Establishment and verification of a nomogram to predict tumor-specific mortality risk in triple-negative breast cancer: a competing risk model based on the SEER cohort study
Highlight box
Key findings
• We used competing risk models to identify risk factors for cancer-specific mortality (CSM) and constructed a CSM risk prediction nomogram for triple-negative breast cancer (TNBC) patients.
What is known and what is new?
• TNBC is the subtype of breast cancer with the worst prognosis, and traditional survival analysis methods are biased when predicting mortality.
• This study is the first to establish a competing risk model for predicting tumor-specific survival in TNBC patients and validate that the accuracy of this model is satisfactory.
What is the implication, and what should change now?
• This is a retrospective study, and additional clinical validation is required.
Introduction
The incidence of breast cancer is the highest and its mortality rate (15%) ranks the second among all malignancies in women. Breast cancer accounts for about 30% of all new incidence of cancers among females (1-3). Currently, the classification of breast cancer at the molecular level includes luminal A, luminal B, human epidermal growth factor receptor 2 (HER-2) overexpression, and triple-negative breast cancer (TNBC) based on the expression levels of progesterone receptor (PR), HER-2, and estrogen receptor (ER) (4,5). Among them, TNBC accounts for 10–15% of all cases of breast cancer and is clinically defined as breast cancer with negative ER, PR, and HER-2. In comparison to other types of breast cancer, TNBC is characterized by more aggressive development, occurring at a younger age, and local recurrence and distant metastasis at an earlier stage (6-8). According to previous studies, TNBC patients are prone to recurrence and distant metastasis within 2 years after surgery. If there is recurrence and metastasis, the overall survival (OS) is only 13–18 months (9,10). Although recent clinical trials have reported that the emerging programmed cell death protein 1 (PD-1) antibodies, anti-programmed death ligand 1 (PD-L1) antibodies, and other related agents are effective in the treatment of TNBC, they have mainly been used in the maintenance treatment of advanced-stage patients with recurrence or metastasis, and the clinical benefits have been quite limited (11-13). Therefore, identifying patients with high risk at an early stage is of great importance in clinical practice and is crucial for clinicians to make individualized treatment decisions. More aggressive treatment strategies can be used at an early stage, especially for high-risk patients.
Even though there are numerous predictive models for patient prognosis, such as TNM staging, they all are based on traditional survival analysis methods that ignore competing events. As multiple causes of survival exist for patients in clinical practise, such as specific diseases, cardiovascular and cerebrovascular accidents, traffic accidents, etc. There is a competitive relationship between different results (14). The competing events are regarded as censored data in the traditional statistical methods (e.g., Kaplan-Meier and Cox proportional hazards model) used in survival analysis. However, there are biases if competing events exist, and it is possible that results inconsistent with real situations can be generated (15,16). In the observation cohort, if the occurrence of certain known events can influence the possibility of other events or even prevent them from happening, then we can consider that there is a competing risk between the 2 events. For example, death from cerebrovascular accident can influence the outcome of death from a specific disease in drug study (17). To analyze the probability of a specific outcome in the survival data which includes various possible outcome measures, Fine and Gray proposed a statistical method named competing risk model (18). Since then, the competing risk model has been used in medical statistics to analyze survival data involving competing events. In medical practice, a significant proportion of patients die from causes unrelated to breast cancer, resulting in inaccurate survival prediction models. Therefore, we must distinguish the causes of patient death and categorize the causes of death on the line in order to enhance the accuracy of our predictions. Predicting patient cancer-specific mortality (CSM) can more precisely identify patients with a high mortality risk, thereby facilitating clinical decision-making.
For the aforementioned reasons, the Surveillance, Epidemiology, and End Results (SEER) database was applied, which contains large multicenter and high-quality research data, to investigate CSM in TNBC patients using a competing risk model. Independent risk factors for the CSM of TNBC were screened and nomograms were established for predicting the risk of CSM at 1, 3, and 5 years. Model validation also presented that the model had satisfactory accuracy and reliability. This provides certain reference for identifying patients who have high risk and making individualized therapeutic decisions in clinical practice. We present the following article in accordance with the TRIPOD reporting checklist (available at https://gs.amegroups.com/article/view/10.21037/gs-22-650/rc).
Methods
Data sources and screening of cases
As a highly authoritative tumor database worldwide, the SEER database (https://seer.cancer.gov/) was established by the National Cancer Institute in 1973 which collected detailed clinical data on patients’ pathological, therapeutic, and prognostic information (19). Currently, the registry number in the SEER database has increased to 18, covering approximately 34.6% of the entire US population (20). We applied for and obtained permission to use this database and the approval number was 14941-Nov2021. SEER is an open-access database available to the public for research. Case data downloaded from this database was completely anonymous and does not involve personal information of the patients, therefore ethical approval was not required in this study. The study was conducted in accordance with the Declaration of Helsinki (as revised in 2013).
SEER*Stat software (version 8.4.0.1; https://seer.cancer.gov/seerstat/) was applied in extracting the clinicopathological, treatment, and prognostic data of the patients with a pathological diagnosis of TNBC from 2010 to 2015. The extracted data included age, diagnosis year, marital status, race, histological type and grade, site of primary tumor, tumor-node-metastasis (TNM) staging according to the American Joint Committee on Cancer (AJCC) 7th edition, surgical method, radiotherapy, chemotherapy, number of metastasized axillary lymph nodes, bone metastasis, brain metastasis, lung metastasis, liver metastases, tumor size, number of tumors, cause of death, survival status, and survival period. The inclusion criteria were as follows: (I) the year of diagnosis was between 2010 and 2015; (II) patient age at the time of TNBC diagnosis was more than 18 years; (III) the pathological diagnosis was TNBC. The exclusion criteria were as follows: (I) patients with unclear clinicopathological data; (II) cases with a survival period of less than 1 month.
Screening of variables
Marital status was grouped as separated, unmarried, married, and others. Race was grouped as Black, White, and other races (American Indian/Alaskan Native, Asian/Pacific Islander). Tumor site was classified as the upper outer quadrant of the breast (C50.4), inner upper quadrant of the breast (C50.2), outer lower quadrant of the breast (C50.5), inner lower quadrant of the breast (C50.3), breast overlapping lesions (C50.8), breast not otherwise specified (NOS; C50.9), and others. The histological type was grouped as invasive ductal carcinoma (8500/3, 8521/3, 8522/3, 8523/3) and other types. The histological grade of the tumor was classified as grade I (highly differentiated), grade II (moderately differentiated), grade III (poorly differentiated), and grade IV (undifferentiated). T staging based on the AJCC 7th edition was grouped as T1, T2, T3, and T4. N staging based on the AJCC 7th edition was classified as N0, N1, N2, and N3. M staging based on the AJCC 7th edition was classified as M0 and M1. The count of positive axillary lymph nodes was classified as 0, 1–3, 4, and more than 4. Liver, brain, bone, and lung metastases were classified as metastasis and no metastasis. The number of tumors was classified as 1 and more than 1. The survival outcomes were classified as survival, death from TNBC, and death from other reasons.
Establishment and verification of the competitive risk model
The patients were assigned into the training set and testing set at a ratio of 7:3 in a random pattern. The competing risk model was constructed by using the training set, and the external validation of the model was conducted by using the testing set. The cumulative incidence of each event in patients was calculated by the cumulative incidences function (CIF). The Gray test was employed to compare the cumulative mortality between groups. CIF curves were plotted for each potential risk factor. The variables with a statistically significant difference in univariate analysis were subjected to the Fine-Gray semiparametric proportional hazards model to screen the independent prognostic risk factors for CSM. The independent prognostic risk factors for CSM were employed to set up the nomograms for predicting the risk for the CSM of TNBC at 1, 3, and 5 years. To validate the model reliability, the training set and testing set were utilized for internal and external validation, respectively. The area under the ROC curve (AUC) and the concordance index (C-index) were utilized to assess the discrimination of the model and the calibration curve was utilized to evaluate the accuracy of the model.
Calculation of the CSM at different times
To compare the difference in the CSM calculated by competing risk model and conventional survival analysis, the CSM of TNBC at different times was measured by utilizing the competing risk model and conventional survival analysis, respectively. Kaplan-Meier analysis was used in traditional survival analysis. CSM and other causes of mortality (OCM) were analyzed using the competing risk model. CSM differences measured respectively by the 2 methods were compared.
Statistical analysis
Continuous variables were expressed as the mean and standard deviation, and nonparametric U test was used for variance analysis. Enumeration data were analyzed by chi-square test for variance analysis. The CIF was used to calculate the cumulative incidence of every event at 1, 3, and 5 years. Risk factors for CSM and other death causes were analyzed using Gray’s test and Fine-Gray semiparametric proportional hazards model. R (version 4.0.2; The R foundation for Statistical Computing, Vienna, Austria) and SPSS 23.0 (IBM Corp., Armonk, NY, USA) were employed for all the statistical analyses. A P value <0.05 was regarded as statistically significant.
Results
Patient screening
From the SEER database, we extracted data on 39,134 patients who had a pathological diagnosis of TNBC between 2010 and 2015. Based on the inclusion and exclusion criteria, the following cases were excluded: (I) age less than 18 years old (n=1); (II) patients with missing data, including race (n=169), marital status (n=2,117), differentiation grade (n=1,831), TNM stage (n=592), T stage (n=229), N stage (n=121), number of metastasized axillary lymph nodes (n=5,419), bone metastasis (n=100), brain metastasis (n=5), liver metastasis (n=2), lung metastasis (n=11), tumor size (n=71); (III) survival period of less than 1 month (n=36). A total of 28,430 cases of TNBC were finally selected for this study. The flowchart for details of patient screening procedure is presented in Figure 1.
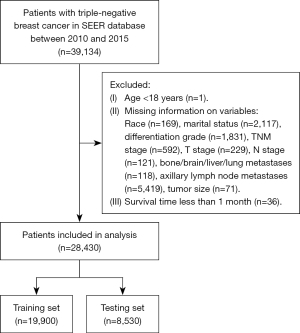
Clinicopathological characteristics of the patients
A total of 28,430 TNBC patients were included and randomly assigned to the training set (n=19,900) and testing set (n=8,530). The clinicopathological information of all the participants are presented in Table 1. The mean age was 58.61±13.60 years, 16,253 (57.17%) were married, and 20,671 (72.71%) were White. There were 10,837 (38.12%) patients with tumors sighted at the upper outer quadrant and 25,680 (90.33%) cases of invasive ductal carcinoma. The counts of the patients with histological grade I, II, III, and IV were 626 (2.2%), 5,043 (17.74%), 22,554 (79.33%), 207 (0.73%), respectively. The counts of patients at TNM stage I and II were 11,335 (39.87%) and 12,559 (44.18%), respectively. The number of patients at T1 and T2 stage were 13,318 (46.84%) and 11,797 (41.49%), respectively. There were 19,329 (67.99%) patients at N0 stage, 6,183 (21.75%) patients at N1 stage, and 27,800 (97.78%) patients at M0 stage. A total of 14,361 (50.51%) patients underwent partial excision, 7,352 (25.86%) underwent total mastectomy, and 6,276 (22.08%) underwent radical mastectomy. A total of 14,293 (50.27%) patients received radiotherapy and 21,272 (74.82%) received chemotherapy. The patients with bone, brain, liver, and lung metastases were 235 (0.83%), 37 (0.13%), 140 (0.49%), and 228 (0.8%), respectively. A total of 21,547 (75.79%) patients had a single tumor. The median time of follow-up was 59 [1–107] months, and 7,014 (24.67%) patients died during follow-up, including 4,801 (68.45%) patients who died from breast cancer and 2,213 (31.55%) patients who died from non-breast cancer events. No difference was found in clinicopathological features between the training and testing sets (all P>0.05).
Table 1
Factors | Training set (n=19,900) | Testing set (n=8,530) | Total patients (n=28,430) | P |
---|---|---|---|---|
Age (years) | 58.52±13.59 | 58.82±13.62 | 58.61±13.60 | 0.086 |
Marriage status, n (%) | 0.728 | |||
Married | 11,398 (57.28) | 4,855 (56.92) | 16,253 (57.17) | |
Divorced | 3,328 (16.72) | 1,424 (16.69) | 4,752 (16.71) | |
Single | 2,454 (12.33) | 1,042 (12.22) | 3,496 (12.3) | |
Others | 2,720 (13.67) | 1,209 (14.17) | 3,929 (13.82) | |
Race, n (%) | 0.382 | |||
White | 14,504 (72.88) | 6,167 (72.3) | 20,671 (72.71) | |
Black | 3,904 (19.62) | 1,734 (20.33) | 5,638 (19.83) | |
Others | 1,492 (7.5) | 629 (7.37) | 2,121 (7.46) | |
Primary site, n (%) | 0.357 | |||
Upper-outer quadrant | 7,542 (37.9) | 3,295 (38.63) | 10,837 (38.12) | |
Upper-inner quadrant | 2,658 (13.36) | 1,163 (13.63) | 3,821 (13.44) | |
Lower-outer quadrant | 1,430 (7.19) | 572 (6.71) | 2,002 (7.04) | |
Lower-inner quadrant | 1,195 (6.01) | 524 (6.14) | 1,719 (6.05) | |
Overlapping lesion | 4,474 (22.48) | 1,903 (22.31) | 6,377 (22.43) | |
Breast, NOS | 1,714 (8.61) | 734 (8.6) | 2,448 (8.61) | |
Others | 887 (4.46) | 339 (3.97) | 1,226 (4.31) | |
Histologic type, n (%) | 0.756 | |||
Infiltrating duct carcinoma | 17,968 (90.29) | 7,712 (90.41) | 25,680 (90.33) | |
Others | 1,932 (9.71) | 818 (9.59) | 2,750 (9.67) | |
Grade, n (%) | 0.25 | |||
Well differentiated | 438 (2.2) | 188 (2.2) | 626 (2.2) | |
Moderately differentiated | 3,538 (17.78) | 1,505 (17.64) | 5,043 (17.74) | |
Poorly differentiated | 15,766 (79.23) | 6,788 (79.58) | 22,554 (79.33) | |
Undifferentiated | 158 (0.79) | 49 (0.57) | 207 (0.73) | |
TNM stage, n (%) | 0.516 | |||
I | 7,961 (40.01) | 3,374 (39.55) | 11,335 (39.87) | |
II | 8,782 (44.13) | 3,777 (44.28) | 12,559 (44.18) | |
III | 2,705 (13.59) | 1,201 (14.08) | 3,906 (13.74) | |
IV | 452 (2.27) | 178 (2.09) | 630 (2.22) | |
T stage, n (%) | 0.312 | |||
T1 | 9,352 (46.99) | 3,966 (46.49) | 13,318 (46.84) | |
T2 | 8,197 (41.19) | 3,600 (42.2) | 11,797 (41.49) | |
T3 | 1,562 (7.85) | 629 (7.37) | 2,191 (7.71) | |
T4 | 789 (3.96) | 335 (3.93) | 1,124 (3.95) | |
N stage, n (%) | 0.246 | |||
N0 | 13,549 (68.09) | 5,780 (67.76) | 19,329 (67.99) | |
N1 | 4,336 (21.79) | 1,847 (21.65) | 6,183 (21.75) | |
N2 | 1,219 (6.13) | 517 (6.06) | 1,736 (6.11) | |
N3 | 796 (4.00) | 386 (4.53) | 1,182 (4.16) | |
M stage, n (%) | 0.333 | |||
M0 | 19,448 (97.73) | 8,352 (97.91) | 27,800 (97.78) | |
M1 | 452 (2.27) | 178 (2.09) | 630 (2.22) | |
Surgery, n (%) | 0.158 | |||
Partial mastectomy | 10,017 (50.34) | 4,344 (50.93) | 14,361 (50.51) | |
Total (simple) mastectomy | 5,173 (25.99) | 2,179 (25.55) | 7,352 (25.86) | |
Radical mastectomy | 4,420 (22.21) | 1,856 (21.76) | 6,276 (22.08) | |
Others | 290 (1.46) | 151 (1.77) | 441 (1.55) | |
Radiation, n (%) | 0.416 | |||
Yes | 10,036 (50.43) | 4,257 (49.91) | 14,293 (50.27) | |
No/unknown | 9,864 (49.57) | 4,273 (50.09) | 14,137 (49.73) | |
Chemotherapy, n (%) | 0.584 | |||
Yes | 14,908 (74.91) | 6,364 (74.61) | 21,272 (74.82) | |
No/unknown | 4,992 (25.09) | 2,166 (25.39) | 7,158 (25.18) | |
LN positive axillary, n (%) | 0.976 | |||
0 | 14,033 (70.52) | 6,004 (70.39) | 20,037 (70.48) | |
1–3 | 4,020 (20.2) | 1,731 (20.29) | 5,751 (20.23) | |
≥4 | 1,847 (9.28) | 795 (9.32) | 2,642 (9.29) | |
Bone metastasis, n (%) | 0.519 | |||
None | 19,731 (99.15) | 8,464 (99.23) | 28,195 (99.17) | |
Yes | 169 (0.85) | 66 (0.77) | 235 (0.83) | |
Brain metastasis, n (%) | 0.971 | |||
None | 19,874 (99.87) | 8,519 (99.87) | 28,393 (99.87) | |
Yes | 26 (0.13) | 11 (0.13) | 37 (0.13) | |
Liver metastasis, n (%) | 0.999 | |||
None | 19,802 (99.51) | 8,488 (99.51) | 28,290 (99.51) | |
Yes | 98 (0.49) | 42 (0.49) | 140 (0.49) | |
Lung metastasis, n (%) | 0.932 | |||
None | 19,741 (99.2) | 8,461 (99.19) | 28,202 (99.2) | |
Yes | 159 (0.8) | 69 (0.81) | 228 (0.8) | |
Total number tumors, n (%) | 0.132 | |||
1 | 15,132 (76.04) | 6,415 (75.21) | 21,547 (75.79) | |
>1 | 4,768 (23.96) | 2,115 (24.79) | 6,883 (24.21) | |
Vital status, n (%) | 0.198 | |||
Alive | 15,015 (75.45) | 6,401 (75.04) | 21,416 (75.33) | |
Breast | 3,373 (16.95) | 1,428 (16.74) | 4,801 (16.89) | |
Others | 1,512 (7.60) | 701 (8.22) | 2,213 (7.78) |
Age is expressed as mean ± standard deviation. TNBC, triple-negative breast cancer; NOS, not otherwise specified; TNM, tumor-node-metastasis; LN, lymph node.
Analysis of risk factors for the CSM of TNBC
The multivariate and univariate competing risk model was employed in analyzing the risk factors for CSM and other causes of mortality (OCM) in patients in the training set. As shown by the univariate competing risk model, the risk factors for CSM included age, marital status, race, primary tumor site, histological grade, N stage, T stage, M stage, TNM stage, surgical method, chemotherapy, count of metastasized axillary lymph nodes, and bone, brain, liver, and lung metastases (Figure S1; Table 1). As shown by the multivariate competing risk model, the independent risk factors for CSM of TNBC included race, age, site of primary tumor, histological grade, TNM stage, T stage, N stage, surgical method, chemotherapy, number of metastasized axillary lymph nodes, brain metastases and liver metastases (Table 2).
Table 2
Factors | Univariate analysis | Multivariate analysis | |||||
---|---|---|---|---|---|---|---|
HR | 95% CI | P | HR | 95% CI | P | ||
Age | 1.002 | 1.000–1.005 | 0.036 | 1.004 | 1.000–1.007 | 0.027 | |
Marriage status | |||||||
Married | Reference | Reference | |||||
Divorced | 1.323 | 1.21–1.448 | <0.001 | 1.088 | 0.984–1.202 | 0.099 | |
Single | 1.367 | 1.237–1.511 | <0.001 | 1.112 | 0.989–1.251 | 0.076 | |
Others | 1.159 | 1.048–1.282 | 0.004 | 1.04 | 0.937–1.154 | 0.046 | |
Race | |||||||
White | Reference | Reference | |||||
Black | 1.309 | 1.209–1.418 | <0.001 | 1.124 | 1.030–1.228 | 0.009 | |
Others | 1.176 | 0.737–0.980 | 0.026 | 0.844 | 0.732–0.975 | 0.021 | |
Primary site | |||||||
Upper-outer quadrant | Reference | Reference | |||||
Upper-inner quadrant | 0.904 | 0.804–1.016 | 0.089 | 1.238 | 1.098–1.396 | <0.001 | |
Lower-outer quadrant | 1.062 | 0.923–1.222 | 0.4 | 1.167 | 1.013–1.345 | 0.032 | |
Lower-inner quadrant | 1 | 0.855–1.168 | 1 | 1.261 | 1.065–1.494 | 0.007 | |
Overlapping lesion | 1.174 | 1.073–1.286 | <0.001 | 1.206 | 1.097–1.325 | <0.001 | |
Breast, NOS | 1.888 | 1.692–2.106 | 0.000 | 1.22 | 1.079–1.379 | 0.002 | |
Others | 1.517 | 1.305–1.764 | <0.001 | 1.149 | 0.980–1.346 | 0.086 | |
Histologic type | |||||||
Infiltrating duct carcinoma | Reference | ||||||
Others | 1.036 | 0.923–1.162 | 0.55 | ||||
Grade | |||||||
Well differentiated | Reference | Reference | |||||
Moderately differentiated | 1.877 | 1.306–2.697 | <0.001 | 1.417 | 1.007–1.994 | 0.045 | |
Poorly differentiated | 2.759 | 1.939–3.926 | <0.001 | 1.738 | 1.246–2.425 | 0.001 | |
Undifferentiated | 2.96 | 1.793–4.886 | <0.001 | 1.639 | 1.004–2.675 | 0.048 | |
TNM stage | |||||||
I | Reference | Reference | |||||
II | 2.951 | 2.658–3.278 | 0.000 | 1.573 | 1.327–1.864 | <0.001 | |
III | 10.011 | 8.990–11.147 | 0.000 | 2.03 | 1.597–2.580 | <0.001 | |
IV | 29.832 | 25.598–34.766 | 0.000 | 4.718 | 3.369–6.609 | 0.000 | |
T stage | |||||||
T1 | Reference | Reference | |||||
T2 | 2.615 | 2.399–2.851 | 0.000 | 1.441 | 1.264–1.642 | <0.001 | |
T3 | 6.152 | 5.520–6.858 | 0.000 | 2.111 | 1.792–2.486 | 0.000 | |
T4 | 10.589 | 9.356–11.983 | 0.000 | 2.263 | 1.876–2.731 | 0.000 | |
N stage | |||||||
N0 | Reference | Reference | |||||
N1 | 3.202 | 2.954–3.470 | 0.000 | 1.22 | 0.972–1.531 | 0.086 | |
N2 | 6.199 | 5.605–6.857 | 0.000 | 0.997 | 0.733–1.358 | 0.99 | |
N3 | 10.321 | 9.239–11.528 | 0.000 | 1.463 | 1.081–1.979 | 0.014 | |
M stage | |||||||
M0 | Reference | ||||||
M1 | 10.144 | 8.933–11.519 | 0.000 | ||||
Surgery | |||||||
Partial mastectomy | Reference | Reference | |||||
Total (simple) mastectomy | 1.474 | 1.346–1.614 | 0.000 | 1.254 | 1.143–1.377 | <0.001 | |
Radical mastectomy | 3.286 | 3.035–3.558 | 0.000 | 1.244 | 1.134–1.365 | <0.001 | |
Others | 5.059 | 4.113–6.223 | 0.000 | 1.969 | 1.550–2.503 | <0.001 | |
Radiation | |||||||
Yes | Reference | ||||||
No/unknown | 1.043 | 0.975–1.115 | 0.22 | ||||
Chemotherapy | |||||||
Yes | Reference | Reference | |||||
No/unknown | 0.819 | 0.755–0.889 | <0.001 | 1.201 | 1.086–1.328 | <0.001 | |
LN positive axillary | |||||||
0 | Reference | Reference | |||||
1–3 | 3.169 | 2.925–3.433 | 0.000 | 1.701 | 1.370–2.111 | <0.001 | |
≥4 | 7.678 | 7.061–8.348 | 0.000 | 3.036 | 2.333–3.949 | <0.001 | |
Bone metastasis | |||||||
None | Reference | Reference | |||||
Yes | 10.49 | 8.580–12.826 | 0.000 | 1.27 | 0.930–1.733 | 0.13 | |
Brain metastasis | |||||||
None | Reference | Reference | |||||
Yes | 15.096 | 8.679–26.257 | 0.000 | 2.295 | 1.345–3.918 | 0.002 | |
Liver metastasis | |||||||
None | Reference | Reference | |||||
Yes | 13.236 | 10.100–17.347 | 0.000 | 1.727 | 1.198–2.491 | 0.003 | |
Lung metastasis | |||||||
None | Reference | Reference | |||||
Yes | 11.252 | 9.055–13.983 | 0.000 | 1.38 | 0.999–1.905 | 0.051 | |
Total number of tumors | |||||||
1 | Reference | ||||||
>1 | 0.929 | 0.858–1.006 | 0.071 |
TNBC, triple-negative breast cancer; NOS, not otherwise specified; TNM, tumor-node-metastasis; LN, lymph node; HR, hazard ratio; CI, confidence interval.
Establishment and evaluation of the competitive risk model
According to the results of multivariate competing risk model in the training set, a prediction nomogram for the CSM risk of TNBC at 1, 3, and 5 years was constructed by using the independent risk factors of CSM (Figure 2). The calibration curves in the testing and training sets confirmed that the nomograms were well consistent with the observed results in actual situations (Figure 3). The data in the training set were applied to conduct the internal validation of the nomogram and the C-index was 0.801 (Table S1). The results of the ROC curve demonstrated that the AUC at 1, 3, and 5 years in the training set were 0.856 [95% confidence interval (CI): 0.839–0.873], 0.81 (95% CI: 0.8–0.819), and 0.782 (95% CI: 0.0772–0.791), respectively (Figure 4A). The AUC of the ROC curve at 1, 3, and 5 years was 0.83 (95% CI: 0.805–0.854), 0.736 (95% CI: 0.720–0.752), and 0.719 (95% CI: 0.703–0.735), respectively (Figure 4B). The data in the testing set were applied in the external validation of the model and the C-index was 0.799 (Table S1). In both the training and testing sets, the model’s specificity and sensitivity are high at 1, 3, and 5 years, indicating that it has a high level of accuracy (Table S2).
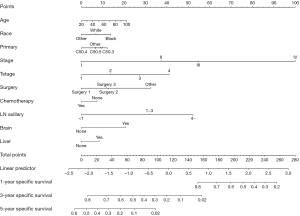
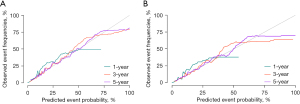
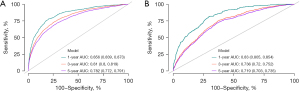
Calculation of CSM at different times
The median time of follow-up of patients was 59 [1–107] months, therefore the CSM of the patients having a follow-up time of 1–8 years (Table 3) was calculated. Compared with the traditional survival analysis, the 1 year CSM calculated by the competing risk model was slightly higher (2.76% in the traditional survival analysis and 2.63% in the competing-risk model). However, the CSM at 2–8 years calculated by the competing risk model was higher. As the time of follow-up increased, the difference gradually widened. In competing risk model analysis, both CSM and OCM elevated as the time of follow-up increased, however, the increase of OCM was greater.
Table 3
Time (year) | Conventional survival analysis (%) | Competing risk analysis (%) | |
---|---|---|---|
CSM | OCM | ||
1 | 2.63 | 2.76 | 1.24 |
2 | 8.68 | 8.00 | 2.64 |
3 | 13.24 | 12.20 | 4.09 |
4 | 16.15 | 14.87 | 5.38 |
5 | 18.09 | 16.62 | 6.75 |
6 | 19.52 | 17.91 | 8.08 |
7 | 20.54 | 18.82 | 9.26 |
8 | 21.08 | 19.30 | 10.73 |
CSM, cancer-specific mortality; OCM, other causes of mortality.
Discussion
Clinically, TNM stage is often used in assessing the prognosis of the patients with breast cancer. The T, N, and M stages of the tumor can influence the prognosis of TNBC and are negatively correlated with patients’ prognosis (21). With the advancement of the TMN stage, the prognosis of patients significantly deteriorates. Therefore, TMN stage has been used by clinicians as a common indicator in assessing the prognosis of the patients with breast cancer and a key reference factor in determining the therapeutic strategy (22,23). However, the prognosis of patients cannot be accurately predicted based only on TNM stage, which was one of the motivations to conduct this study. To date, some researchers have established prediction models for the prognosis of TNBC. However, most of the studies have applied conventional survival analysis methods, without considering the effects of competing events in the occurrence of events of interest, that is, deaths from non-breast cancer reasons were not considered. Tumor-specific mortality in patients is likely to be overstated (24-27). In order to identify and predict CSM risk for TNBC more accurately, we used a competing risk model to investigate 28,430 TNBC cases in the SEER database. The results presented that, after the last follow-up, the mortality rate of the patients was 24.67%, of which 16.89% died of TNBC and 7.78% died of other factors. The CSM calculated by the traditional survival analysis method was higher than the CSM calculated by the competing risk model. In this case, using a competing risk model to investigate the prognosis is more accurate and more consistent with real situations in clinical practice.
In this study, all factors influencing TNBC prognosis which were currently available in the SEER database were included, and the risk factors for CSM were identified by a competing risk model. It was found that marital status, race, age, site of the primary tumor, histological grade, tumor stage, N stage, M stage, T stage, surgical method, chemotherapy, lymph node metastasis, brain metastasis, and liver metastasis were independent prognostic factors for TNBC. A predictive nomogram was then constructed using some important clinical prognostic factors, and the validation of the model also showed that the prediction reliability and accuracy of the model were satisfactory.
Age is remarkably related to the occurrence and development of breast cancer, tumor biological characteristics, and prognosis. Age is often considered an important reference factor in clinical decision making (28-30). Research has found that compared with older patients, the pathological and analytical types of breast cancer and prognosis were worse, and the rates of metastasis and recurrence were higher in younger patients (31-33). Other studies have reported that older patients had higher mortality rates (34), which is because older patients often had more underlying diseases (e.g., heart disease, cerebrovascular disease, diabetes, etc.) compared with younger patients. These underlying diseases could influence the patient’s tolerance and compliance with chemotherapy, therefore more conservative therapeutic strategies were often preferred for older patients (35-37). In the existing studies, traditional methods for prognostic analysis were used. Non-breast cancer mortality was not excluded from the mortality rate, and there was a certain bias in judging the prognosis. Our study illustrated that the age hazard ratio (HR) in the univariate analysis was 1.002 (1.000–1.005) (P=0.036), and that HR in the multivariate analysis was 1.004 (1.000–1.007) (P=0.027). The effect of age on CSM was not significant. In our study, age was a continuous variable and stratification analysis was not conducted on age.
Previous studies have reported that differences existed in the incidence and prognosis of breast cancer among patients of different ethnicities, which might be due to the differences in patients’ economic conditions, living environment, genomics, and epigenetics (38,39). Our study also found that the risk of CSM was higher in the Black race in comparison with the White. Marital status is also an important factor affecting patients’ prognosis (40). Our study found that the risk of CSM in unmarried and divorced patients was 1.37 times and 1.32 times that of the married patients, respectively. However, in multivariate analysis, no difference was found in the effect of marital status on CSM. Therefore, we interpreted that marriage was not associated with the CSM of TNBC, and randomized controlled trials are required for further validation.
The survival rate differed when the tumors were located in different sites. Studies have demonstrated that the upper outer quadrant of the breast is the most common site where breast cancer occurs, and the prognosis was generally considered the best if breast cancer was located in the upper outer quadrant (41,42). A study reported that the prognosis was best if the tumors originated in the lower lateral part of the breast (43). We also found that the most common primary tumor site in TNBC was the upper outer quadrant, which accounted for 38.12%. The incidence of tumors located in other sites was relatively balanced. Compared with the upper outer quadrant, the risk of CSM was elevated when tumors were located in all other sites.
TNBC patients are not sensitive to endocrine and targeted therapies, and chemotherapy and radiotherapy are key therapeutic strategies (26). Studies have presented that receiving radiotherapy and chemotherapy after surgery has significant clinical value in improving the prognosis of patients (26,44). Recently, neo-adjuvant chemotherapy has gradually become the first choice of systemic therapy for TNBC, especially for those who have locally advanced tumors (45,46). Neoadjuvant chemotherapy remarkably has been shown to improve the prognosis of the patients who received neo-adjuvant chemotherapy before surgery and achieved pathologic complete response during surgery (47). For patients with a locally advanced or advanced tumor, local radiotherapy can be beneficial to long-term survival (48). Our study illustrated that the risk of CSM was significantly increased if patients were not treated with chemotherapy, indicating that chemotherapy could provide clinical benefit for TNBC. However, our results found that radiotherapy did not affect CSM in TNBC patients, which was resulted from the differences in the clinicopathological features between the patients with and without radiotherapy. This bias was not excluded in our comparisons. Hence, the effect of radiotherapy on the risk for CSM of TNBC patients requires further exploration.
Metastasis is a key factor for poor prognosis of breast cancer. As reported, metastases occurred in approximately 5–10% of newly diagnosed cases of breast cancer, and about 500,000 people around the world die from metastatic breast cancer annually (49). Distant metastases are most commonly found in the liver, lungs, bone, and brain. Distant metastases critically influence the life quality and prognosis of patients (50-52). TNBC, among all the breast cancer subtypes, has the highest possibility of distant metastasis. There is an urgent need for effective treatment for metastatic TNBC, which remains the biggest challenge in breast cancer treatment (53). Lymph node metastasis, a pivotal clinical factor for judging the stage of breast cancer and prognosis, is also one of the factors for making clinical treatment decisions (54). In breast cancer, common methods for diagnosing metastasis of lymph node are biopsy of sentinel lymph node, hollow needle biopsy, axillary lymph node dissection, and evaluation with imaging (55). The prognosis of the patients with lymph node metastases is poorer than those without. More than 4 metastasized axillary lymph nodes indicates a higher risk of distant metastases (56,57). Our study discovered that axillary lymph node metastases, brain metastases, and liver metastases were independent risk factors for the CSM of TNBC. Compared with the cases of negative axillary lymph nodes, the HRs of 1–3 metastasized lymph nodes and more than 4 metastasized lymph nodes were 3.036 (2.333–3.949) and 1.701 (1.370–2.111), respectively, which was consistent with the currently recognized results in other research. Patients with brain and liver metastases had a significantly higher risk of CSM. No significant difference was found between bone metastases and lung metastases in multivariate analysis.
This study was conducted by including large multicenter studies and high-quality SEER database to ensure the accuracy of the identified independent risk factors affecting the CSM of TNBC patients. As far as we know, this study is the first to establish a competing risk model for predicting tumor-specific survival in TNBC patients and validate that the accuracy of this model is satisfactory. However, limitations still existed. First, this study was a retrospective study, therefore selection bias was hard to avoid, which might have led to bias in results. Secondly, the clinical diagnosis and treatment of TNBC is a complex and multi-stage process. If more comprehensive data like tumor markers, family history, menstrual history, fertility status, genomic status, and body mass can be included, the prediction accuracy of the model can be improved. Finally, our predictive model has only been validated by using the internal data. Further validation is required by conducting prospective studies or high-quality randomized controlled trials.
Conclusions
This study is the first to use a competing risk model to study the CSM risk in TNBC patients, screen the independent prognostic factors for the CSM of TNBC, and construct a predictive nomogram for CSM risk. The accuracy and reliability of the nomogram are satisfactory. It can be used in clinical evaluation of the prognosis of TNBC patients, and to provide certain theoretical support for clinicians to make decisions.
Acknowledgments
Funding: This work was supported by the Open Project of Embryonic Stem Cell Research Hubei Key Laboratory (No. 2022ESOF016) and the Foundation Project of Taihe Hospital, Hubei University of Medicine (No. 2022JJXM023).
Footnote
Reporting Checklist: The authors have completed the TRIPOD reporting checklist. Available at https://gs.amegroups.com/article/view/10.21037/gs-22-650/rc
Conflicts of Interest: All authors have completed the ICMJE uniform disclosure form (available at https://gs.amegroups.com/article/view/10.21037/gs-22-650/coif). The authors have no conflicts of interest to declare.
Ethical Statement: The authors are accountable for all aspects of the work in ensuring that questions related to the accuracy or integrity of any part of the work are appropriately investigated and resolved. The study was conducted in accordance with the Declaration of Helsinki (as revised in 2013).
Open Access Statement: This is an Open Access article distributed in accordance with the Creative Commons Attribution-NonCommercial-NoDerivs 4.0 International License (CC BY-NC-ND 4.0), which permits the non-commercial replication and distribution of the article with the strict proviso that no changes or edits are made and the original work is properly cited (including links to both the formal publication through the relevant DOI and the license). See: https://creativecommons.org/licenses/by-nc-nd/4.0/.
References
- DeSantis CE, Ma J, Gaudet MM, et al. Breast cancer statistics, 2019. CA Cancer J Clin 2019;69:438-51. [Crossref] [PubMed]
- Li Z, Zhang H, Wang X, et al. Identification of cuproptosis-related subtypes, characterization of tumor microenvironment infiltration, and development of a prognosis model in breast cancer. Front Immunol 2022;13:996836. [Crossref] [PubMed]
- Sung H, Ferlay J, Siegel RL, et al. Global Cancer Statistics 2020: GLOBOCAN Estimates of Incidence and Mortality Worldwide for 36 Cancers in 185 Countries. CA Cancer J Clin 2021;71:209-49. [Crossref] [PubMed]
- Loibl S, Poortmans P, Morrow M, et al. Breast cancer. Lancet 2021;397:1750-69. Erratum in: Lancet 2021;397:1710. [Crossref] [PubMed]
- Hayes DF. Further Progress for Patients with Breast Cancer. N Engl J Med 2019;380:676-7. [Crossref] [PubMed]
- Yin L, Duan JJ, Bian XW, et al. Triple-negative breast cancer molecular subtyping and treatment progress. Breast Cancer Res 2020;22:61. [Crossref] [PubMed]
- Valachis A, Nyström P, Fredriksson I, et al. Treatment patterns, risk for hospitalization and mortality in older patients with triple negative breast cancer. J Geriatr Oncol 2021;12:212-8. [Crossref] [PubMed]
- Crozier JA, Pezzi TA, Hodge C, et al. Addition of chemotherapy to local therapy in women aged 70 years or older with triple-negative breast cancer: a propensity-matched analysis. Lancet Oncol 2020;21:1611-9. [Crossref] [PubMed]
- Garrido-Castro AC, Lin NU, Polyak K. Insights into Molecular Classifications of Triple-Negative Breast Cancer: Improving Patient Selection for Treatment. Cancer Discov 2019;9:176-98. [Crossref] [PubMed]
- Zhao S, Ma D, Xiao Y, et al. Molecular Subtyping of Triple-Negative Breast Cancers by Immunohistochemistry: Molecular Basis and Clinical Relevance. Oncologist 2020;25:e1481-91. [Crossref] [PubMed]
- Voorwerk L, Slagter M, Horlings HM, et al. Immune induction strategies in metastatic triple-negative breast cancer to enhance the sensitivity to PD-1 blockade: the TONIC trial. Nat Med 2019;25:920-8. [Crossref] [PubMed]
- Dirix LY, Takacs I, Jerusalem G, et al. Avelumab, an anti-PD-L1 antibody, in patients with locally advanced or metastatic breast cancer: a phase 1b JAVELIN Solid Tumor study. Breast Cancer Res Treat 2018;167:671-86. [Crossref] [PubMed]
- Schmid P, Rugo HS, Adams S, et al. Atezolizumab plus nab-paclitaxel as first-line treatment for unresectable, locally advanced or metastatic triple-negative breast cancer (IMpassion130): updated efficacy results from a randomised, double-blind, placebo-controlled, phase 3 trial. Lancet Oncol 2020;21:44-59. [Crossref] [PubMed]
- Wolbers M, Koller MT, Stel VS, et al. Competing risks analyses: objectives and approaches. Eur Heart J 2014;35:2936-41. [Crossref] [PubMed]
- Kim HT. Cumulative incidence in competing risks data and competing risks regression analysis. Clin Cancer Res 2007;13:559-65. [Crossref] [PubMed]
- Verduijn M, Grootendorst DC, Dekker FW, et al. The analysis of competing events like cause-specific mortality--beware of the Kaplan-Meier method. Nephrol Dial Transplant 2011;26:56-61. [Crossref] [PubMed]
- Haller B, Schmidt G, Ulm K. Applying competing risks regression models: an overview. Lifetime Data Anal 2013;19:33-58. [Crossref] [PubMed]
- Austin PC, Lee DS, Fine JP. Introduction to the Analysis of Survival Data in the Presence of Competing Risks. Circulation 2016;133:601-9. [Crossref] [PubMed]
- Yang J, Li Y, Liu Q, et al. Brief introduction of medical database and data mining technology in big data era. J Evid Based Med 2020;13:57-69. [Crossref] [PubMed]
- Pan X, Yang W, Chen Y, et al. Nomogram for predicting the overall survival of patients with inflammatory breast cancer: A SEER-based study. Breast 2019;47:56-61. [Crossref] [PubMed]
- Sharma P, Kimler BF, O’Dea A, et al. Randomized Phase II Trial of Anthracycline-free and Anthracycline-containing Neoadjuvant Carboplatin Chemotherapy Regimens in Stage I-III Triple-negative Breast Cancer (NeoSTOP). Clin Cancer Res 2021;27:975-82. [Crossref] [PubMed]
- Teichgraeber DC, Guirguis MS, Whitman GJ. Breast Cancer Staging: Updates in the AJCC Cancer Staging Manual, 8th Edition, and Current Challenges for Radiologists, From the AJR Special Series on Cancer Staging. AJR Am J Roentgenol 2021;217:278-90.
- O’Sullivan CC, Loprinzi CL, Haddad TC. Updates in the Evaluation and Management of Breast Cancer. Mayo Clin Proc 2018;93:794-807. [Crossref] [PubMed]
- Lim YJ, Lee SW, Choi N, et al. A Novel Prognostic Nomogram for Predicting Risks of Distant Failure in Patients with Invasive Breast Cancer Following Postoperative Adjuvant Radiotherapy. Cancer Res Treat 2018;50:1140-8. [Crossref] [PubMed]
- Hou N, Wu J, Xiao J, et al. Development, verification, and comparison of a risk stratification model integrating residual cancer burden to predict individual prognosis in early-stage breast cancer treated with neoadjuvant therapy. ESMO Open 2021;6:100269. [Crossref] [PubMed]
- Min N, Wei Y, Zheng Y, et al. Advancement of prognostic models in breast cancer: a narrative review. Gland Surg 2021;10:2815-31. [Crossref] [PubMed]
- Dai D, Jin H, Wang X. Nomogram for predicting survival in triple-negative breast cancer patients with histology of infiltrating duct carcinoma: a population-based study. Am J Cancer Res 2018;8:1576-85.
- Zhang W, Zhang BL, He JJ, et al. Clinicopathological characteristics and treatment of young women with breast cancer in China: a nationwide multicenter 10-year retrospective study. Gland Surg 2021;10:175-85. [Crossref] [PubMed]
- Paluch-Shimon S, Cardoso F, Partridge AH, et al. ESO-ESMO 4th International Consensus Guidelines for Breast Cancer in Young Women (BCY4). Ann Oncol 2020;31:674-96. [Crossref] [PubMed]
- Breast Cancer Association Consortium. Breast Cancer Risk Genes - Association Analysis in More than 113,000 Women. N Engl J Med 2021;384:428-39. [Crossref] [PubMed]
- Copson ER, Maishman TC, Tapper WJ, et al. Germline BRCA mutation and outcome in young-onset breast cancer (POSH): a prospective cohort study. Lancet Oncol 2018;19:169-80. [Crossref] [PubMed]
- Anders CK, Hsu DS, Broadwater G, et al. Young age at diagnosis correlates with worse prognosis and defines a subset of breast cancers with shared patterns of gene expression. J Clin Oncol 2008;26:3324-30. [Crossref] [PubMed]
- Avci O, Tacar SY, Seber ES, et al. Breast cancer in young and very young women; Is age related to outcome? J Cancer Res Ther 2021;17:1322-7. [Crossref] [PubMed]
- Freedman RA, Keating NL, Lin NU, et al. Breast cancer-specific survival by age: Worse outcomes for the oldest patients. Cancer 2018;124:2184-91. [Crossref] [PubMed]
- Barish R, Lynce F, Unger K, et al. Management of Cardiovascular Disease in Women With Breast Cancer. Circulation 2019;139:1110-20. [Crossref] [PubMed]
- Ramin C, Schaeffer ML, Zheng Z, et al. All-Cause and Cardiovascular Disease Mortality Among Breast Cancer Survivors in CLUE II, a Long-Standing Community-Based Cohort. J Natl Cancer Inst 2021;113:137-45. [Crossref] [PubMed]
- Upshaw JN. Cardio-oncology: protecting the heart from curative breast cancer treatment. Gland Surg 2018;7:350-65. [Crossref] [PubMed]
- Jones GS, Hoadley KA, Olsson LT, et al. Hepatocyte growth factor pathway expression in breast cancer by race and subtype. Breast Cancer Res 2021;23:80. [Crossref] [PubMed]
- Ko NY, Hong S, Winn RA, et al. Association of Insurance Status and Racial Disparities With the Detection of Early-Stage Breast Cancer. JAMA Oncol 2020;6:385-92. [Crossref] [PubMed]
- Shrout MR, Renna ME, Madison AA, et al. Breast cancer survivors’ satisfying marriages predict better psychological and physical health: A longitudinal comparison of satisfied, dissatisfied, and unmarried women. Psychooncology 2021;30:699-707. [Crossref] [PubMed]
- Sohn VY, Arthurs ZM, Sebesta JA, et al. Primary tumor location impacts breast cancer survival. Am J Surg 2008;195:641-4. [Crossref] [PubMed]
- Hwang KT, Kim J, Kim EK, et al. Poor Prognosis of Lower Inner Quadrant in Lymph Node-negative Breast Cancer Patients Who Received No Chemotherapy: A Study Based on Nationwide Korean Breast Cancer Registry Database. Clin Breast Cancer 2017;17:e169-84. [Crossref] [PubMed]
- Siotos C, McColl M, Psoter K, et al. Tumor Site and Breast Cancer Prognosis. Clin Breast Cancer 2018;18:e1045-52. [Crossref] [PubMed]
- Bianchini G, De Angelis C, Licata L, et al. Treatment landscape of triple-negative breast cancer - expanded options, evolving needs. Nat Rev Clin Oncol 2022;19:91-113. [Crossref] [PubMed]
- Hwang SY, Park S, Kwon Y. Recent therapeutic trends and promising targets in triple negative breast cancer. Pharmacol Ther 2019;199:30-57. [Crossref] [PubMed]
- Yao DS, Wang W, Chang JY, et al. Neoadjuvant pyrotinib plus nab-paclitaxel, doxorubicin, and cyclophosphamide for HER2-positive locally advanced breast cancer: a retrospective case-series study. Gland Surg 2021;10:3362-8. [Crossref] [PubMed]
- Kennedy WR, Tricarico C, Gabani P, et al. Predictors of Distant Metastases in Triple-Negative Breast Cancer Without Pathologic Complete Response After Neoadjuvant Chemotherapy. J Natl Compr Canc Netw 2020;18:288-96. [Crossref] [PubMed]
- He MY, Rancoule C, Rehailia-Blanchard A, et al. Radiotherapy in triple-negative breast cancer: Current situation and upcoming strategies. Crit Rev Oncol Hematol 2018;131:96-101. [Crossref] [PubMed]
- Cardoso F, Spence D, Mertz S, et al. Global analysis of advanced/metastatic breast cancer: Decade report (2005-2015). Breast 2018;39:131-8. [Crossref] [PubMed]
- Tahara RK, Brewer TM, Theriault RL, et al. Bone Metastasis of Breast Cancer. Adv Exp Med Biol 2019;1152:105-29. [Crossref] [PubMed]
- Wang Y, Ye F, Liang Y, et al. Breast cancer brain metastasis: insight into molecular mechanisms and therapeutic strategies. Br J Cancer 2021;125:1056-67. [Crossref] [PubMed]
- Hosonaga M, Saya H, Arima Y. Molecular and cellular mechanisms underlying brain metastasis of breast cancer. Cancer Metastasis Rev 2020;39:711-20. [Crossref] [PubMed]
- Al-Mahmood S, Sapiezynski J, Garbuzenko OB, et al. Metastatic and triple-negative breast cancer: challenges and treatment options. Drug Deliv Transl Res 2018;8:1483-507. [Crossref] [PubMed]
- Arroyo-Crespo JJ, Armiñán A, Charbonnier D, et al. Characterization of triple-negative breast cancer preclinical models provides functional evidence of metastatic progression. Int J Cancer 2019;145:2267-81. [Crossref] [PubMed]
- Horváth Z, Paszt A, Simonka Z, et al. Is axillary lymph node dissection necessary for positive preoperative aspiration cytology lymph node results? Eur J Surg Oncol 2020;46:504-10. [Crossref] [PubMed]
- Liu F, Hardiman T, Wu K, et al. Systemic immune reaction in axillary lymph nodes adds to tumor-infiltrating lymphocytes in triple-negative breast cancer prognostication. NPJ Breast Cancer 2021;7:86. [Crossref] [PubMed]
- Li CH, Karantza V, Aktan G, et al. Current treatment landscape for patients with locally recurrent inoperable or metastatic triple-negative breast cancer: a systematic literature review. Breast Cancer Res 2019;21:143. [Crossref] [PubMed]
(English Language Editor: J. Jones)