Development and validation of a tissue-based DNA methylation risk-score model to predict the prognosis of surgically resected pancreatic cancer patients
Introduction
Pancreatic cancer (PC) is a highly lethal malignancy (1) with a 5-year patient survival rate of only 6% (2). Early-stage PC is usually asymptomatic, and most patients are diagnosed at an advanced stage after the tumor invades surrounding tissues or metastasizes to distant organs. Complete surgical resection is a potentially curative therapy for patients with PC. However, only 27% of patients with resected PC survive for 5 years (3,4). A precise prognostic staging classifier would help clinicians to identify surgically resected patients who are at especially high risk for occult metastasis or have a worse-than-expected prognosis among patients who meet clinicopathological criteria for the same-stage disease. Traditional clinical classifiers, including the pathologic American Joint Committee on Cancer (AJCC) tumor-node-metastasis (TNM) staging, serum cancer antigen (CA) 125, serum protein-carbohydrate antigen 19-9 (CA19-9), and carcinoembryonic antigen (CEA), are still used to assess the prognosis risk stratification and aid treatment decision-making (5,6). It is challenging to predict patient outcomes due to the wide variability in results and genetic heterogeneity. In this regard, there is an urgent need to identify accurate data from the clinical examination of patient specimens to allow for risk stratification and prognostic prediction, which could have a potential benefit in reducing mortality from this currently lethal disease.
Aberrant DNA methylation has been noted in many studies as a common epigenetic change in cancer, causing gene regulation changes that promote oncogenesis (7). When it occurs in gene promoters, it can modify DNA accessibility to transcription factors and help recruit silencing-associated proteins, thus resulting in gene silencing (8). Cancer cells harbor global hypomethylation and regional hypermethylation, especially in CpG-rich contexts. Utilizing DNA methylation as a biomarker offers several advantages compared with other epigenetic alterations, including but not limited to, higher sensitivity and dynamic range, multiple altered sites within each targeted region, and a tremendous number of targeted regions in a disease. In PC, DNA methylation has been studied as a mechanism associated with the process of tumorigenesis, which also affects the regulation of genome stability and gene transcription (9). Genome-wide studies of CpG islands have revealed that thousands of methylated genes can potentially differentiate PC tumor tissues from normal tissues (10). Several studies have attempted to derive blood-based DNA methylation biomarkers for the early detection and diagnosis of PC (11,12). Recently, several studies have explored the genome-wide detection of DNA methylation profiling to screen potential prognosis-related tumor biomarkers. However, most of studies were limited by unsatisfied accuracy or developed based on small cohort.
In this study, we analyzed the methylation profiles of tumor and matched adjacent long-distance tissues from 50 patients with PC using methylated DNA sequencing to identify potential prognosis-specific DNA methylation markers. We also developed and validated a practical and reliable tissue-based DNA methylation risk score to improve risk stratification for post-operative patients with PC. We further demonstrated that our risk score could independently predict patients with high mortality risk and increase precision in clinical decision-making. We present the following article in accordance with the TRIPOD reporting checklist (available at https://gs.amegroups.com/article/view/10.21037/gs-22-517/rc).
Methods
Study design and participants
Surgical tissue samples from 50 patients with stage I to IIB PC diagnosed at The First Affiliated Hospital of Soochow University were used to derive differentially expressed genes associated with prognosis. All the patients had R0 (≥1 mm margin) resections. The following data were collected: clinical and pathologic characteristics (gender, age, smoking history, tumor grade and stage), follow-up data and biological data (CEA, CA199, CA125, CA153, AFP, and serum ferritin).
In the validation cohort, DNA methylation and corresponding clinical data were retrieved from 166 patients with stage I to IIB PC in the publicly available The Cancer Genome Atlas-Pancreatic ductal adenocarcinoma (TCGA-PDAC) dataset. Ninety-eight patients (59.03%) had R0 resections.
All samples and clinical data were collected after approval by the First Affiliated Hospital of Soochow University Institutional Review Board (No. 2019054). Written informed consent was obtained from every participant to use their samples. All collection and usage of human tissue samples and clinical data were carried out in accordance with the Declaration of Helsinki (as revised in 2013).
Tissue sample collection
All tumor tissue samples were collected during surgery after the frozen intraoperative section proved malignant. To analyze the methylation characteristics of the PC patients’ normal adjacent pancreatic tissues, we collected adjacent long-distance pancreatic tissues that were at least 3 cm away from the edge of the tumor. All the pancreatic tumor and adjacent samples were stored at −80 ℃.
DNA extraction from tissues samples
DNA from tumor and adjacent tissue samples was extracted using the QIAamp DNA FFPE Tissue Kit (Qiagen, Valencia, CA, USA). The presence of tumor cells in the tumor samples and the absence of tumor cells in the adjacent samples were confirmed by histopathological assessment before DNA extraction. DNA was quantified with the Qubit 2.0 fluorimeter (ThermoFisher Scientific, Waltham, MA, USA). The minimum amount of input DNA was more than 50 ng. Extracted tissue DNA was stored in an IDTE buffer at −20 ℃.
Bisulfite targeted sequencing and methylation data processing
The whole-genome bisulfite sequencing library was generated by the brELSA method (Burning Rock Biotech, Guangzhou, China) (13,14). Briefly, purified cell-free DNA (cfDNA) was incubated with sodium bisulfite. The converted fragments were ligated to the appropriate adapters, then amplified and purified to generate the whole-genome bisulfite sequencing library. Bespoke pancreatic-cancer methylation profiling RNA baits were performed that covered 80,672 CpG sites and spanned 1.05 Mb of the human genome. The target libraries were subsequently quantified by RT-PCR (Kapa Biosciences, Wilmington, MA, USA) and sequenced on a NovaSeq 6000 (Illumina, San Diego, CA, USA) with an average sequencing depth of 500× on the tissue sample.
Bisulfite sequencing data analysis was performed using an optimized pipeline. Raw sequencing data were trimmed by Trimmomatic (v.0.32) and aligned by BWA-meth (v.0.2.2). PCR duplicates from aligned sequences were marked with Samblaster (v.0.1.20). The low mapping quality (MAPQ <20) or improper pairing reads were then removed by Sambamba (v.0.4.7). The overlapping reads from paired reads were removed using in-house scripts.
Analysis of methylation patterns
Methylation levels were defined as scores to reflect the methylation features of each sample (13,14). The genomic region between the neighboring CpG sites with the r2 value was defined as the methylation block. A total of 80,672 CpG sites were grouped into 8,312 methylation blocks by linkage disequilibrium. MethylMean was defined as the average methylation level in a particular genomic region within a methylation block and is presented as the following equation:
Where 𝑙: represents the number of sequencing reads for the multiple CpG sites that cover the methylation block; M1: represents the number of sequencing reads for methylated CpG sites per methylation block covered in the forward strand; M2: represents the number of sequencing reads for methylated CpG sites per methylation block covered in the reverse strand; U1: represents the number of sequencing reads for methylated CpG sites per methylation block uncovered in the forward strand; U2: represents the number of sequencing reads for methylated CpG sites per methylation block uncovered in the reverse strand.
Statistical analysis
Initially, a univariate Cox regression analysis was used to identify the overall survival (OS)-related differential methylation blocks (DMBs) with the “survival” R package. The hazard ratio (HR) and P value were provided. The DMBs with P<0.001 were identified. A total of 18 survival-related DMBs were obtained. Seven DMBs were identified by a Least Absolute Shrinkage and Selection Operator (LASSO) regression analysis using the “glmnet” R package. Stepwise regression analysis was applied to optimize the model. Finally, four DMBs were identified for potential clinical application as the prognostic signature. By fitting a multivariable Cox proportional hazards model on these four DMBs, we determined the coefficients of each DMB and obtained a combined prognostic score (designated as the risk score) for each individual. The sensitivity and specificity of the risk score in predicting patient survival was analyzed using a time-dependent ROC curve.
All the data were analyzed using the R package (R version 4.0.2; R: The R-Project for Statistical Computing, Vienna, Austria). The DMBs were initially screened with the “limma” R package. Methylation blocks with an Δβ>0.15 and a Benjamini-Hochberg adjusted P<0.05 were identified as DMBs. Methylation blocks with an Δβ>0.15 and Benjamini-Hochberg adjusted P<0.05 were defined as hypermethylation blocks, whereas methylation blocks with Δβ<−0.15 and Benjamini-Hochberg adjusted P<0.05 were defined as hypomethylation blocks. A Kyoto Encyclopedia of Genes and Genomes (KEGG) functional enrichment analysis was performed using the “clusterProfiler” R package. Gene set enrichment analysis (GSEA) was performed to elucidate the molecular mechanisms of the predictive groups (15). Immune and estimate scores were calculated using the Estimation of STromal and Immune cells in MAlignant Tumor tissues using Expression data (ESTIMATE) algorithm (16). The abundance of B, CD4+ T cells, CD8+ T cells, dendritic cells, neutrophils, and macrophages were estimated using the tumor immune estimation resource (TIMER) algorithm (17).
Student’s t-test was performed to determine the differences in groups. All statistical tests were two-sided with a P value less than 0.05 considered statistically significant. All statistical analyses were carried out using the software R version 4.0.1 (http://www.r-project.org).
Results
Baseline patient characteristics
Table 1 presents the baseline information for the 50 patients comprising the training cohort, all of whom underwent surgery for PC at The First Affiliated Hospital of Soochow University (SU cohort). The majority of patients had mediastinal lymph node involvement (62%). The primary site for 64% of patients was the pancreatic head, and 58% had elevated CA199 levels (≥37 U/mL).
Table 1
Clinicopathological features | Subtypes | Overall (n=50), n (%) |
---|---|---|
Sex | Female | 20 (40.0) |
Male | 30 (60.0) | |
Age, years | <60 | 17 (34.0) |
≥60 | 33 (66.0) | |
Smoking | N | 43 (86.0) |
Y | 7 (14.0) | |
Drinking | N | 46 (92.0) |
Y | 4 (8.0) | |
CEA, ng/mL | <5 | 22 (44.0) |
≥5 | 17 (34.0) | |
NA | 11 (22.0) | |
CA199, U/mL | <37 | 17 (34.0) |
≥37 | 29 (58.0) | |
NA | 4 (8.0) | |
CA125, U/mL | <35 | 32 (64.0) |
≥35 | 13 (26.0) | |
NA | 5 (10.0) | |
CA153, U/mL | <25 | 41 (82.0) |
≥25 | 3 (6.0) | |
NA | 6 (12.0) | |
AFP, μg/L | <25 | 43 (86.0) |
≥25 | 2 (4.0) | |
NA | 5 (10.0) | |
SF, ng/mL | <200 | 10 (20.0) |
≥200 | 11 (22.0) | |
NA | 29 (58.0) | |
Site | Body | 18 (36.0) |
Head | 32 (64.0) | |
Stage | IA | 1 (2.0) |
IIA | 29 (58.0) | |
IIB | 20 (40.0) | |
T | T1 | 1 (2.0) |
T2 | 16 (32.0) | |
T3 | 33 (66.0) | |
N | N0 | 31 (62.0) |
N1 | 19 (38.0) |
SU, Soochow University; CEA, carcinoembryonic antigen; CA, carbohydrate antigen; AFP, alpha fetoprotein; SF, serum ferritin.
A total of 166 stage I–II PC patients from the TCGA-PDAC cohort made up the validation cohort. Because of the limited information available for the TCGA-PDAC cohort, tumor markers and pathological staging information in these patients were excluded from further analysis. As shown in Table 2, there was no significant distribution bias between the training and validation cohorts concerning sex and age (P>0.05). The analysis process used to identify the prognostic DNA methylation signature is shown in Figure 1.
Table 2
Features | Subtypes | Overall (n=216), n (%) | SU (n=50), n (%) | TCGA (n=166), n (%) | P |
---|---|---|---|---|---|
Sex | Female | 93 (43.1) | 20 (40.0) | 73 (44.0) | 0.738 |
Male | 123 (56.9) | 30 (60.0) | 93 (56.0) | ||
Age, years | <60 | 70 (32.4) | 17 (34.0) | 53 (31.9) | 0.919 |
≥60 | 146 (67.6) | 33 (66.0) | 113 (68.1) | ||
Stage | I | 1 (0.5) | 0 (0.0) | 1 (0.6) | <0.001 |
IA | 6 (2.8) | 1 (2.0) | 5 (3.0) | ||
IB | 13 (6.0) | 0 (0.0) | 13 (7.8) | ||
IIA | 59 (27.3) | 29 (58.0) | 30 (18.1) | ||
IIB | 137 (63.4) | 20 (40.0) | 117 (70.5) |
PC, pancreatic cancer; SU, Soochow University; TCGA-PDAC, The Cancer Genome Atlas-pancreatic ductal adenocarcinoma.
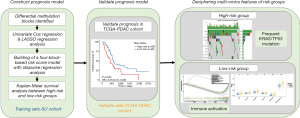
Construction and validation of the prognostic DNA methylation risk score
We found that 1,162 DMBs were markedly changed between the 50 pairs of tumor and adjacent tissues (Figure 2A). We considered these potential prognostic DMBs, of which 737 were hypermethylation blocks, and 425 were hypomethylation blocks (Figure 2B). To further investigate the biological functions of these 1,162 DMBs, a KEGG pathway enrichment analysis was performed. Genes involved in the hypermethylated blocks were significantly enriched in neuroactive ligand-receptor interaction and Ca+ signaling pathways, and those involved in the hypomethylated blocks were enriched in E. coli infection and leukocyte transendothelial migration pathways (Figure 2C,2D). All these pathways were PC related (18-21). Molecular interaction network diagrams of the selected top KEGG-related hypermethylated and hypomethylated blocks are presented in Figure S1.
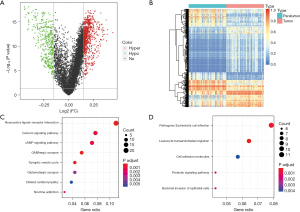
To identify the DMBs associated with OS, we performed univariate Cox regression and LASSO Cox regression analyses in sequence. We conducted the univariate Cox regression analysis to determine the DMBs related to survival, then calculated the HR for each DMB in both cohorts (Tables S1,S2). By selecting the DMBs with a P value <0.001, we identified 18 DMBs that were significantly correlated with PC patient survival. Then, LASSO Cox regression reduced the number of DMBs to four for potential clinical application as a prognostic signature. Finally, the four survival-associated DMBs were identified by stepwise regression analysis (Figure 3). The calculated coefficients for each DMB based on multivariate Cox regression are listed in Table S3. The genes related to the four DMBs and associated with survival were HOXA10, WASF2, CSTF3-DT, and LINC01624 (Table S4).
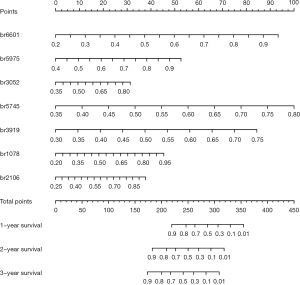
To assist in using the identified survival-associated DMBs in clinical practice, we developed a model to predict the OS of patients based on these four prognostic-related DMBs. A risk score was derived for each patient based on this model. The Youden index was used to set the cutoff value as 14.04. The 50 patients were stratified into a low-risk group (risk score ≥14.04) and a high-risk group (risk score <14.04). The survival status of patients in the low- and high-risk groups was observed in both the SU and TCGA-PDAC cohorts.
The relationship between risk score and prognosis in the SU cohort was established using a Kaplan-Meier (KM) survival analysis. The prognosis of patients in the high-risk group was significantly worse than in the low-risk group (P<0.001; Figure 4A-4D). As all the follow-up data were obtained within 2 years, receiver operating characteristic (ROC) curves were used to assess the prognostic performance of the risk score using OS data at 1 year and 2 years after diagnosis. The ROC area under the curve (AUC) value in the SU cohort at 1 and 2 years was 0.789 and 0.852, respectively (Figure 4E). There was no significant difference in clinical and pathological factors between the high- and low-risk groups (Table 3). In the independent TCGA-PDAC cohort survival analysis, patients in the high-risk group (cutoff value 14.04 derived from the SU cohort) also showed significantly poorer OS than those in the low-risk group (P=0.004; Figure 5A-5D). The ROC AUC value in the validation cohort at 1- and 2-year was 0.677 and 0.611, respectively (Figure 5E). In the TCGA-PDAC R0 resection patients, the KM survival analysis showed that the OS of PC patients in the high-risk group was significantly worse than that in the low-risk group (P=0.016; Figure S2). As most of the follow-up data covered only 2 years, we constructed a calibration curve of the predicted 1-year OS in PC patients using the DNA methylation model (Figure S3).
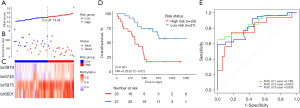
Table 3
Variables | High risk (n=19), n (%) | Low risk (n=31), n (%) | P |
---|---|---|---|
Sex | 9 (47.4) | 11 (35.5) | 0.592 |
10 (52.6) | 20 (64.5) | ||
Age, years | 6 (31.6) | 11 (35.5) | 1.000 |
13 (68.4) | 20 (64.5) | ||
Smoking | 16 (84.2) | 27 (87.1) | 1.000 |
3 (15.8) | 4 (12.9) | ||
Drinking | 18 (94.7) | 28 (90.3) | 0.983 |
1 (5.3) | 3 (9.7) | ||
CEA, ng/mL | 8 (42.1) | 14 (45.2) | 0.561 |
8 (42.1) | 9 (29.0) | ||
3 (15.8) | 8 (25.8) | ||
CA199, U/mL | 4 (21.1) | 13 (41.9) | 0.213 |
14 (73.7) | 15 (48.4) | ||
1 (5.3) | 3 (9.7) | ||
CA125, U/mL | 9 (47.4) | 23 (74.2) | 0.111 |
8 (42.1) | 5 (16.1) | ||
2 (10.5) | 3 (9.7) | ||
CA153, U/mL | 15 (78.9) | 26 (83.9) | 0.566 |
2 (10.5) | 1 (3.2) | ||
2 (10.5) | 4 (12.9) | ||
AFP, μg/L | 17 (89.5) | 26 (83.9) | 0.528 |
0 (0.0) | 2 (6.5) | ||
2 (10.5) | 3 (9.7) | ||
SF, ng/mL | 3 (15.8) | 7 (22.6) | 0.182 |
2 (10.5) | 9 (29.0) | ||
14 (73.7) | 15 (48.4) | ||
Site | 9 (47.4) | 9 (29.0) | 0.314 |
10 (52.6) | 22 (71.0) | ||
Stage | 0 (0.0) | 1 (3.2) | 0.554 |
10 (52.6) | 19 (61.3) | ||
9 (47.4) | 11 (35.5) | ||
T | 0 (0.0) | 1 (3.2) | 0.646 |
7 (36.8) | 9 (29.0) | ||
12 (63.2) | 21 (67.7) | ||
N | 10 (52.6) | 21 (67.7) | 0.442 |
9 (47.4) | 10 (32.3) |
SU, Soochow University; CEA, carcinoembryonic antigen; CA, carbohydrate antigen; AFP, alpha fetoprotein; SF, serum ferritin.
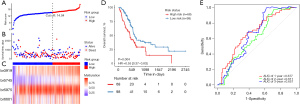
A four-block-based risk score was an independent prognostic indicator for patient survival
To show the predictive ability of the risk score, we assessed well-known potential prognostic clinicopathological parameters in PC patients, including age, gender, smoking, metastasis sites, CEA, CA199, CA153, and CA125 status. There was no significant difference in clinical and pathological factors between the high- and low-risk groups (Table 3). However, the KM survival analysis showed that patients with elevated CA125 (≥35 U/mL) had a shorter OS than patients with normal CA 125 (<35 U/mL, P=0.028; Figure S4). In addition, patients in the high-risk group with elevated CA 125 had a shorter OS than those in the low-risk group with normal CA 125 (P<0.003; Figure 6A). We then performed a multivariable prognostic analysis to evaluate the independent contribution of the risk score using a model that contained important clinicopathologic parameters, including age, sex, CA 125, T, and N stage. Figure 6B shows that the four-block-based risk score remained an independent prognostic indicator when analyzed by Cox regression [P<0.001, HR 2.760 (1.733–4.40)].
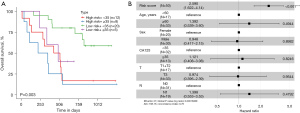
A low-risk score was linked to a high level of immune infiltration
We performed further bioinformatic analyses to explore the genomic alterations, altered pathways, and potentially applicable drugs correlated with the two different risk groups. We found that 260 upregulated and 1,203 downregulated differentially expressed genes (DEGs) were markedly changed between the high- and low-risk groups (|log2FC| >1, adj.P value <0.05; Figure 7A). We then performed a KEGG pathway enrichment analysis to further investigate the biological functions of these 1,463 DEGs. Genes involved in the low-risk group were significantly enriched in immune-related pathways and biological processes such as “cytokine-cytokine receptor interaction” and “chemokine signaling pathway” (Figure 7B,7C). We subsequently used the gene module of the TIMER database to explore the infiltration levels in the high- and low-risk groups. As shown in Figure 7D, patients in the low-risk group had a significantly higher proportion of B cells, CD4+ T cells, CD8+ T cells, neutrophils, macrophages, and myeloid dendritic cells. The cytolytic activity (CYT) and immune scores were also significantly higher in the low-risk group (P<0.001, Figure 7E,7F). Taken together, our results indicated that the risk score was significantly related to immune infiltration and may help to predict the immune microenvironment.
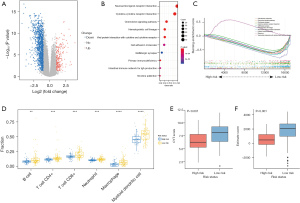
Molecular characteristics and chemotherapeutic sensitivities of patients in the low- and high-risk groups
Genetic mutations in the low- and high-risk groups were analyzed, and the top ten ranked genes with the highest mutation rates were identified. The mutation rates of KRAS, TP53, CDKN2A, and SMAD4 were greater than or equal to 15% in both the low- and high-risk groups (Figure 8A,8B). We also found that the low-risk group had a higher prevalence of the KRAS missense mutation and TP53 mutation than the high-risk group (P≤0.05, Figure 8C).
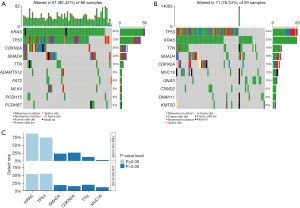
For patients with advanced PC, systemic therapy could potentially reduce the tumor burden and prolong their life. This study further analyzed the drug response of patients in the two groups to three chemotherapy agents, including cisplatin, gemcitabine, and paclitaxel. These chemotherapy drugs showed significant IC50 value differences between the low- and high-risk groups, with the low-risk patients showing increased sensitivity to cisplatin (P=0.0029, Figure 9A) and the high-risk patients showing increased sensitivity to gemcitabine (P=0.017, Figure 9B), and paclitaxel (P=0.003, Figure 9C).
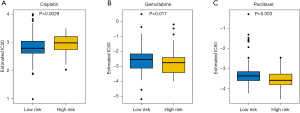
Discussion
Currently, there is no commercially prognostic assay available for patients with PC. Predicting the risk level for patients after surgical resection based on individual tumor biology would clearly benefit informed therapeutic decision-making. Currently, the only accepted prognostic tools for guiding clinical treatment decisions are TNM staging and CA199 expression. However, the prognostic performance of TNM staging for most stages IB–IIB resected PC patients is very limited, with the survival curves being virtually identical (6). The commonly used serum biomarker for predicting prognosis is CA199. Although this antigen is overexpressed in 80% of PC patients, the specificity for PC is poor, and therefore its use as a diagnostic biomarker is not recommended (22). Therefore, we designed a tissue-based, risk-score model based on bisulfate sequencing approaches using data from 50 paired PC tumor and adjacent tissue specimens. We demonstrated that this four-block-based risk score is independent of patients’ clinical features and is able to stratify patients into high- and low-risk groups. More importantly, we confirmed that the risk score could be regarded as an independent predictor of prognostic survival after considering various variables, including age, sex, CA199 expression, and smoking status. We also confirmed that the risk score could stratify patients into high- and low-risk groups in the external cohort from the TCGA-PDAC database and was more effective in forecasting survival time for PC patients in the early stages (stages I–II).
Recently, several studies have explored the genome-wide detection of DNA methylation profiling to screen potential prognosis-related tumor biomarkers. For example, a biomarker panel comprised of a six-gene prognostic signature correlated with survival based on the different methylation patterns of metastatic versus non-metastatic PC (23). This model was trained in a cohort of 34 patients and validated in a cohort of 67 patients. However, their study was derived from the tumor stage at presentation and not from patient survival. Another study developed a 13-gene expression model that predicted the survival of patients with PC and could prove useful for predicting response to chemotherapy (24). Their model was derived from only 15 human PC tumors with stage III or IV disease and validated in a public database of 101 patients with AJCC stage IIb or less. Li et al. (25) developed a set of eight differentially methylated cfDNA markers that could distinguish patients with PC from healthy individuals, but their model was dependent on multiple clinical features. We found none of these signatures have overlapped genes with our risk score. Different studies often yield different combinations of genes, probably due to study design, characteristics of the study cohort, and analysis approaches. In our study, applying our risk score and the cutoff risk score derived from the test cohort to a publicly available gene expression dataset as the external validation cohort was challenging. Each cohort may have a different distribution of patient characteristics, and the experiments may not be implemented by the same procedure with the same platform. Nevertheless, the fact that our risk score was externally validated on a public database adds to the unbiased nature of our study.
Our pathway analysis revealed that many immune-related pathways were enriched in the low-risk group but not in the high-risk group. In addition, differences in tumor-infiltrating immune cells also revealed the distinct tumor immune microenvironment in these two groups. Patients defined as high-risk showed poor OS. We found that 85% of high-risk patients carried KRAS mutations, compared with only 53% of low-risk patients. A previous study identified KRAS mutations in more than 80% of PC patients, and these patients tended to have poor OS independent of tumor stage (26).
Chemotherapy is an essential treatment for advanced disease. Our results revealed that the low-risk group was sensitive to cisplatin, while the high-risk group was sensitive to gemcitabine and paclitaxel. Taken together, our results suggest that our risk-score model is not only able to predict prognosis but also can identify patients with different immune and molecular features and chemotherapy drug sensitivities.
With respect to the four genes involved in this study, only HOXA10 has been intensively studied in various cancer types (27-31), including PC. HOXA10 is a subset of the homeobox gene family, which is well conserved during evolution at the genomic level (27). In this study, we found that HOXA10 expression was correlated with the patient’s OS, which is consistent with previously reported tumor suppressive roles for HOXA10 in prostate cancer (28). Another study showed that HOXA10 promoted cell invasion and the MMP-3 expression of PC cells via the TGFβ2-p38 MAPK pathway and facilitated cell migration and invasion (32). Transcriptomic analysis comparing tumor and adjacent pancreatic tissues also confirmed the overexpression of HOXA10 in tumors (31). Interestingly, genes such as WASF, CSTF3-DT, and LINC01624 have not been reported in PC or any other forms of cancer, to the best of our knowledge. It might indicate that their gene expression is not only regulated by methylation but also controlled by a complex regulatory system. Future studies are strongly recommended.
Despite the significance of our model, several limitations might affect the interpretation of our results. Firstly, the biological functions and molecular mechanisms of some genes involved in the risk score remain unclear. Further studies are required to support the use of our risk score and investigate these various pathways. Secondly, as some important clinicopathological parameters associated with prognosis were unavailable in the external TCGA-PDAC database, we could not conduct a comprehensive analysis of OS in this study. Finally, the sequencing coverage and depth of the SU and TCGA-PDAC cohorts using different assays were varied. Technical variables between the cohorts might introduce bias to the performance of our model. The cross-platform comparison might potentially explain why the AUC of our model in the TCGA-PDAC cohort was lower than that in the testing cohort. Therefore, multi-center, large-scale, prospective studies are required to validate our risk score in clinical practice.
Conclusions
In summary, we developed and validated a four-block risk score for the prognosis of PC patients by analyzing DNA methylation profiles. Our study confirmed that the DNA methylation-based risk score could effectively stratify PC patients into low- and high-risk groups and is also an independent prognostic predictor of OS.
Acknowledgments
We thank all the participants and their families. We also thank Dr. Xi Li, Dr. Jian Wang, and Dr. Xiaozhe Li (Burning Rock Biotech, Guangzhou, China) for their assistance in manuscript writing and data analysis.
Funding: This study was supported by the National Natural Science Foundation of China project (grant number: 81902385), the Foundation Research Project of the Natural Science Foundation of Jiangsu Province (grant number: BK 20201173), the Medical Research of Jiangsu Province project (grant number: Y 2018094) and the Suzhou People’s Livelihood Science and Technology project (grant number: SYS2018037).
Footnote
Reporting Checklist: The authors have completed the TRIPOD reporting checklist. Available at https://gs.amegroups.com/article/view/10.21037/gs-22-517/rc
Data Sharing Statement: Available at https://gs.amegroups.com/article/view/10.21037/gs-22-517/dss
Conflicts of Interest: All authors have completed the ICMJE uniform disclosure form (available at https://gs.amegroups.com/article/view/10.21037/gs-22-517/coif). The authors have no conflicts of interest to declare.
Ethical Statement: The authors are accountable for all aspects of the work in ensuring that questions related to the accuracy or integrity of any part of the work are appropriately investigated and resolved. All samples and clinical data were collected after approval by the First Affiliated Hospital of Soochow University Institutional Review Board (No. 2019054). Written informed consent was obtained from every participant to use their samples. All collection and usage of human tissue samples and clinical data were carried out in accordance with the Declaration of Helsinki (as revised in 2013).
Open Access Statement: This is an Open Access article distributed in accordance with the Creative Commons Attribution-NonCommercial-NoDerivs 4.0 International License (CC BY-NC-ND 4.0), which permits the non-commercial replication and distribution of the article with the strict proviso that no changes or edits are made and the original work is properly cited (including links to both the formal publication through the relevant DOI and the license). See: https://creativecommons.org/licenses/by-nc-nd/4.0/.
References
- Siegel RL, Miller KD, Jemal A. Cancer statistics, 2019. CA Cancer J Clin 2019;69:7-34. [Crossref] [PubMed]
- Vincent A, Herman J, Schulick R, et al. Pancreatic cancer. Lancet 2011;378:607-20. [Crossref] [PubMed]
- Ferrone CR, Brennan MF, Gonen M, et al. Pancreatic adenocarcinoma: the actual 5-year survivors. J Gastrointest Surg 2008;12:701-6. [Crossref] [PubMed]
- Conlon KC, Klimstra DS, Brennan MF. Long-term survival after curative resection for pancreatic ductal adenocarcinoma. Clinicopathologic analysis of 5-year survivors. Ann Surg 1996;223:273-9. [Crossref] [PubMed]
- Kim YC, Kim HJ, Park JH, et al. Can preoperative CA19-9 and CEA levels predict the resectability of patients with pancreatic adenocarcinoma? J Gastroenterol Hepatol 2009;24:1869-75. [Crossref] [PubMed]
- Helm J, Centeno BA, Coppola D, et al. Histologic characteristics enhance predictive value of American Joint Committee on Cancer staging in resectable pancreas cancer. Cancer 2009;115:4080-9. [Crossref] [PubMed]
- Koch A, Joosten SC, Feng Z, et al. Analysis of DNA methylation in cancer: location revisited. Nat Rev Clin Oncol 2018;15:459-66. [Crossref] [PubMed]
- Jones PA, Baylin SB. The epigenomics of cancer. Cell 2007;128:683-92. [Crossref] [PubMed]
- Brancaccio M, Natale F, Falco G, et al. Cell-Free DNA Methylation: The New Frontiers of Pancreatic Cancer Biomarkers' Discovery. Genes (Basel) 2019;11:14. [Crossref] [PubMed]
- Natale F, Vivo M, Falco G, et al. Deciphering DNA methylation signatures of pancreatic cancer and pancreatitis. Clin Epigenetics 2019;11:132. [Crossref] [PubMed]
- Russo M, Misale S, Wei G, et al. Acquired Resistance to the TRK Inhibitor Entrectinib in Colorectal Cancer. Cancer Discov 2016;6:36-44. [Crossref] [PubMed]
- Kisiel JB, Raimondo M, Taylor WR, et al. New DNA Methylation Markers for Pancreatic Cancer: Discovery, Tissue Validation, and Pilot Testing in Pancreatic Juice. Clin Cancer Res 2015;21:4473-81. [Crossref] [PubMed]
- Liu Y, Feng Y, Hou T, et al. Investigation on the potential of circulating tumor DNA methylation patterns as prognostic biomarkers for lung squamous cell carcinoma. Transl Lung Cancer Res 2020;9:2356-66. [Crossref] [PubMed]
- Yang Y, Zheng D, Wu C, et al. Detecting Ultralow Frequency Mutation in Circulating Cell-Free DNA of Early-Stage Non-small Cell Lung Cancer Patients with Unique Molecular Identifiers. Small Methods 2019;3:1900206. [Crossref]
- Subramanian A, Tamayo P, Mootha VK, et al. Gene set enrichment analysis: a knowledge-based approach for interpreting genome-wide expression profiles. Proc Natl Acad Sci U S A 2005;102:15545-50. [Crossref] [PubMed]
- Yoshihara K, Shahmoradgoli M, Martínez E, et al. Inferring tumour purity and stromal and immune cell admixture from expression data. Nat Commun 2013;4:2612. [Crossref] [PubMed]
- Li T, Fan J, Wang B, et al. TIMER: A Web Server for Comprehensive Analysis of Tumor-Infiltrating Immune Cells. Cancer Res 2017;77:e108-10. [Crossref] [PubMed]
- Huang C, Li N, Li Z, et al. Tumour-derived Interleukin 35 promotes pancreatic ductal adenocarcinoma cell extravasation and metastasis by inducing ICAM1 expression. Nat Commun 2017;8:14035. [Crossref] [PubMed]
- Wei P, Tang H, Li D. Insights into pancreatic cancer etiology from pathway analysis of genome-wide association study data. PLoS One 2012;7:e46887. [Crossref] [PubMed]
- Gregório C, Soares-Lima SC, Alemar B, et al. Calcium Signaling Alterations Caused by Epigenetic Mechanisms in Pancreatic Cancer: From Early Markers to Prognostic Impact. Cancers (Basel) 2020;12:1735. [Crossref] [PubMed]
- Luo W, Cao Z, Qiu J, et al. Novel Discoveries Targeting Pathogenic Gut Microbes and New Therapies in Pancreatic Cancer: Does Pathogenic E. coli Infection Cause Pancreatic Cancer Progression Modulated by TUBB/Rho/ROCK Signaling Pathway? A Bioinformatic Analysis. Biomed Res Int 2020;2020:2340124. [Crossref] [PubMed]
- Luo G, Jin K, Deng S, et al. Roles of CA19-9 in pancreatic cancer: Biomarker, predictor and promoter. Biochim Biophys Acta Rev Cancer 2021;1875:188409. [Crossref] [PubMed]
- Stratford JK, Bentrem DJ, Anderson JM, et al. A six-gene signature predicts survival of patients with localized pancreatic ductal adenocarcinoma. PLoS Med 2010;7:e1000307. [Crossref] [PubMed]
- Thompson MJ, Rubbi L, Dawson DW, et al. Pancreatic cancer patient survival correlates with DNA methylation of pancreas development genes. PLoS One 2015;10:e0128814. [Crossref] [PubMed]
- Li S, Wang L, Zhao Q, et al. Genome-Wide Analysis of Cell-Free DNA Methylation Profiling for the Early Diagnosis of Pancreatic Cancer. Front Genet 2020;11:596078. [Crossref] [PubMed]
- Buscail L, Bournet B, Cordelier P. Role of oncogenic KRAS in the diagnosis, prognosis and treatment of pancreatic cancer. Nat Rev Gastroenterol Hepatol 2020;17:153-68. [Crossref] [PubMed]
- Cheng W, Jiang Y, Liu C, et al. Identification of aberrant promoter hypomethylation of HOXA10 in ovarian cancer. J Cancer Res Clin Oncol 2010;136:1221-7. [Crossref] [PubMed]
- Hatanaka Y, de Velasco MA, Oki T, et al. HOXA10 expression profiling in prostate cancer. Prostate 2019;79:554-63. [Crossref] [PubMed]
- Guo C, Ju QQ, Zhang CX, et al. Overexpression of HOXA10 is associated with unfavorable prognosis of acute myeloid leukemia. BMC Cancer 2020;20:586. [Crossref] [PubMed]
- Chen W, Wu G, Zhu Y, et al. HOXA10 deteriorates gastric cancer through activating JAK1/STAT3 signaling pathway. Cancer Manag Res 2019;11:6625-35. [Crossref] [PubMed]
- Mao Y, Shen J, Lu Y, et al. RNA sequencing analyses reveal novel differentially expressed genes and pathways in pancreatic cancer. Oncotarget 2017;8:42537-47. [Crossref] [PubMed]
- Cui XP, Qin CK, Zhang ZH, et al. HOXA10 promotes cell invasion and MMP-3 expression via TGFβ2-mediated activation of the p38 MAPK pathway in pancreatic cancer cells. Dig Dis Sci 2014;59:1442-51. [Crossref] [PubMed]
(English Language Editor: D. Fitzgerald)