrane-reviews/revman) was used to evaluate the quality of the included original studies according to the QUADAS-2 scale. The risk of bias was primarily attributed to patient selection and flow and timing. The risk of bias from the index test was low, as was the risk of bias from the reference standard. Figure 2 displays the quality evaluation of the included literature.
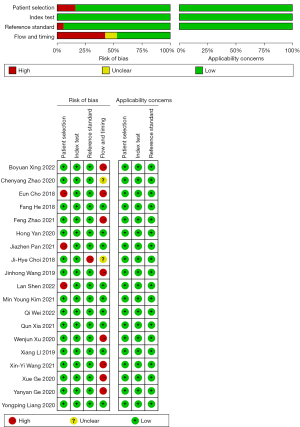
Threshold effect
The 2×2 table data (TP, FP, FN, TN) from nine studies of US + S-detect in the senior radiologist group were imported into the Meta Disc 1.4 statistical software for analysis, yielding a Spearman correlation coefficient of −0.213 (P=0.411). The Spearman correlation coefficient from seven studies of US + S-detect in the junior radiologist group was 0.280 (P=0.379), indicating that there was no threshold effect in either group.
Diagnostic performance of the combination of S-detect with senior radiologists
Seventeen of the studies (involving 3,119 lesions) reported on the diagnostic performance of the combination of S-detect with senior radiologists in differentiating benign from malignant breast masses. Combining S-detect with senior radiologists, the pooled sensitivity was 0.93 [95% confidence interval (CI): 0.89–0.95, I2=64.74%] and the pooled specificity was 0.86 (95% CI: 0.80–0.90, I2=86.11%). Figure 3A shows the Forest plot of the sensitivity and specificity. The AUC of the summary receiver operating characteristic (SROC) curve was 0.96 (95% CI: 0.93–0.97) (Figure 3B).
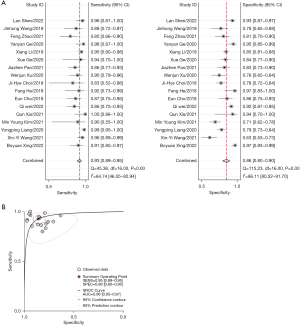
Diagnostic performance of the combination of S-detect with junior radiologists
Twelve of the studies (involving 2,953 lesions) reported on the performance of the combination of S-detect with junior radiologists in diagnosing breast cancer. By combining S-detect with junior radiologists, the pooled sensitivity was 0.89 (95% CI: 0.83–0.93, I2=74.48%), the pooled specificity was 0.79 (95% CI: 0.72–0.84, I2=92.94%) (Figure 4A), and the AUC of the SROC curve was 0.91 (95% CI: 0.88–0.93) (Figure 4B).
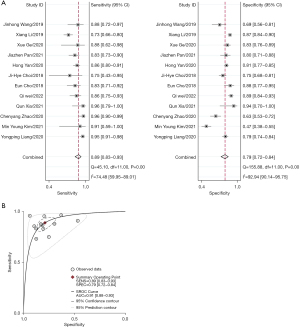
Comparison of diagnostic accuracy between the combination of S-detect with senior radiologists and the combination of S-detect with junior radiologists
Combining S-detect with senior radiologists, the pooled sensitivity, pooled specificity, and the AUC in differentiating benign from malignant breast masses were 0.93, 0.86 and 0.96. The pooled sensitivity, pooled specificity, and the AUC of junior radiologists were 0.89, 0.79 and 0.91. The pooled sensitivity, pooled specificity, and the AUC of senior radiologists plus S-detect were all higher than that of junior radiologists plus S-detect. There is significant difference in the AUC (P<0.01), however, there were without significant difference in the pooled sensitivity (P=0.188) and pooled specificity (P=0.086).
Heterogeneity detection
Subgroup analysis
To identify the potential factors contributing to heterogeneity, the original studies were divided into subgroups according to reference standard, number of included lesions, BI-RADS diagnostic threshold, the method of US + S-detect and the methodological quality of the study for subgroup analysis of the included literature in the senior and junior radiologist groups, respectively. The subgroup analysis results are shown in Table 2 and Table 3.
Table 2
Covariate/subgroup | Studies, n | Sensitivity (95% CI) | I2 | Specificity (95% CI) | I2 |
---|---|---|---|---|---|
Reference test | |||||
One method | 7 | 0.95 (0.89–0.98) | 73.21 | 0.85 (0.76–0.91) | 84.51 |
Two methods | 10 | 0.91 (0.87–0.94) | 58.69 | 0.86 (0.79–0.91) | 88.00 |
No. of lesion | |||||
≥200 | 4 | 0.95 (0.89–0.98) | 83.24 | 0.84 (0.78–0.89) | 83.63 |
<200 | 13 | 0.92 (0.88–0.94) | 52.94 | 0.87 (0.80–0.91) | 88.93 |
BI-RADS diagnostic cut-offs | |||||
BI-RADS 4a and 4b | 11 | 0.93 (0.89–0.96) | 73.46 | 0.87 (0.81–0.91) | 76.90 |
BI-RADS 3 and others | 6 | 0.92 (0.86–0.95) | 33.37 | 0.83 (0.72–0.90) | 90.35 |
US combined with the S-detect method | |||||
Objective | 12 | 0.94 (0.91–0.97) | 66.63 | 0.87 (0.80–0.92) | 87.34 |
Subjective and others | 5 | 0.84 (0.78–0.89) | 0 | 1.83 (0.75–0.89) | 86.40 |
The methodological quality of the study | |||||
High-quality | 6 | 0.96 (0.90–0.98) | 74.08 | 0.85 (0.77–0.90) | 88.11 |
Acceptable-quality | 11 | 0.91 (0.87–0.94) | 45.62 | 0.85 (0.78–0.90) | 85.09 |
US, ultrasound; BI-RADS, breast imaging reporting and data system.
Table 3
Covariate/subgroup | Studies, n | Sensitivity (95% CI) | I2 | Specificity (95% CI) | I2 |
---|---|---|---|---|---|
Reference test | |||||
One method | 6 | 0.90 (0.84–0.94) | 57.31 | 0.76 (0.63–0.85) | 93.86 |
Two methods | 6 | 0.86 (0.77–0.92) | 76.41 | 0.82 (0.74–0.88) | 90.42 |
No. of lesion | |||||
≥200 | 5 | 0.87 (0.78–0.92) | 86.80 | 0.83 (0.78–0.87) | 82.43 |
<200 | 7 | 0.90 (0.83–0.94) | 44.67 | 0.75 (0.63–0.84) | 92.87 |
BI-RADS diagnostic cut-offs | |||||
BI-RADS 4a and 4b | 7 | 0.88 (0.81–0.92) | 82.17 | 0.69 (0.56–0.82) | 65.06 |
BI-RADS 3 and others | 5 | 0.91 (0.83–0.95) | 54.43 | 0.75 (0.58–0.87) | 95.74 |
US combined with S-detect method | |||||
Objective | 7 | 0.90 (0.82–0.95) | 86.31 | 0.78 (0.67–0.86) | 95.99 |
Subjective and others | 5 | 0.86 (0.80–0.90) | 0 | 1.78 (0.64–0.88) | 96.14 |
The methodological quality of the study | |||||
High-quality | 7 | 0.91 (0.83–0.96) | 85.80 | 0.90 (0.82–0.95) | 95.99 |
Acceptable-quality | 5 | 0.84 (0.78–0.88) | 0 | 0.79 (0.74–0.84) | 58.12 |
US, ultrasound; BI-RADS, breast imaging reporting and data system.
In the senior radiologist group, the heterogeneity for sensitivity of the BI-RADS 3 and others group, the subjective and others group, and acceptable-quality group were reduced to 50%, indicating that these three factors were among the sources of heterogeneity of sensitivity in the original study. In the junior radiologist group, the heterogeneity for sensitivity of the No. of lesion <200, subjective and others, and acceptable-quality subgroups were reduced to 50%, indicating that these three factors were a source of heterogeneity of sensitivity in the original study.
Sensitivity analysis
Sensitivity analyses were conducted separately for the senior and junior radiologist groups. After included literature were removed successively, the remaining literature was combined to calculate the value of I2 for sensitivity analysis. and no significant effect of the literature on the results was found, indicating that the results of this meta-analysis are stable.
Clinical value analysis
Fagan diagrams were constructed (Figure 5), assuming a pre-test probability of 50%, and post-test probabilities of 87% and 81% were obtained after calculation for the senior and junior radiologist groups, respectively. This indicated that the application of US + S-detect has a high clinical application for the diagnosis of breast cancer.
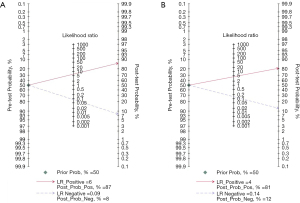
Discussion
US is widely used for the diagnosis of breast lesions, but it is influenced by the subjective factors of the operator. Furthermore, it has been reported that when using the BI-RADS vocabulary for the assessment of breast masses, the specificity of less experienced radiologists is significantly lower than that of experienced radiologists (37). With the development of medical imaging technology, the feasibility of artificial intelligence technology applied to the diagnosis of breast diseases has been reported by several articles. S-detect reduces the influence of the subjective factors of radiologists to a certain extent, making the results more objective (38). In this meta-analysis, we collected domestic and international articles on the combined diagnosis of US + S-detect by senior and junior radiologists to investigate whether the combination of both could improve the discrimination of benign and malignant breast masses.
A previous meta-analysis (39) reported that the pooled sensitivity and specificity of S-detect in diagnosing breast cancer were 0.82 and 0.86, respectively, and could be a complement to conventional US. In our meta-analysis, we selected 19 articles in which US + S-detect were combined to diagnose breast masses; seventeen of these articles evaluated the diagnostic performance of US + S-detect for breast cancer by senior radiologists and twelve articles evaluated the diagnostic performance of US + S-detect for breast cancer by junior radiologists. The diagnostic indexes of US + S-detect by senior and junior radiologists were respectively calculated and quantitatively pooled by meta-analysis. The literature included in both groups had no threshold effect, but the heterogeneity was significant, so a random-effects model was used for the combined analysis of data. The results showed that in the senior radiologist group, the pooled sensitivity, specificity, and AUC of US + S-detect were 0.93 (95% CI: 0.89–0.95), 0.86 (95% CI: 0.80–0.90), and 0.96 (95% CI: 0.93–0.97). In the junior radiologist group, pooled sensitivity, pooled specificity, and AUC of US + S-detect were 0.89 (95% CI: 0.83–0.93), 0.79 (95% CI: 0.72–0.84) and 0.91 (95% CI: 0.88–0.93).
It was suggested that the diagnostic performance was high in both groups; pooled sensitivity, the pooled specificity and AUC were all higher in the senior radiologist group than in the junior radiologist group, and the AUC value was significantly different between the two groups (P<0.01). Some articles showed that the improvement was more significant in the junior radiologist group compared to US (19-21,23). Meanwhile, we analyzed the clinical application value of US + S-detect by plotting Fagan diagrams, setting the pre-test probability at 50% and the post-test probability at 87% and 81% for the senior and junior groups, respectively. The pre-test probability refers to the prevalence rate estimated by the clinician based on the patient’s medical history, signs, and personal experience before the patient undergoes this test. The more the post-test probability increases compared to the pre-test probability, the more valuable the test is in terms of clinical application. Therefore, the US + S-detect is of high clinical value for the diagnosis of breast cancer by both senior and junior radiologists.
The malignancy rate of breast masses classified as BI-RADS 4a is approximately 2–10%, and most category 4a breast masses, although benign, may be subject to unnecessary biopsy (40,41). A previous meta-analysis by Park et al. (42) reported that downgrading of BI-RADS category 4a breast masses to BI-RADS category 3 when US elastography was combined with US for diagnosis reduced unnecessary biopsies in 41.1% of breast category 4a nodules. Combining S-detect can also reduce the biopsy rate by downgrading BI-RADS 4a masses. Combining S-detect to diagnose breast masses reduced the rate of unnecessary biopsies (22,23,25), but downgrading can also result in false-negative results for malignant nodes, so further training in S-detect and the empirical judgment of radiologists is also important.
Since the results of the heterogeneity test showed that the I2 values of sensitivity and specificity were >50% for both the senior and junior radiologist groups, indicating a pronounced heterogeneity between the original studies, the causes of heterogeneity need to be explored. The results of the subgroup analysis to determine the factors that may lead to heterogeneity. It showed that the heterogeneity for sensitivity of the BI-RADS diagnostic cut-offs, US combined with the S-detect method, and the methodological quality of the study may lead to the sources of heterogeneity of sensitivity in the senior radiologist groups. In the junior radiologist group, The No. of lesion, US combined with the S-detect method, and the methodological quality of the study indicated that these three factors may be a source of heterogeneity of sensitivity in the original study. The sources of heterogeneity were further explored by sensitivity analysis, and no significant effect of the literature on the results was observed, indicating good stability of the meta-analysis.
Limitations of this meta-analysis: (I) the study populations of most of the original studies were Chinese patients, leading to the possibility of geographical bias, and thus, more high-quality studies from other countries should be included in future studies to further validate the reliability of the diagnostic results. (II) There was a high degree of heterogeneity in the included literature, and some of the original studies included cases from a retrospective study method; therefore, more high-quality prospective multicenter studies are needed to further explore the diagnostic efficacy of US + S-detect for breast cancer in-depth.
Conclusions
In this study, we collected diagnostic studies from domestic and foreign countries for meta-analysis to evaluate the diagnostic performance of US + S-detect for breast cancer by radiologists of different seniority levels. The combined results showed that the sensitivity and the AUC were all high in the senior and junior radiologist groups, which have a high clinical application value. However, the results of this study are somewhat heterogeneous, and thus, it is still necessary to collect more articles and accumulate a larger sample size for validation.
Acknowledgments
Funding: This work was supported by the Agriculture and Social development plan of Hangzhou (grant No. 20190101A09).
Footnote
Reporting Checklist: The authors have completed the PRISMA-DTA reporting checklist. Available at https://gs.amegroups.com/article/view/10.21037/gs-22-643/rc
Conflicts of Interest: All authors have completed the ICMJE uniform disclosure form (available at https://gs.amegroups.com/article/view/10.21037/gs-22-643/coif). The authors have no conflicts of interest to declare.
Ethical Statement: The authors are accountable for all aspects of the work in ensuring that questions related to the accuracy or integrity of any part of the work are appropriately investigated and resolved.
Open Access Statement: This is an Open Access article distributed in accordance with the Creative Commons Attribution-NonCommercial-NoDerivs 4.0 International License (CC BY-NC-ND 4.0), which permits the non-commercial replication and distribution of the article with the strict proviso that no changes or edits are made and the original work is properly cited (including links to both the formal publication through the relevant DOI and the license). See: https://creativecommons.org/licenses/by-nc-nd/4.0/.
References
- Sung H, Ferlay J, Siegel RL, et al. Global Cancer Statistics 2020: GLOBOCAN Estimates of Incidence and Mortality Worldwide for 36 Cancers in 185 Countries. CA Cancer J Clin 2021;71:209-49. [Crossref] [PubMed]
- O'Mahony M, Comber H, Fitzgerald T, et al. Interventions for raising breast cancer awareness in women. Cochrane Database Syst Rev 2017;2:CD011396. [Crossref] [PubMed]
- Akram M, Iqbal M, Daniyal M, et al. Awareness and current knowledge of breast cancer. Biol Res 2017;50:33. [Crossref] [PubMed]
- Guo R, Lu G, Qin B, et al. Ultrasound Imaging Technologies for Breast Cancer Detection and Management: A Review. Ultrasound Med Biol 2018;44:37-70. [Crossref] [PubMed]
- Funke M. Diagnostic imaging of breast cancer: An update. Radiologe 2016;56:921-38. [Crossref] [PubMed]
- Xue S, Zhao Q, Tai M, et al. Correlation between Breast Ultrasound Microcalcification and the Prognosis of Breast Cancer. J Healthc Eng 2021;2021:6835963. [Crossref] [PubMed]
- Bhatt AA, Whaley DH, Lee CU. Ultrasound-Guided Breast Biopsies: Basic and New Techniques. J Ultrasound Med 2021;40:1427-43. [Crossref] [PubMed]
- Spak DA, Plaxco JS, Santiago L, et al. BI-RADS® fifth edition: A summary of changes. Diagn Interv Imaging 2017;98:179-90.
- Cho HC, Sun S, Min Hyun C, et al. Automated ultrasound assessment of amniotic fluid index using deep learning. Med Image Anal 2021;69:101951. [Crossref] [PubMed]
- Zhao Z, Yang C, Wang Q, et al. A deep learning-based method for detecting and classifying the ultrasound images of suspicious thyroid nodules. Med Phys 2021;48:7959-70. [Crossref] [PubMed]
- Jiang M, Li CL, Luo XM, et al. Ultrasound-based deep learning radiomics in the assessment of pathological complete response to neoadjuvant chemotherapy in locally advanced breast cancer. Eur J Cancer 2021;147:95-105. [Crossref] [PubMed]
- Kim K, Song MK, Kim EK, et al. Clinical application of S-Detect to breast masses on ultrasonography: a study evaluating the diagnostic performance and agreement with a dedicated breast radiologist. Ultrasonography 2017;36:3-9. [Crossref] [PubMed]
- Park HJ, Kim SM, La Yun B, et al. A computer-aided diagnosis system using artificial intelligence for the diagnosis and characterization of breast masses on ultrasound: Added value for the inexperienced breast radiologist. Medicine (Baltimore) 2019;98:e14146. [Crossref] [PubMed]
- Wu JY, Zhao ZZ, Zhang WY, et al. Computer-Aided Diagnosis of Solid Breast Lesions With Ultrasound: Factors Associated With False-negative and False-positive Results. J Ultrasound Med 2019;38:3193-202. [Crossref] [PubMed]
- Whiting PF, Rutjes AW, Westwood ME, et al. QUADAS-2: a revised tool for the quality assessment of diagnostic accuracy studies. Ann Intern Med 2011;155:529-36. [Crossref] [PubMed]
- Higgins JP, Thompson SG, Deeks JJ, et al. Measuring inconsistency in meta-analyses. BMJ 2003;327:557-60. [Crossref] [PubMed]
- Zhou Y, Dendukuri N. Statistics for quantifying heterogeneity in univariate and bivariate meta-analyses of binary data: the case of meta-analyses of diagnostic accuracy. Stat Med 2014;33:2701-17. [Crossref] [PubMed]
- He F, Xiao J, Wen H, et al. S-detect computer-aided ultrasound in differential diagnosis of benign malignant mass-like breast lesions with maximun diameter ≤2cm. Chinese Journal of Medical Imaging Technology 2018;34:1207-10.
- Cho E, Kim EK, Song MK, et al. Application of Computer-Aided Diagnosis on Breast Ultrasonography: Evaluation of Diagnostic Performances and Agreement of Radiologists According to Different Levels of Experience. J Ultrasound Med 2018;37:209-16. [Crossref] [PubMed]
- Choi JH, Kang BJ, Baek JE, et al. Application of computer-aided diagnosis in breast ultrasound interpretation: improvements in diagnostic performance according to reader experience. Ultrasonography 2018;37:217-25. [Crossref] [PubMed]
- Kim MY, Kim SY, Kim YS, et al. Added value of deep learning-based computer-aided diagnosis and shear wave elastography to b-mode ultrasound for evaluation of breast masses detected by screening ultrasound. Medicine (Baltimore) 2021;100:e26823. [Crossref] [PubMed]
- Wang XY, Cui LG, Feng J, et al. Artificial intelligence for breast ultrasound: An adjunct tool to reduce excessive lesion biopsy. Eur J Radiol 2021;138:109624. [Crossref] [PubMed]
- Wei Q, Zeng SE, Wang LP, et al. The Added Value of a Computer-Aided Diagnosis System in Differential Diagnosis of Breast Lesions by Radiologists With Different Experience. J Ultrasound Med 2022;41:1355-63. [Crossref] [PubMed]
- Xia Q, Cheng Y, Hu J, et al. Differential diagnosis of breast cancer assisted by S-Detect artificial intelligence system. Math Biosci Eng 2021;18:3680-9. [Crossref] [PubMed]
- Zhao C, Xiao M, Liu H, et al. Reducing the number of unnecessary biopsies of US-BI-RADS 4a lesions through a deep learning method for residents-in-training: a cross-sectional study. BMJ Open 2020;10:e035757. [Crossref] [PubMed]
- Yongping L, Zhou P, Juan Z, et al. Performance of Computer-Aided Diagnosis in Ultrasonography for Detection of Breast Lesions Less and More Than 2 cm: Prospective Comparative Study. JMIR Med Inform 2020;8:e16334. [Crossref] [PubMed]
- Xing BY, Dong M, Liu DT, et al. The Diagnostic Value of Ultrasound S-Detect Technology for Small Breast Nodules with Diameter ≤2cm. Chinese J Ultrasound Med 2022;38:626-9.
- Xu WJ. The Value of S-Detect Combined with Strain Elastography in the Diagnosis of Breast Tumor (master). Shantou University; 2020.
- Wang JH. Diagnostic value of S-Detect technology in solid lesions of the breast (master). Shantou University; 2019.
- Zhao F, Xiao JD, Wen H, et al. Application of computer-aided ultrasonography in assisting radiologists to diagnose early breast cancer. Journal of Molecular Imaging 2021;44:226-31.
- Shen L, Wang Y. The application value of artificial intelligence S-Detect technology combined with elastic imaging in ultrasonic diagnosis of breast lesions. Modern Medical Imagelogy 2022;31.
- Pan JZ, Cha HL, Du LW, et al. The application in evaluation of breast nodules by different combined schemes of S-Detect technique and conventional ultrasound.·Journal of Nanjing Medical University (Natural Science Edition) 2021;41.
- Yan H, Li X, Cheng HF, et al. Clinical application of S-Detect on breast masses on ultrasonography: a study analyzing the influence factors and the combined diagnosis with a radiologist specializing in breast lesions. Journal of China Clinic Medical Imaging 2020;31.
- Ge YY, Wang JH, Hu YB, et al. Application value of conventional ultrasound combined with S-Detect in the diagnosis of benign and malignant breast masses. Chin J Lab D iagn 2020;24.
- Ge X, Du JW, Wang H, et al. Value of S-Desect Technique in Diagnosing Breast Masses Properties of Ultrasound Doctors At Different Levels. Chinese J Ultrasound Med 2020;36.
- Li X, Cheng HF, Yan H, et al. Diagnostic Value of Conventional Ultrasound Combined with S-Detect in Breast Lesions. Chinese J Ultrasound Med 2019;35.
- Lee YJ, Choi SY, Kim KS, et al. Variability in Observer Performance Between Faculty Members and Residents Using Breast Imaging Reporting and Data System (BI-RADS)-Ultrasound, Fifth Edition (2013). Iran J Radiol 2016;13:e28281.
- Zhao C, Xiao M, Ma L, et al. Enhancing Performance of Breast Ultrasound in Opportunistic Screening Women by a Deep Learning-Based System: A Multicenter Prospective Study. Front Oncol 2022;12:804632. [Crossref] [PubMed]
- Li J, Sang T, Yu WH, et al. The value of S-Detect for the differential diagnosis of breast masses on ultrasound: a systematic review and pooled meta-analysis. Med Ultrason 2020;22:211-9. [Crossref] [PubMed]
- Zhao Z, Hou S, Li S, et al. Application of Deep Learning to Reduce the Rate of Malignancy Among BI-RADS 4A Breast Lesions Based on Ultrasonography. Ultrasound Med Biol 2022;48:2267-75. [Crossref] [PubMed]
- Covington MF. Ultrasound Elastography May Better Characterize BI-RADS 3 and BI-RADS 4A Lesions to Decrease False-Positive Breast Biopsy Rates and Enable Earlier Detection of Breast Cancer. J Am Coll Radiol 2022;19:635-6. [Crossref] [PubMed]
- Park SY, Kang BJ. Combination of shear-wave elastography with ultrasonography for detection of breast cancer and reduction of unnecessary biopsies: a systematic review and meta-analysis. Ultrasonography 2021;40:318-32. [Crossref] [PubMed]
(English Language Editor: A. Kassem)